Remembering to learn
Understanding the dynamics of networks of memristors, a new paradigm for low-power computation inspired by the structure of the brain.
Memristors are resistors that preserve memory. They can be used to regulate and limit the amount of current that flows through a circuit, as they are able to change the value of their resistance based on their memory of the current and the voltage in the network.
This project investigates how an analytical description of simple memristive circuits can be used to understand the properties of more complicated networks of memristors. Our overall approach uses idealised models where the dynamics can be solved exactly to uncover emergent behaviour. For example, we explore the relationship between the topology of the circuit and the dynamics of memristors using techniques from graph theory. Using mean field theory, we identify the asymptotic behaviour of memristor dynamics beyond the linear regime. We verify our analytic results with numerical solutions, demonstrating the ability of simple models to explain the collective behaviour of complex networks.
The idea that memristors have internal memory means that it is possible for a network of them to learn. This ability can be harnessed to implement models of neural systems, and hence study and mimic the brain. It also makes memristors a potential alternative paradigm for solid-state memory.
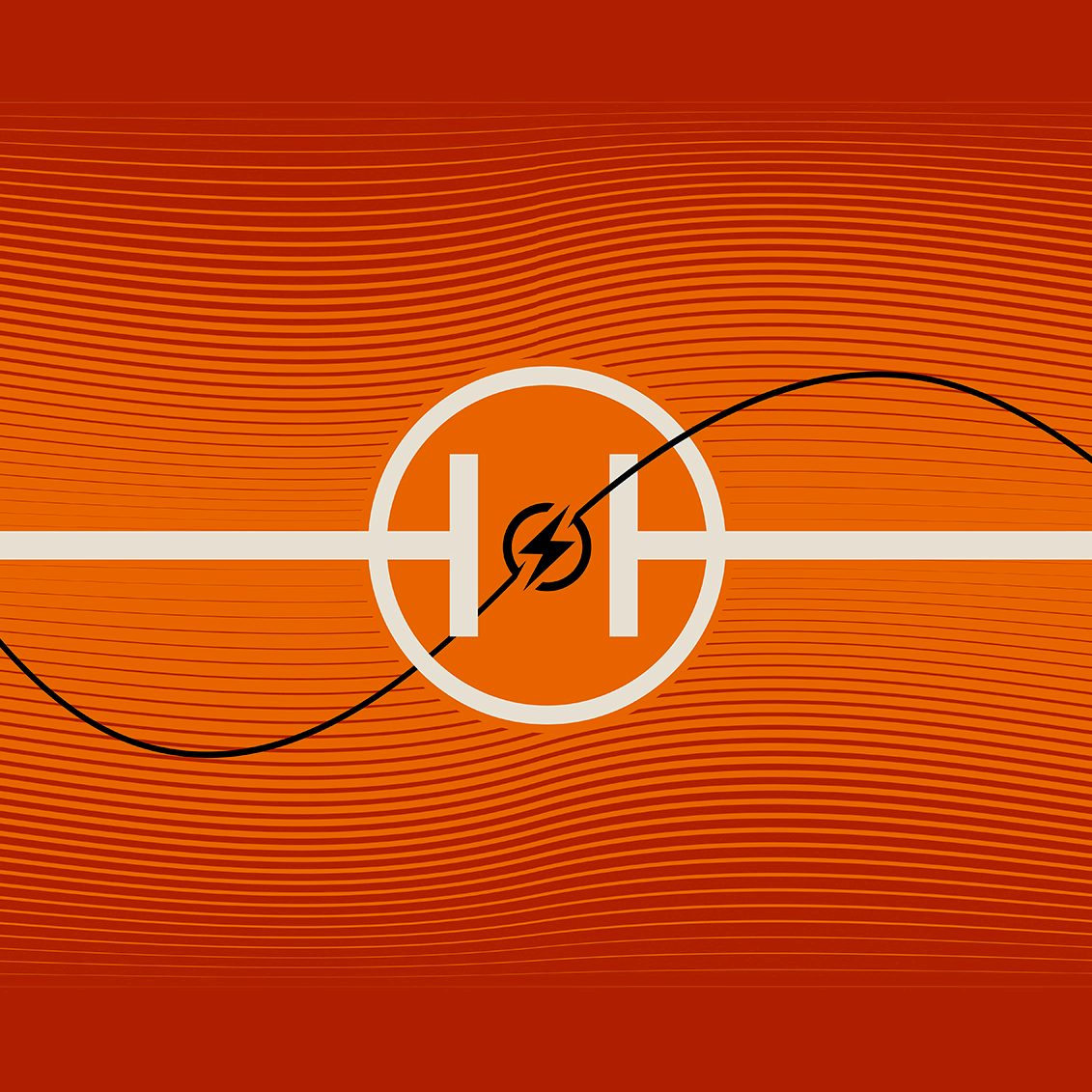
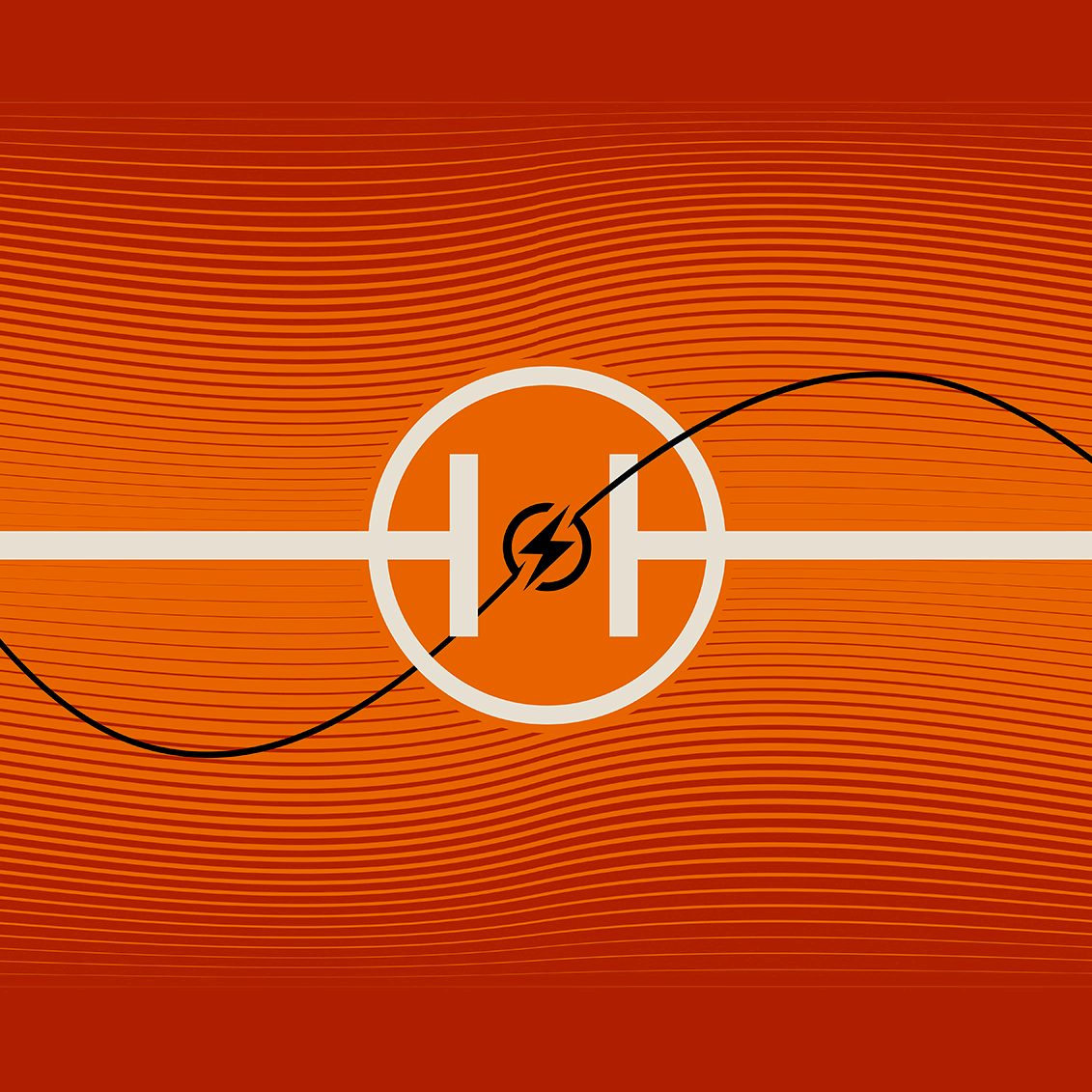
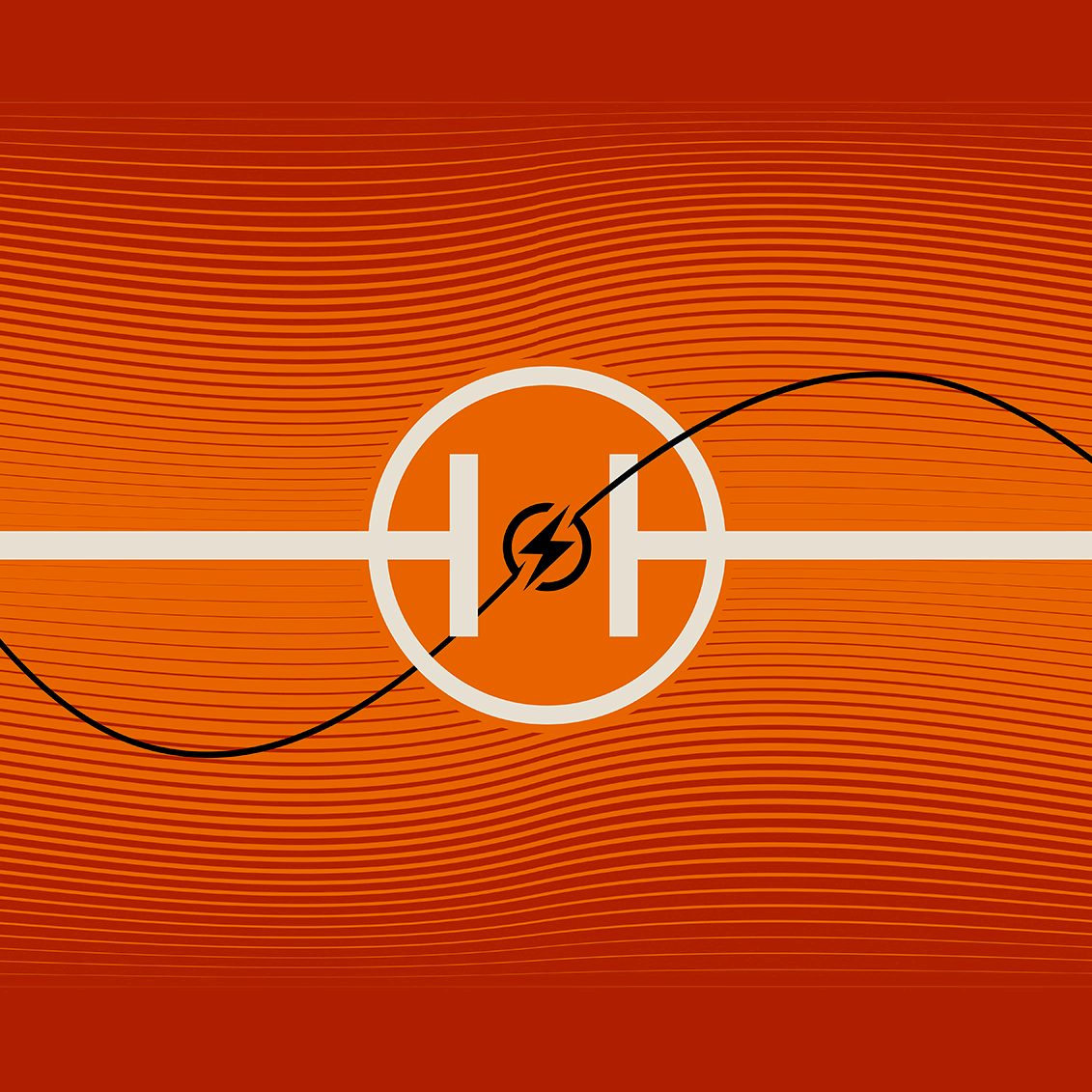
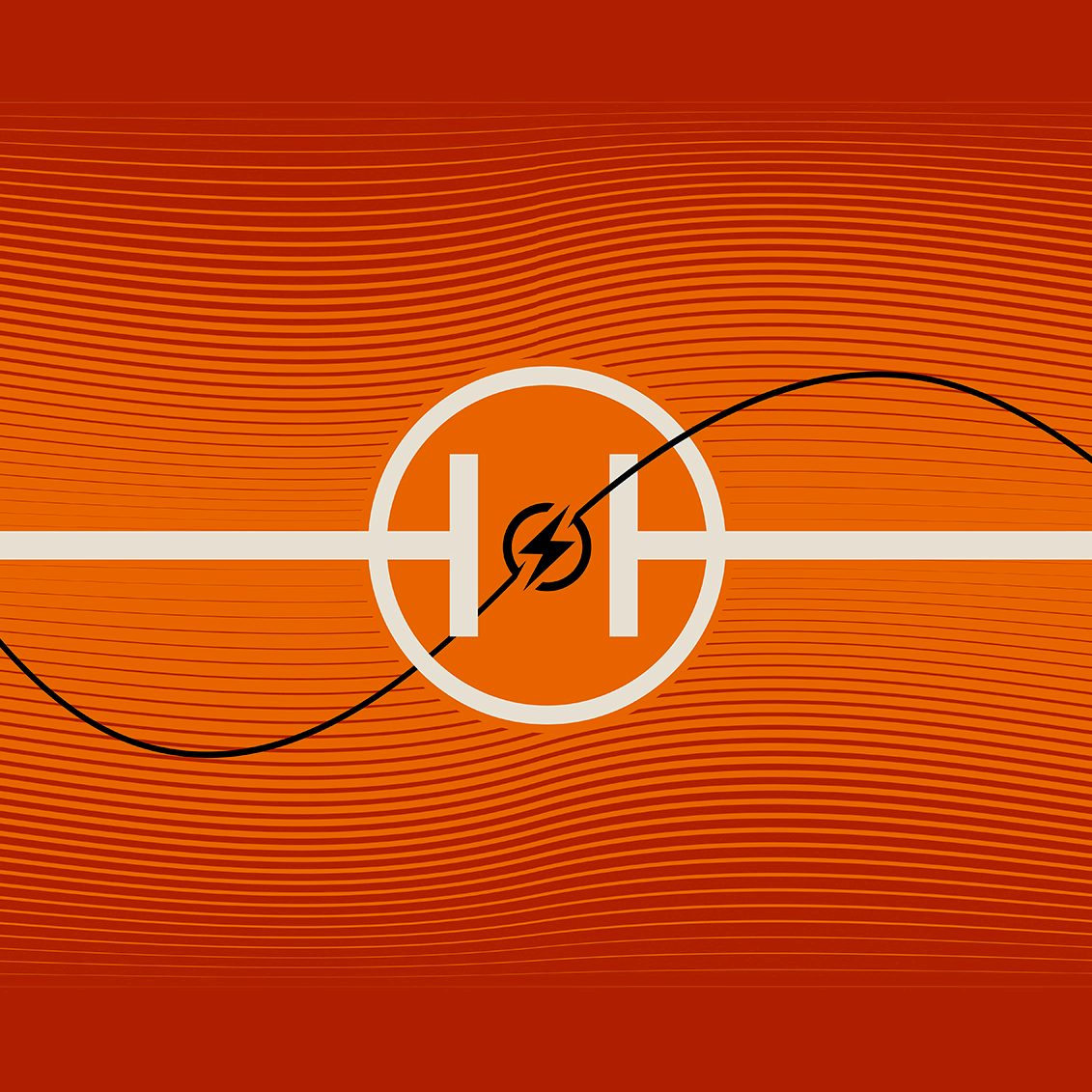
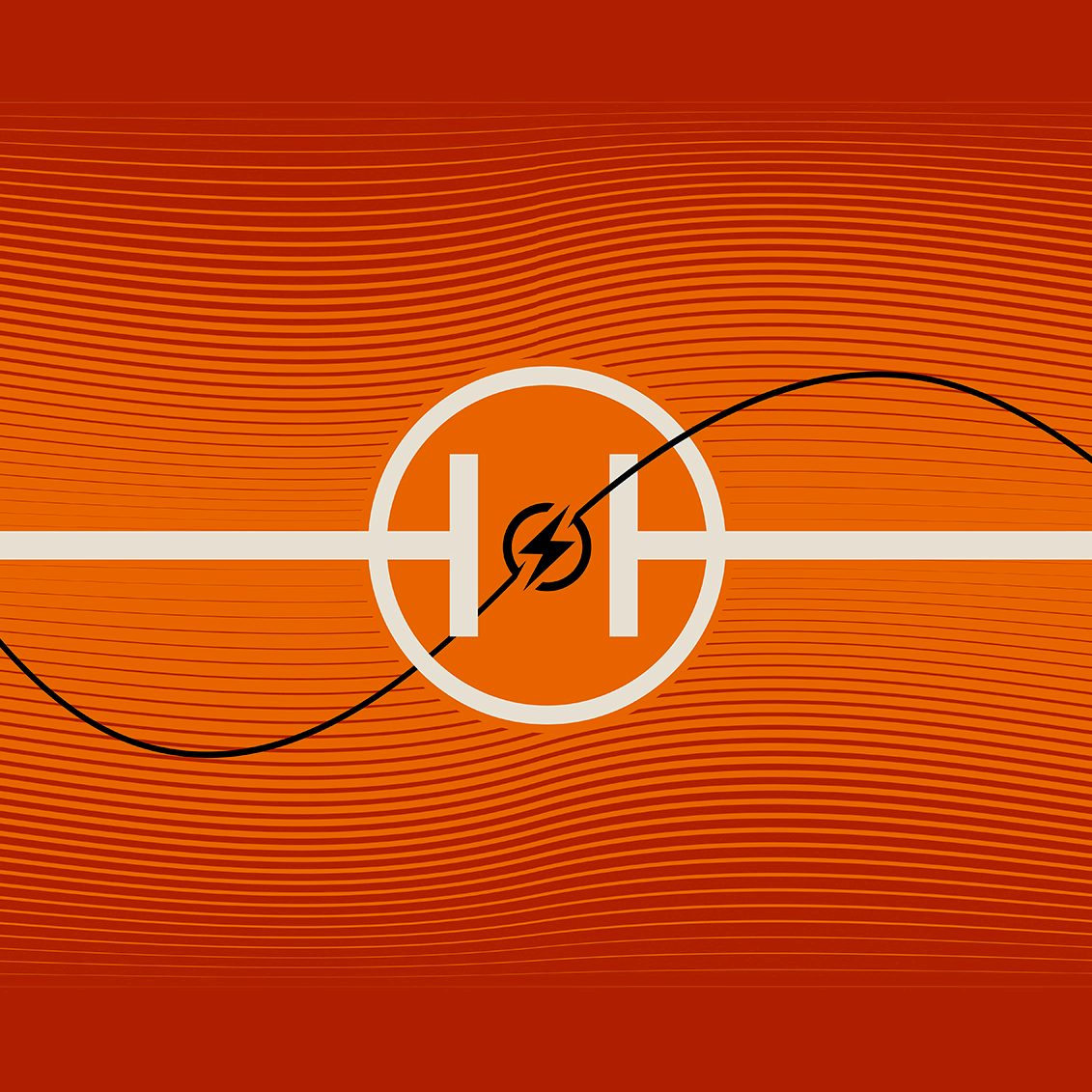
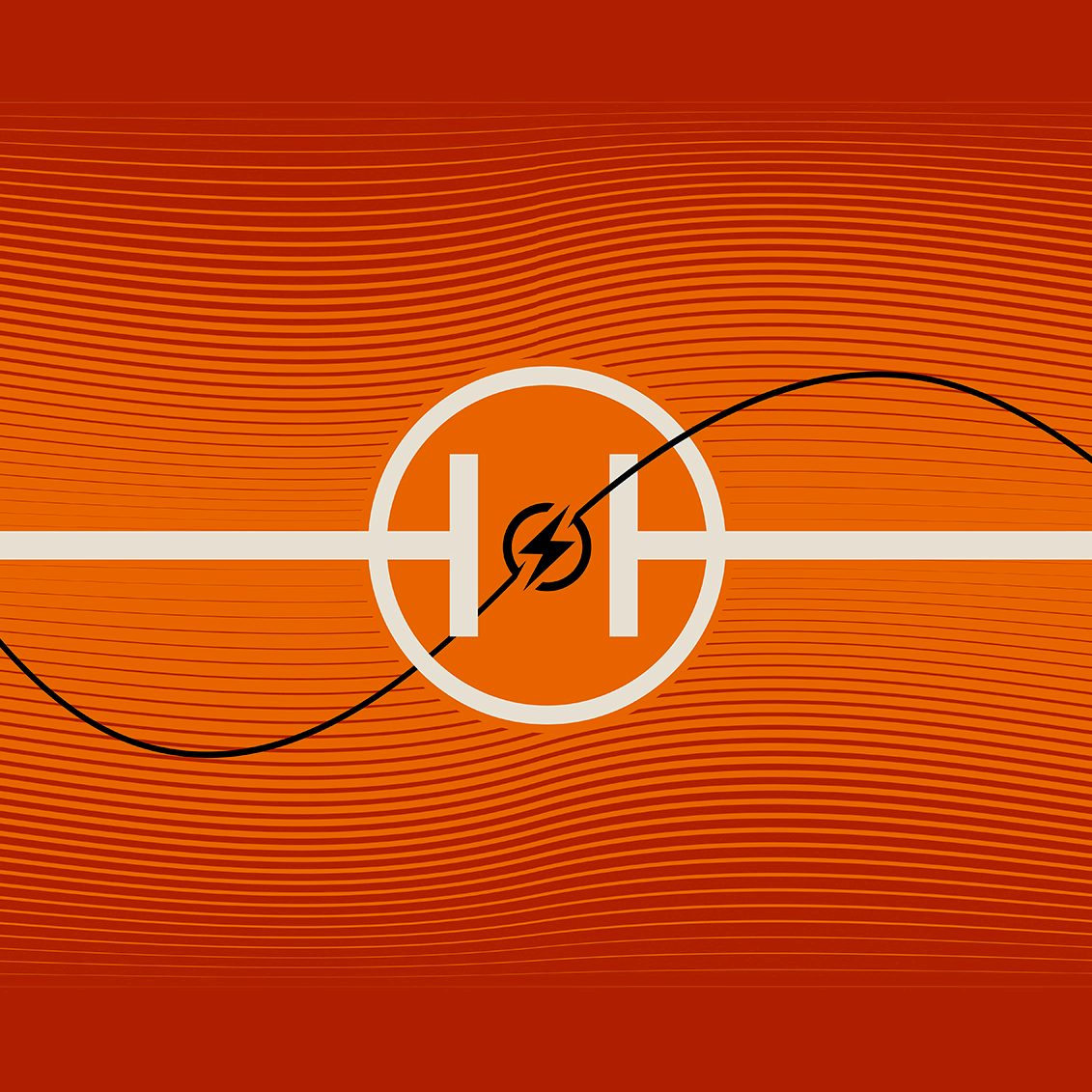
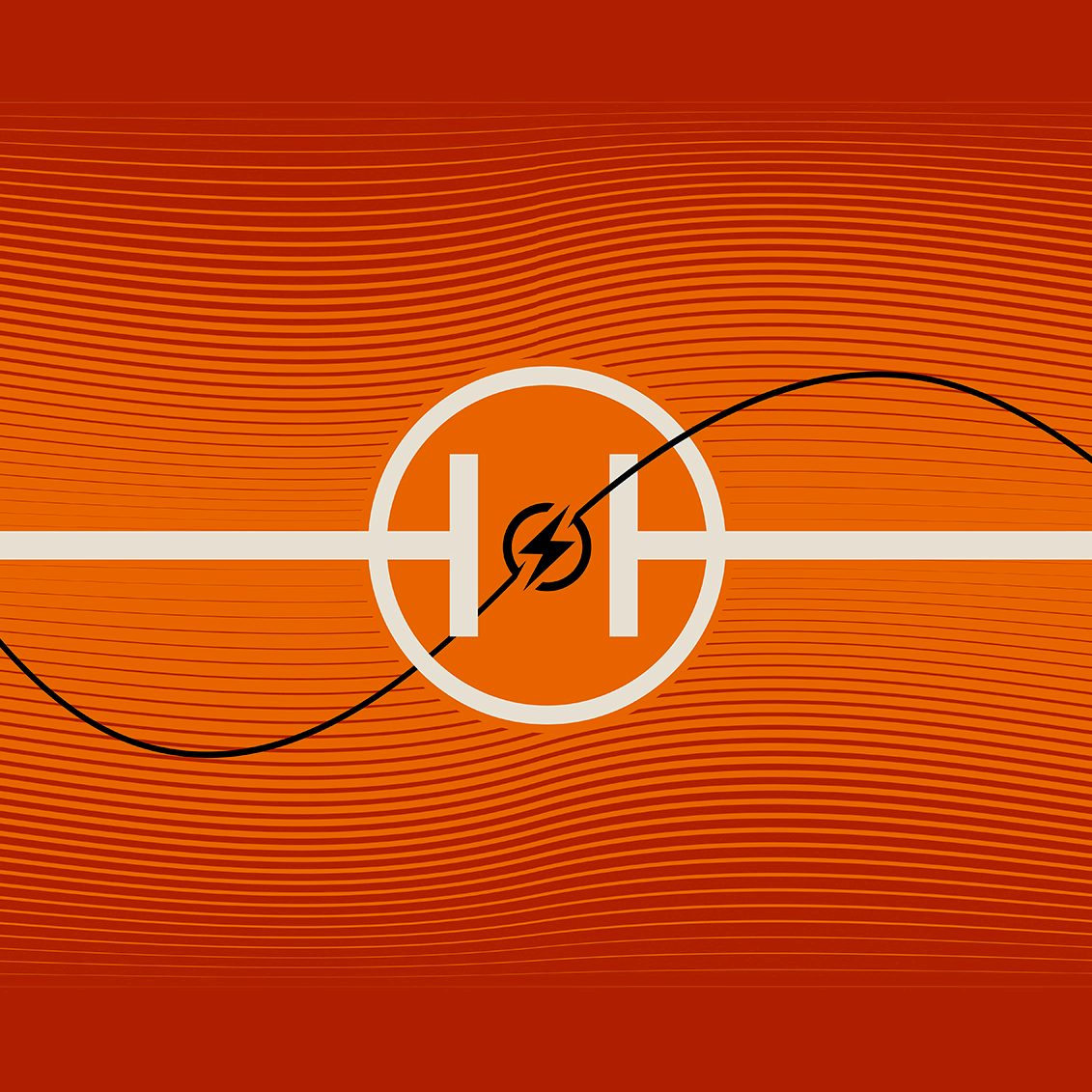
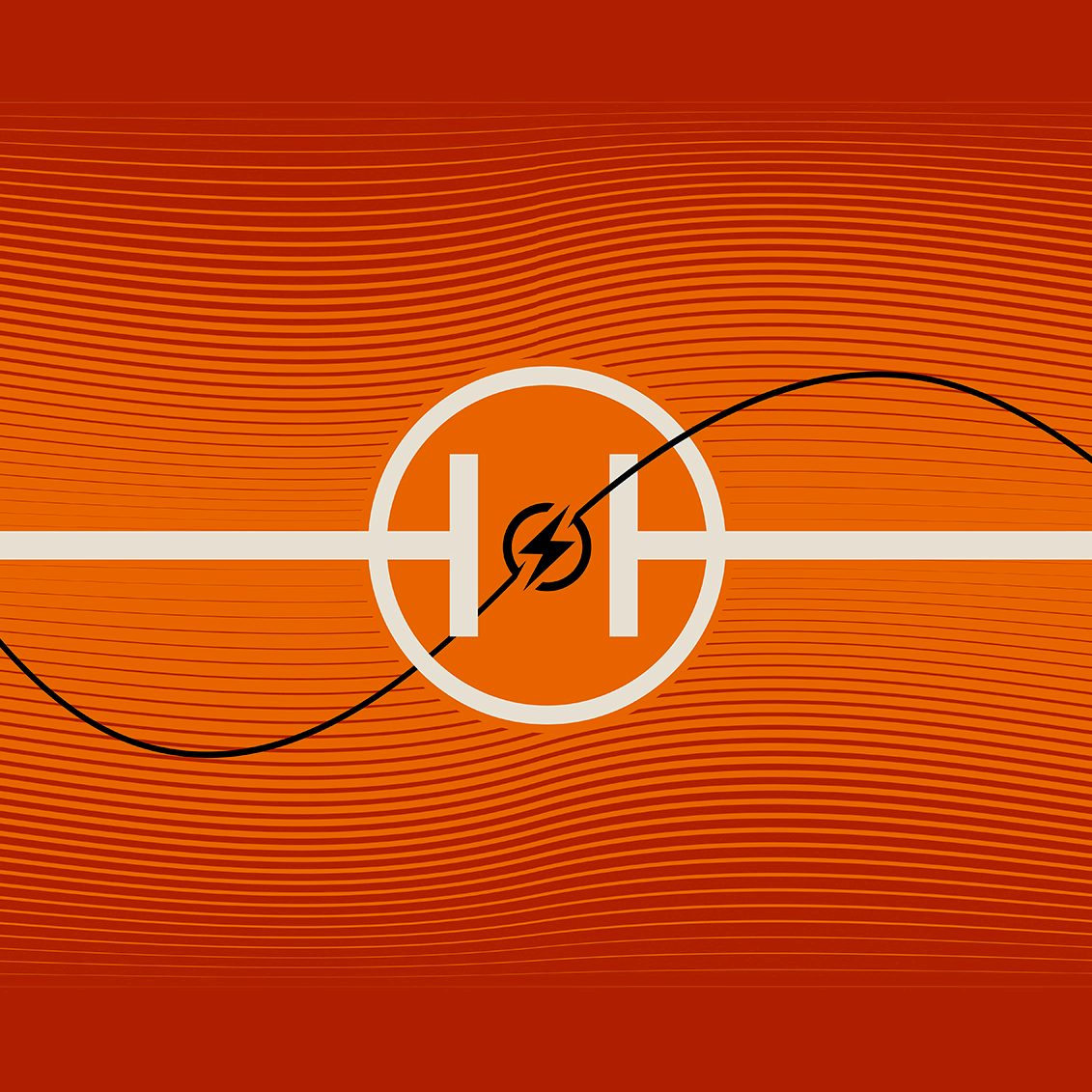
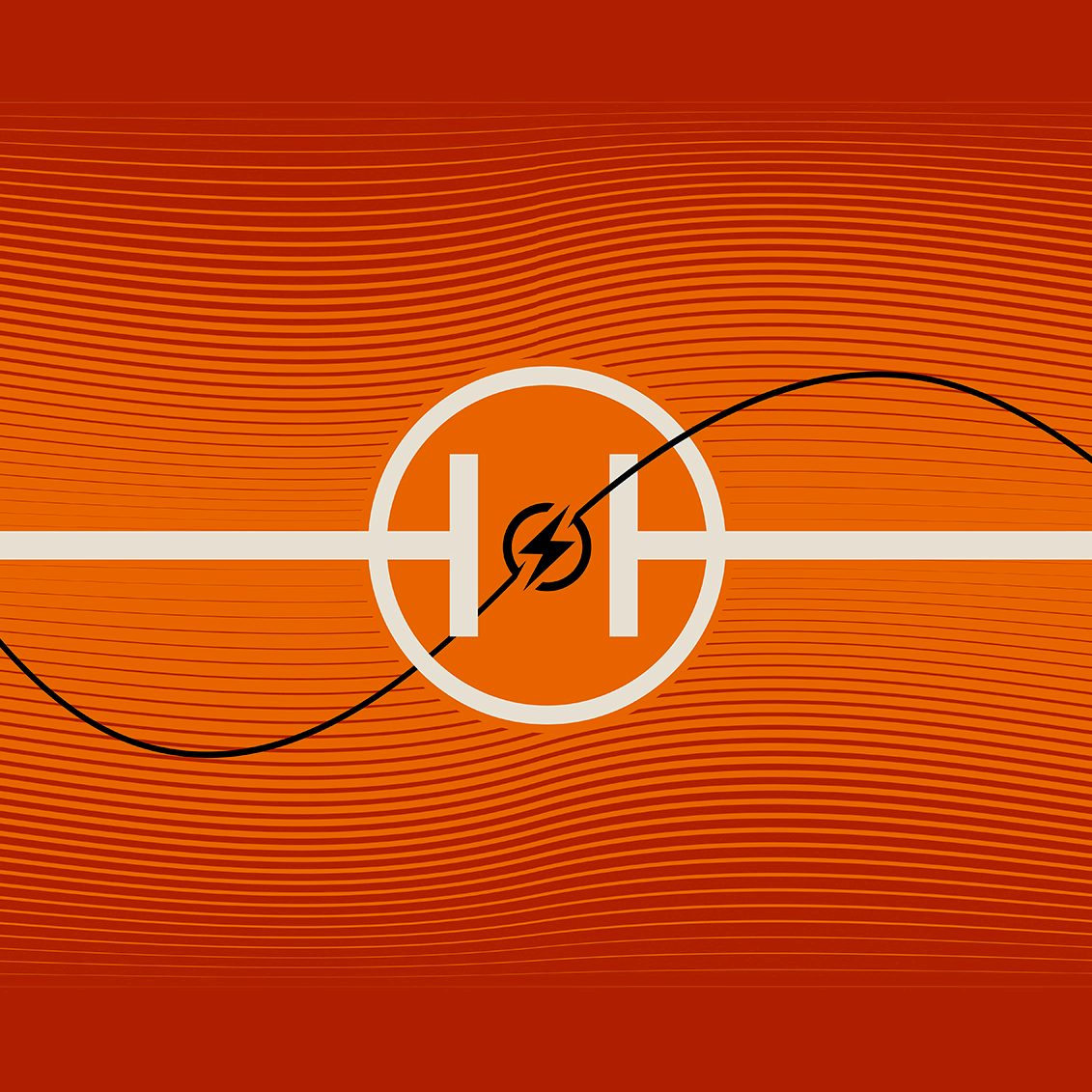
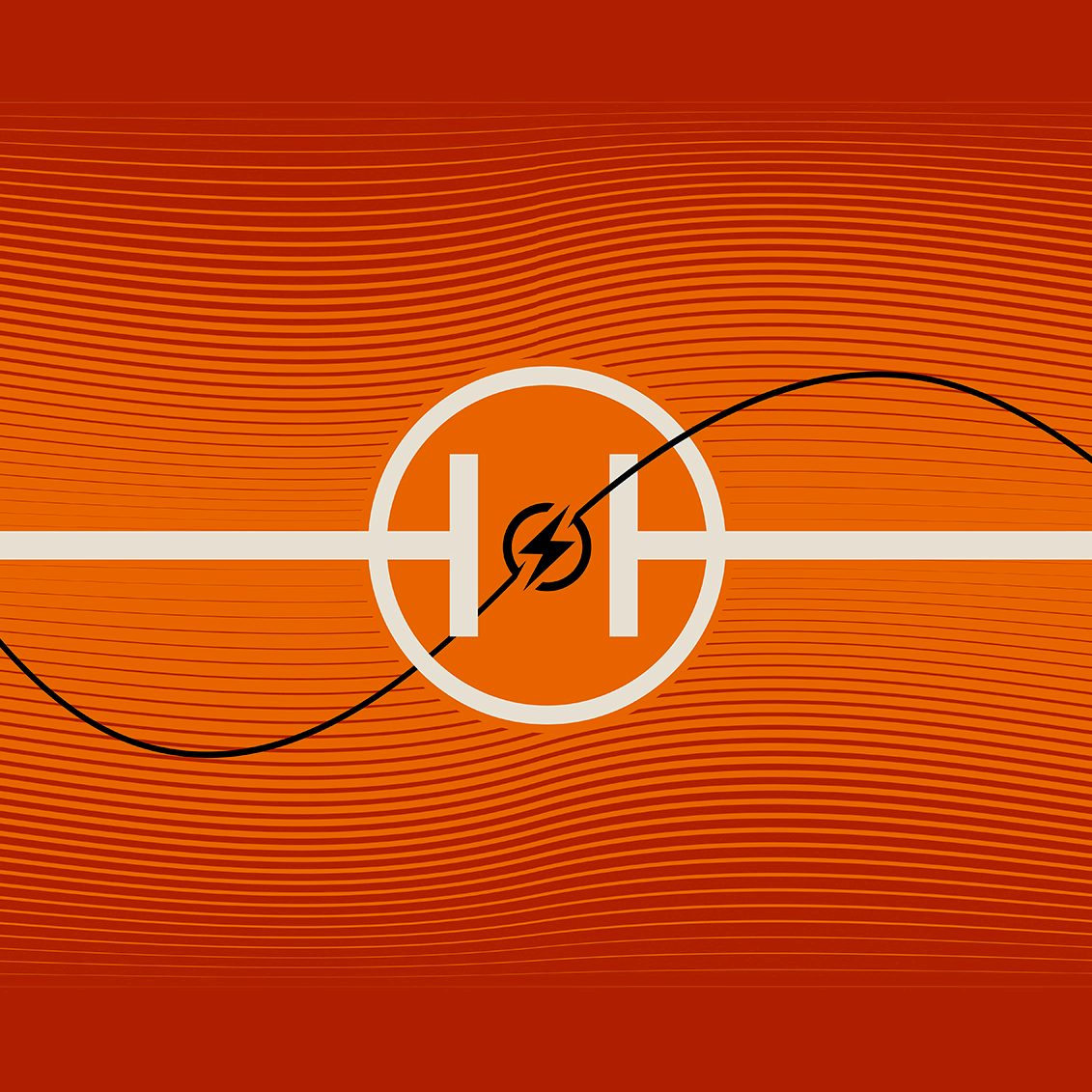
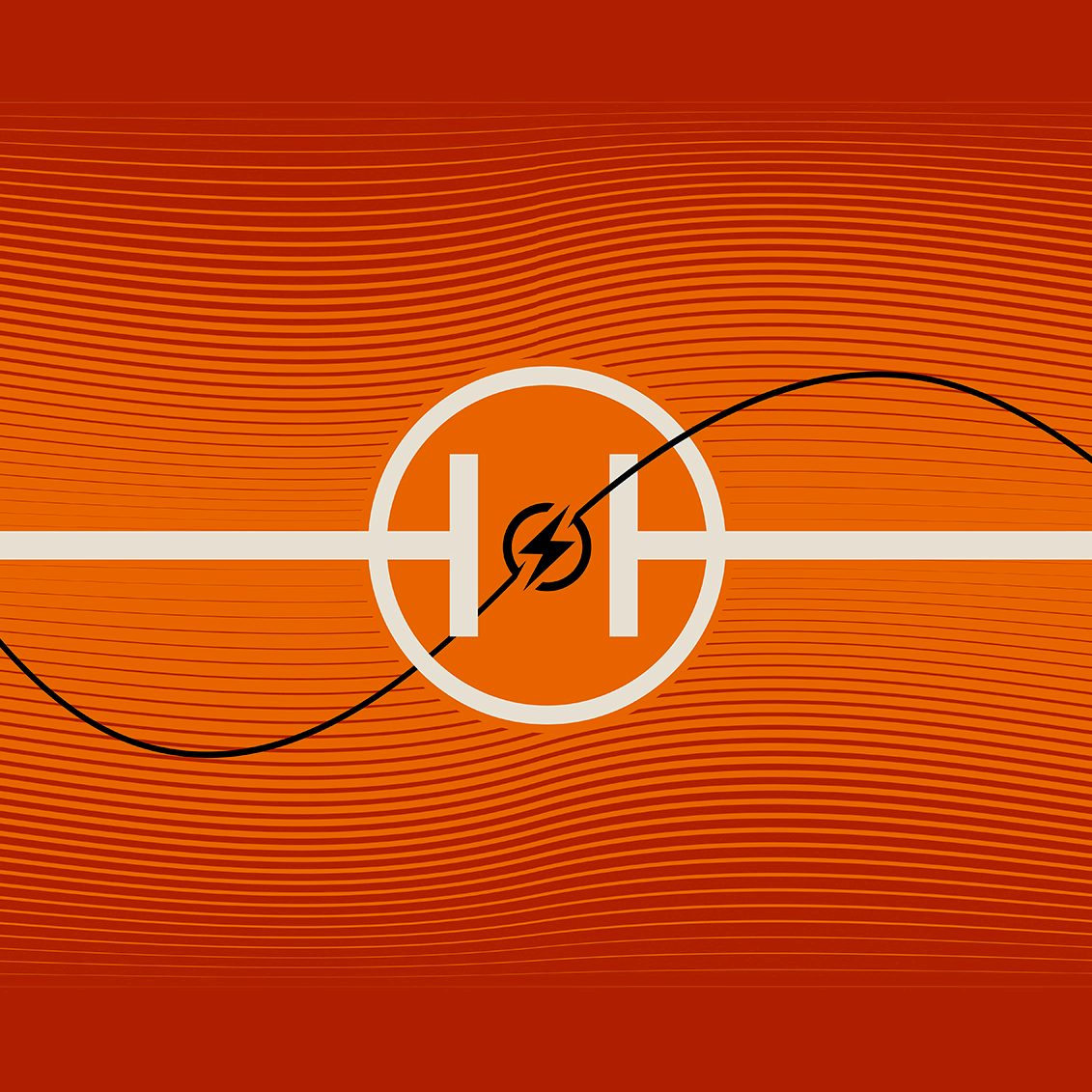
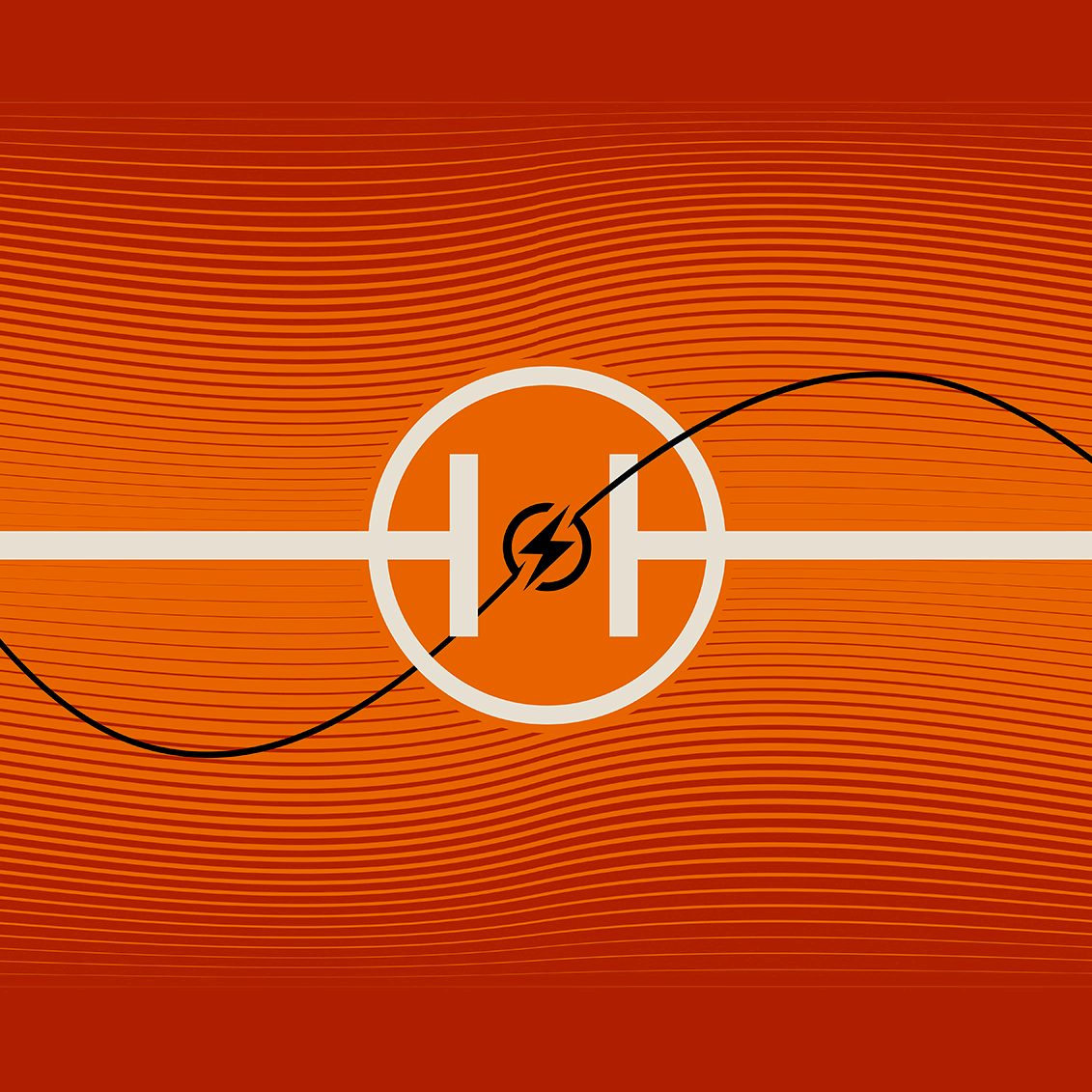
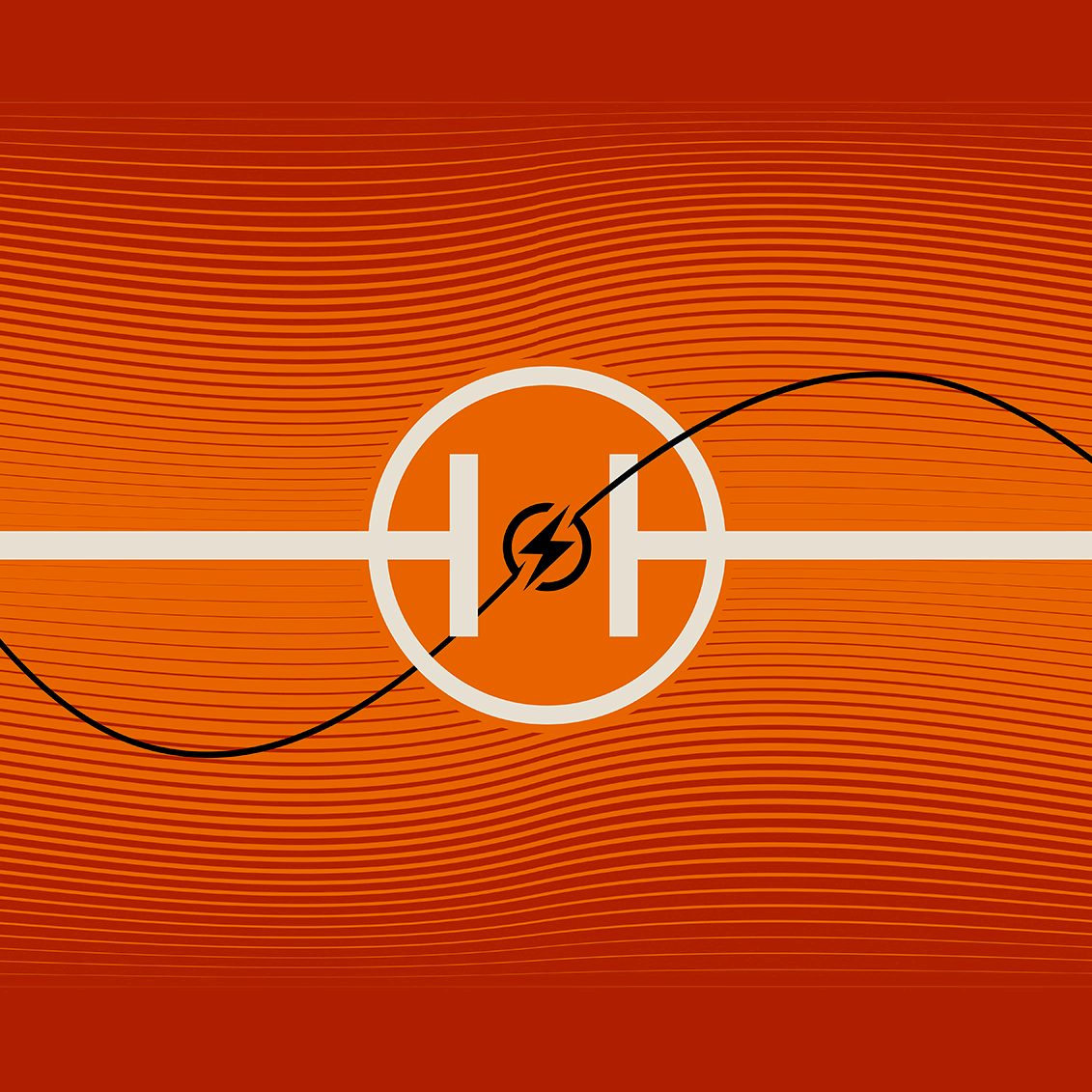
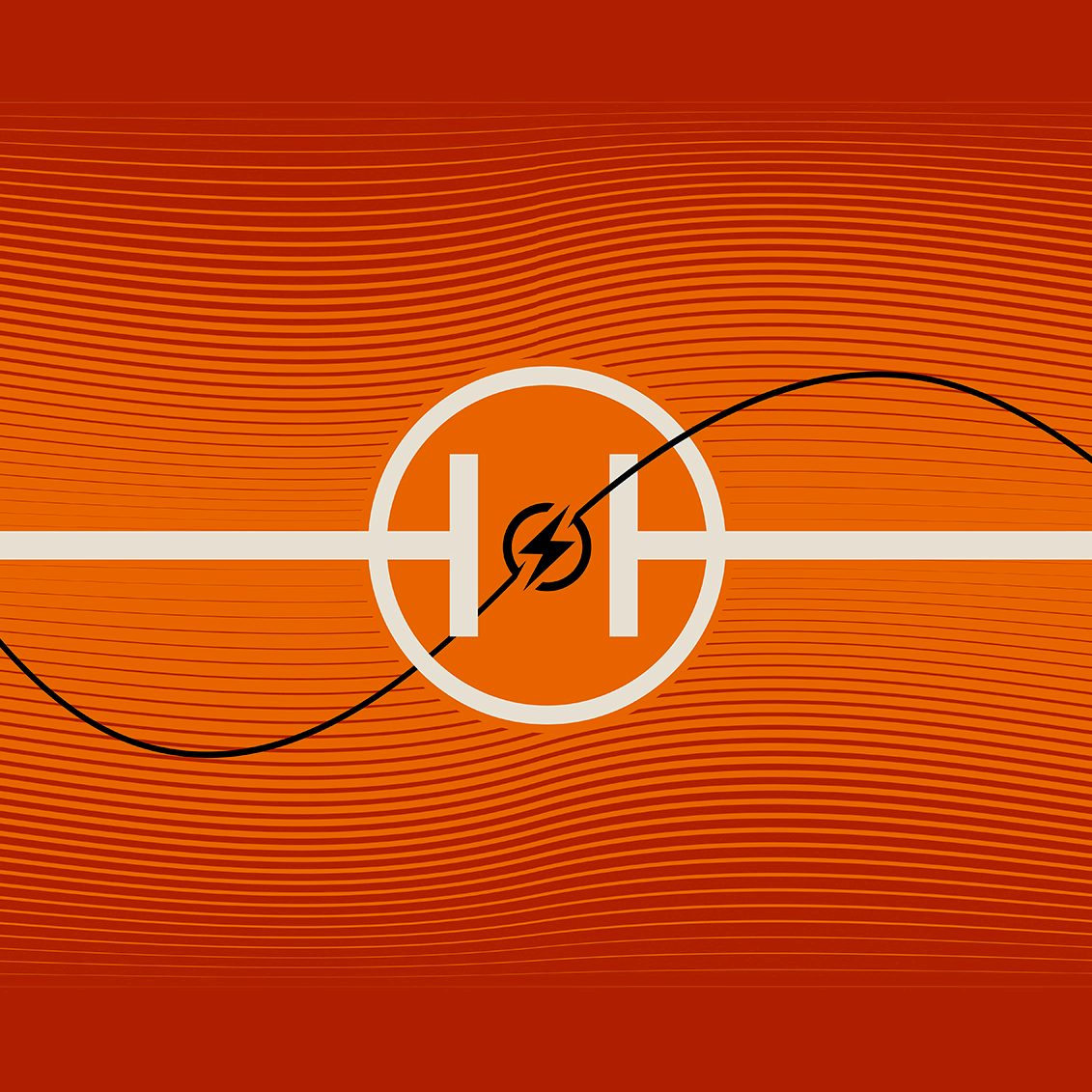
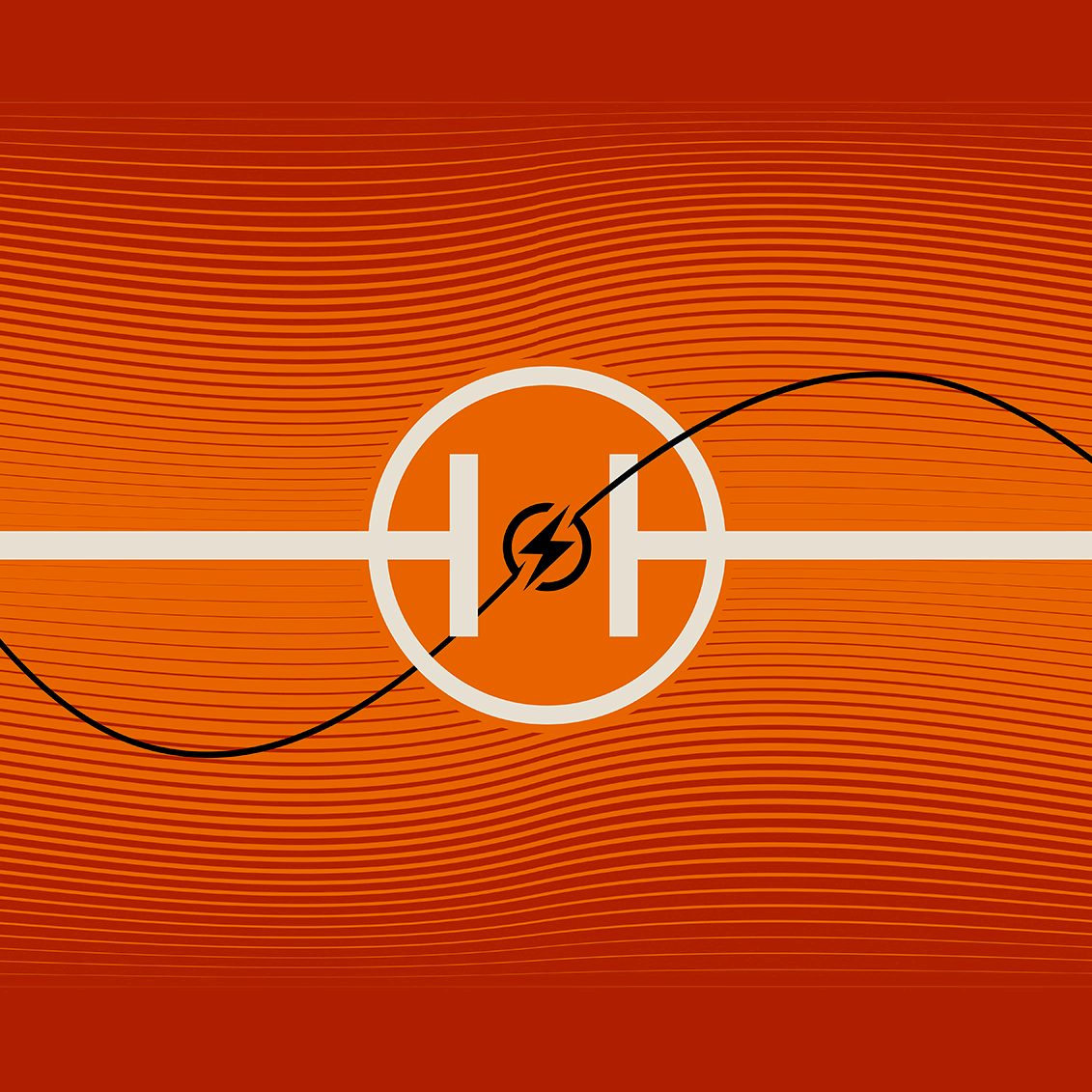
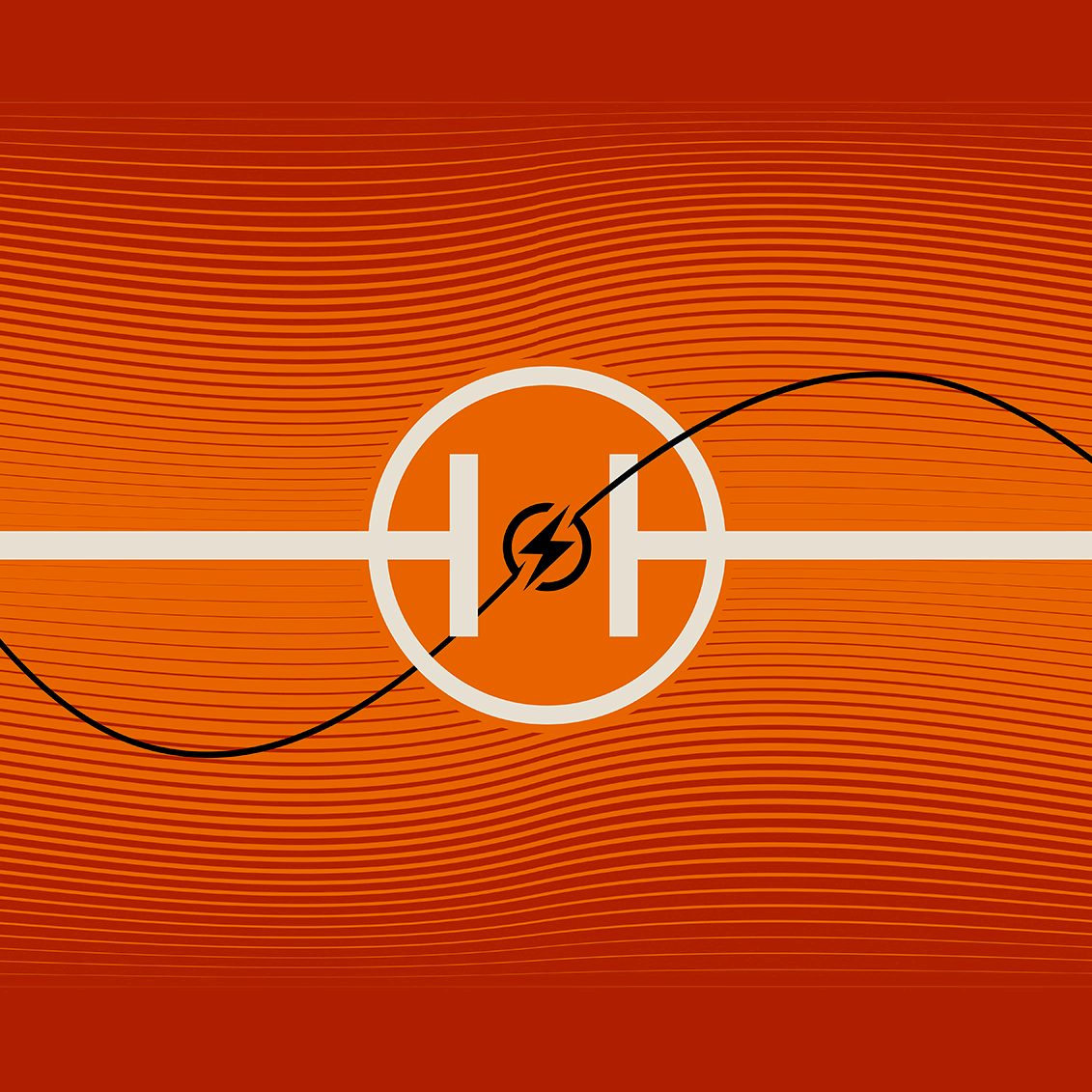
Papers in this project
Memristive networks
A simple solvable model of memristive networks suggests a correspondence between the asymptotic states of memristors and the Ising model.
Solvable memristive circuits
Exact solutions for the dynamics of interacting memristors predict whether they relax to higher or lower resistance states given random initialisations.
Memristive networks and learning
Memristive networks preserve memory and have the ability to learn according to analysis of the network’s internal memory dynamics.
Dynamics of memristors
Exact equations of motion provide an analytical description of the evolution and relaxation properties of complex memristive circuits.
From memory to scale-free
A local model of preferential attachment with short-term memory generates scale-free networks, which can be readily computed by memristors.