The mise en scene of memristive networks: effective memory, dynamics and learning
Memristive networks preserve memory and have the ability to learn according to analysis of the network’s internal memory dynamics.
International Journal of Parallel, Emergent and Distributed Systems 33, 350 (2017)
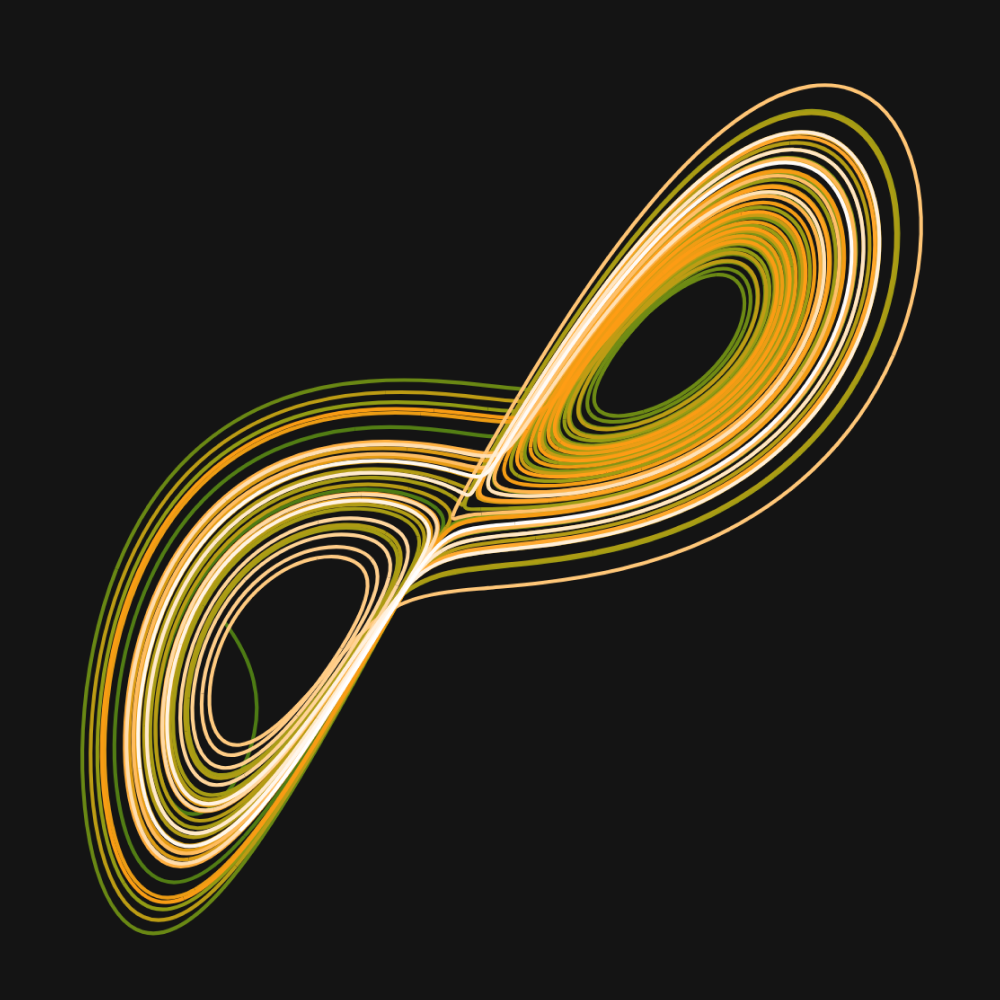
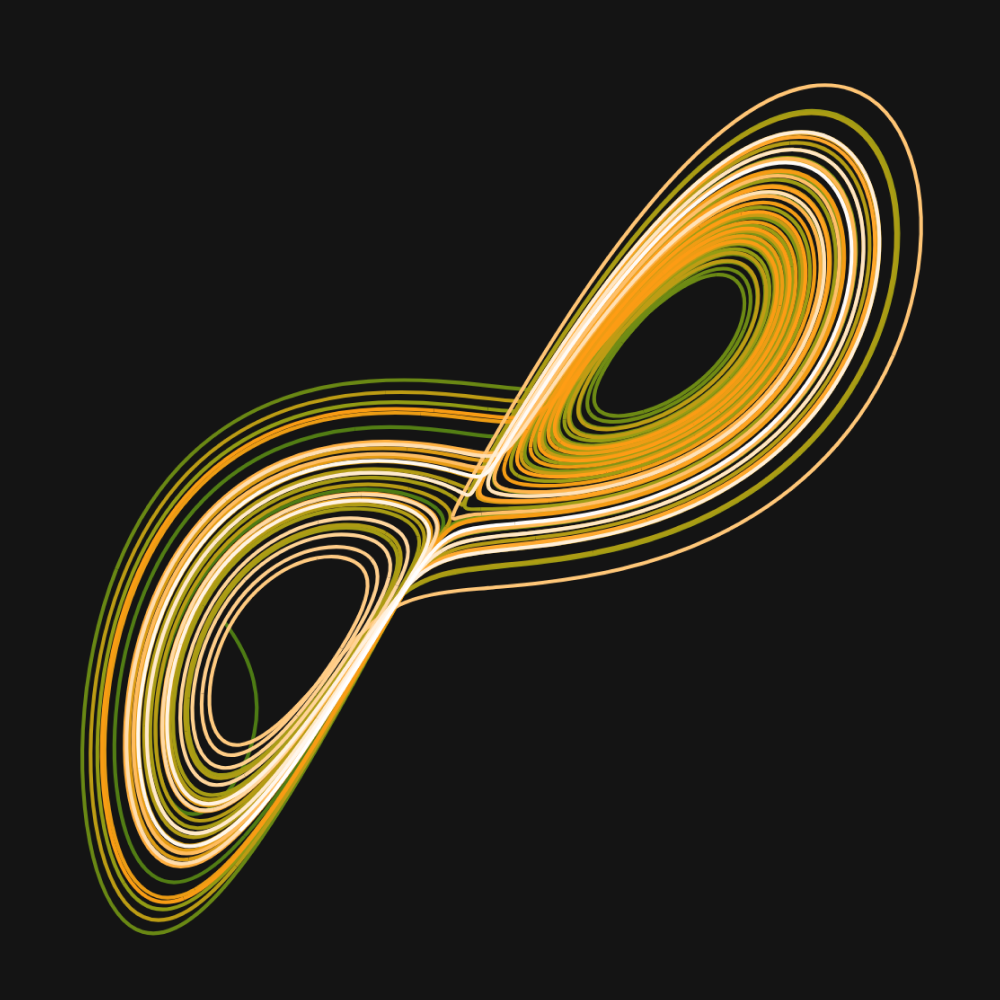
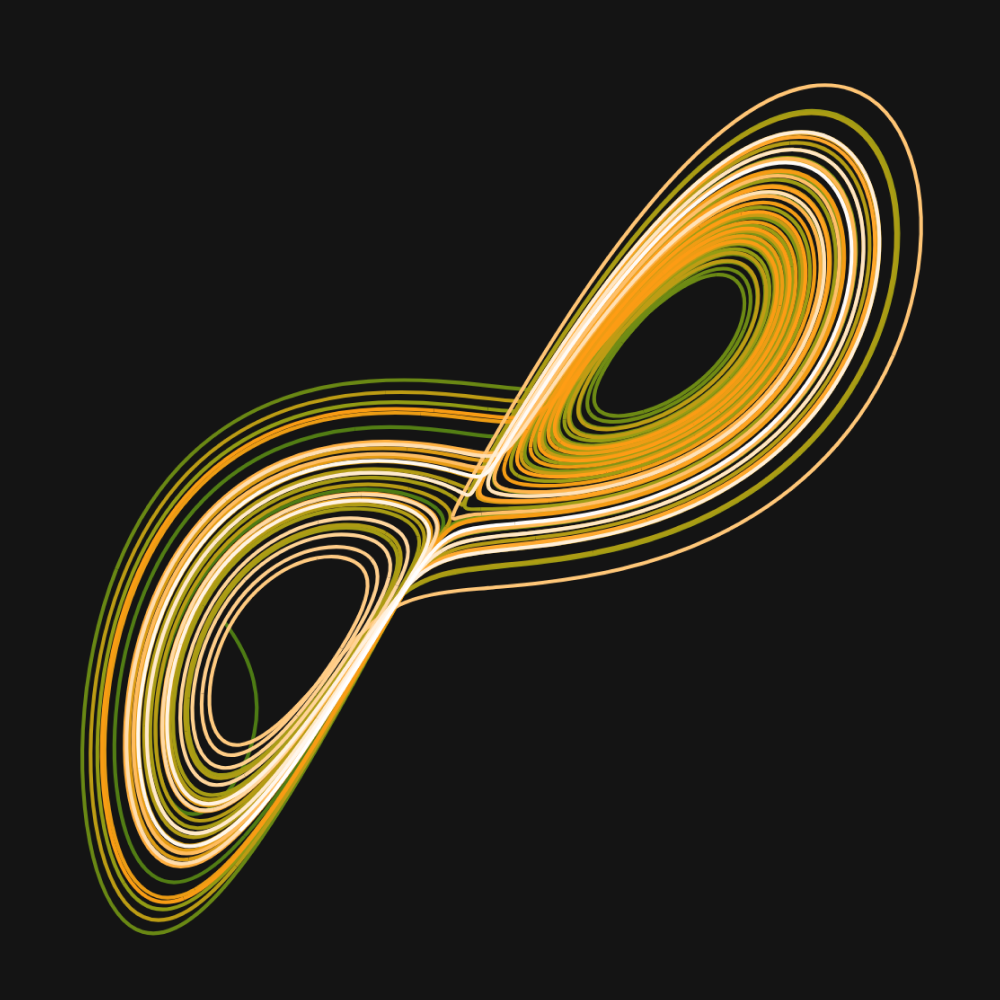
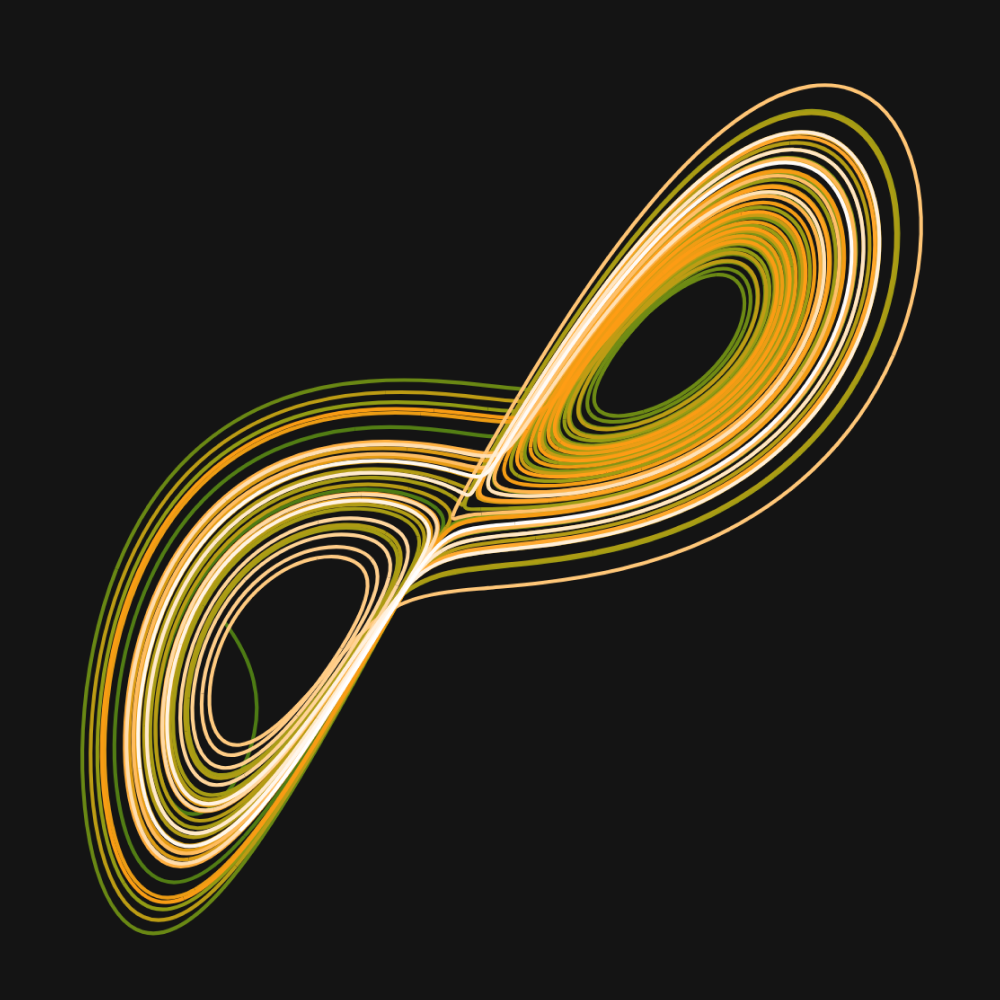
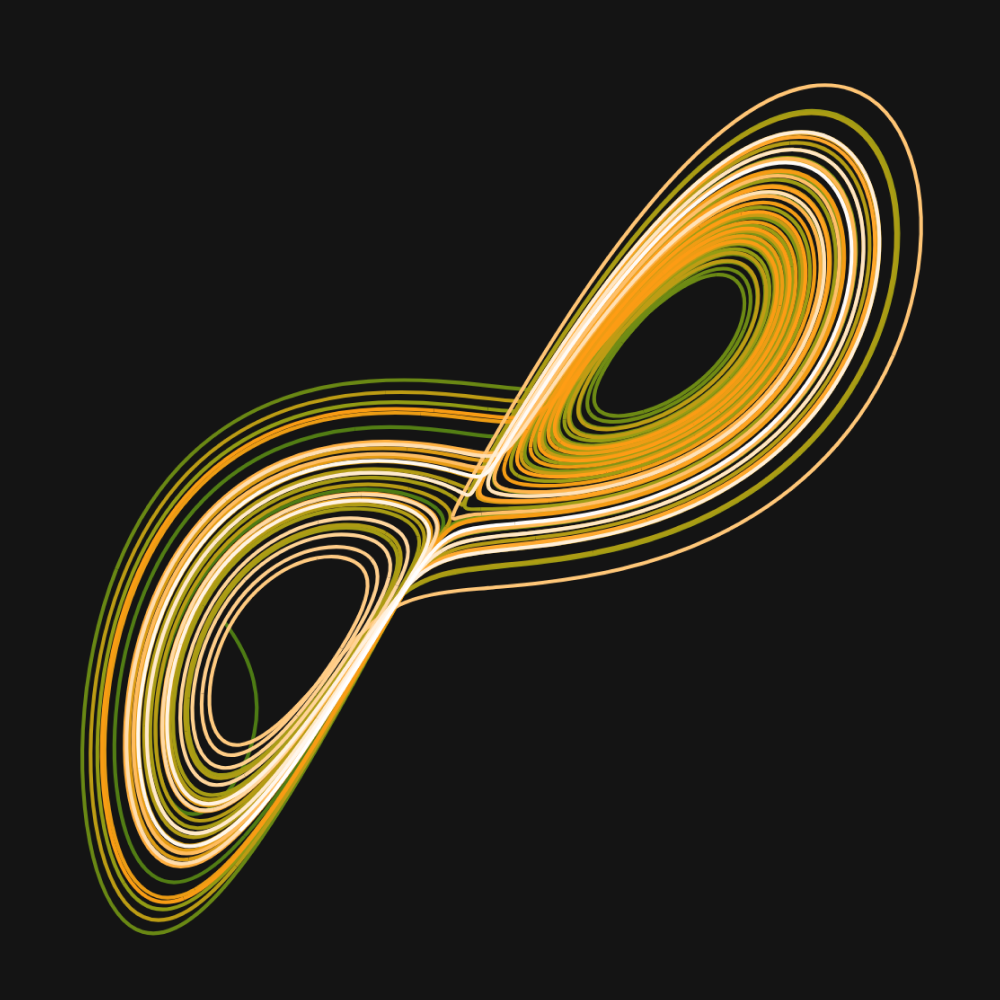
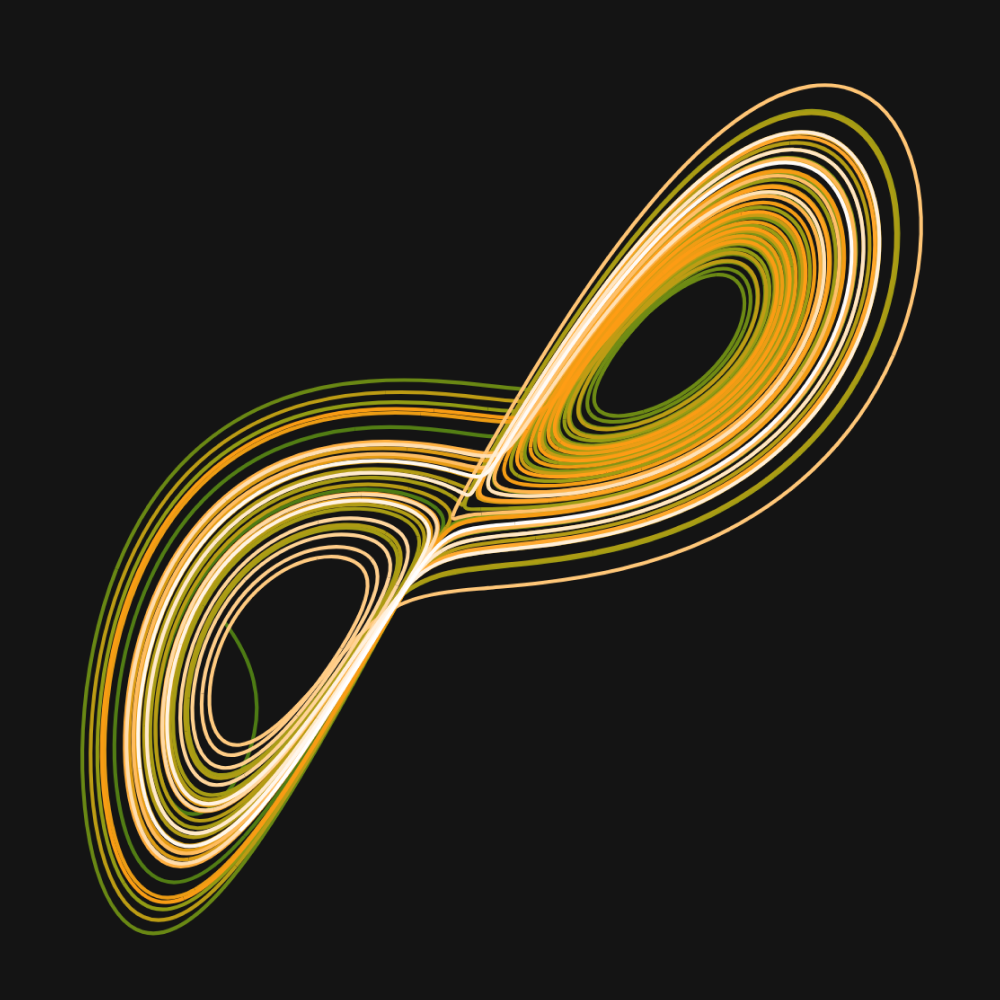
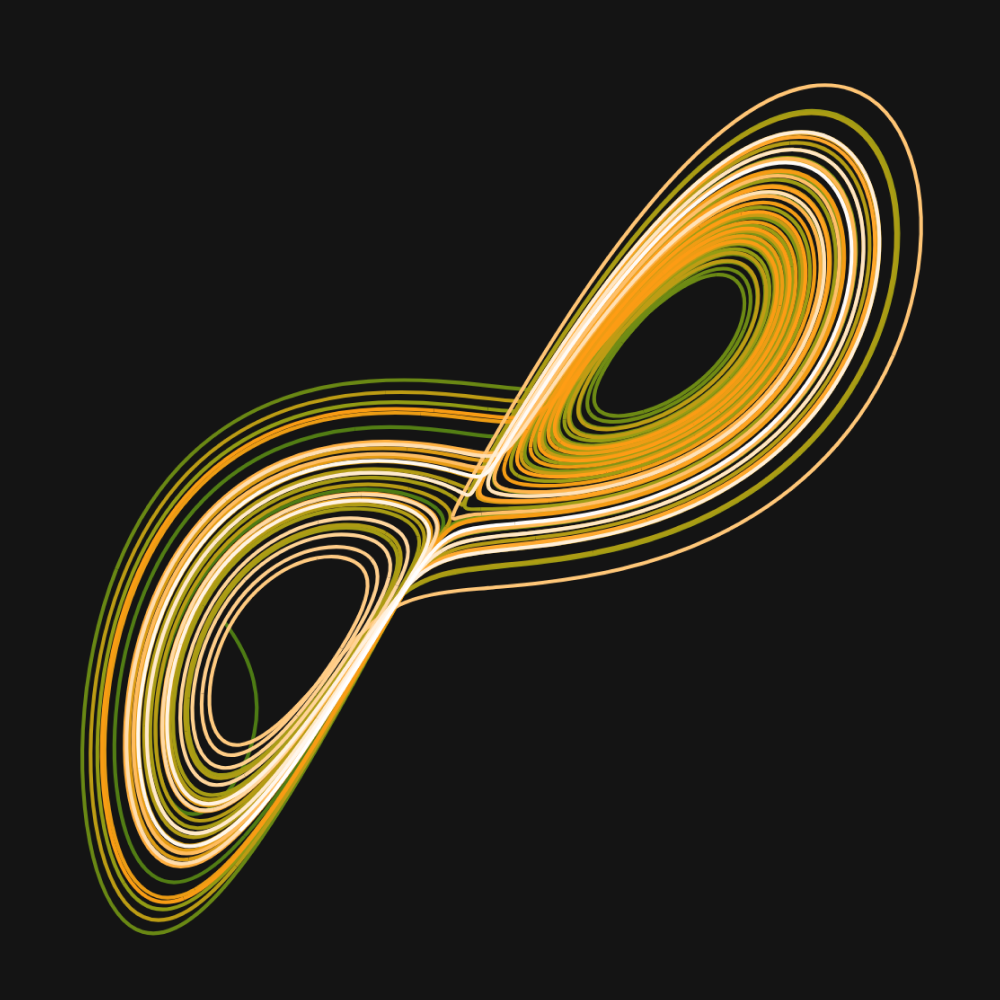
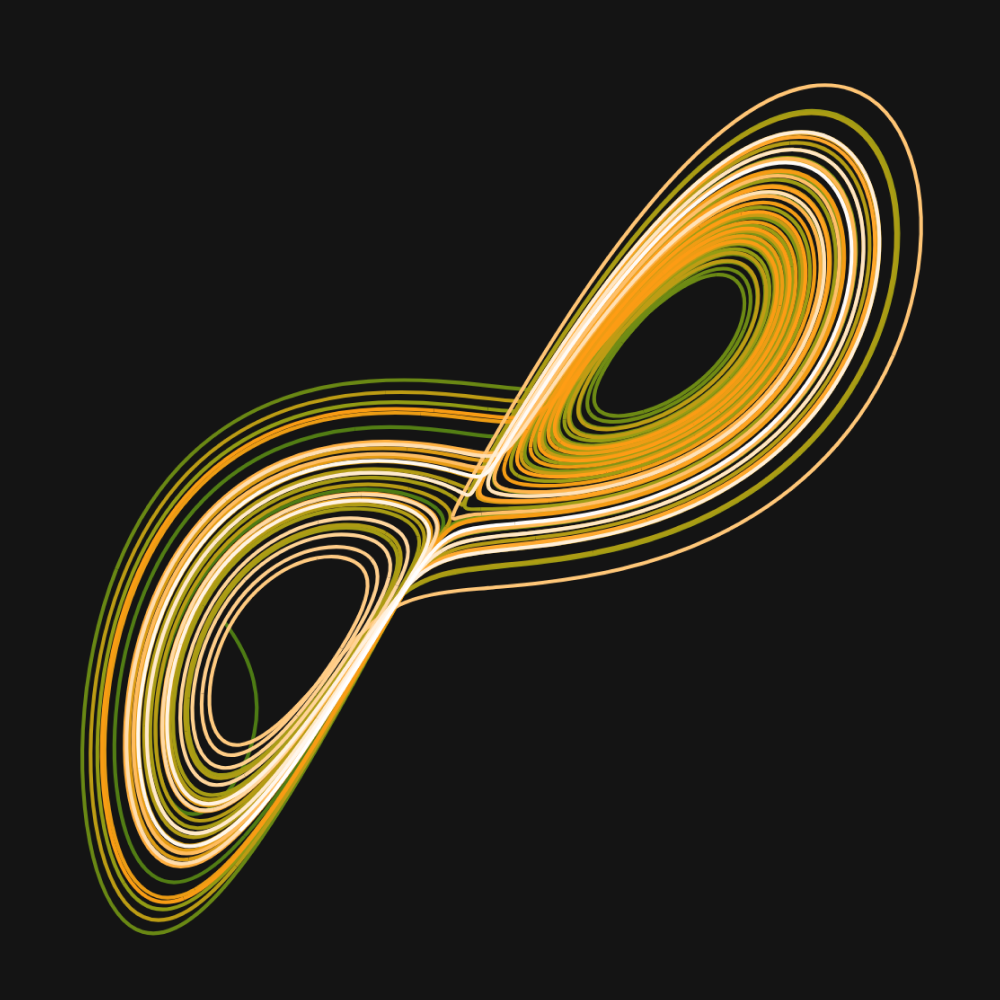
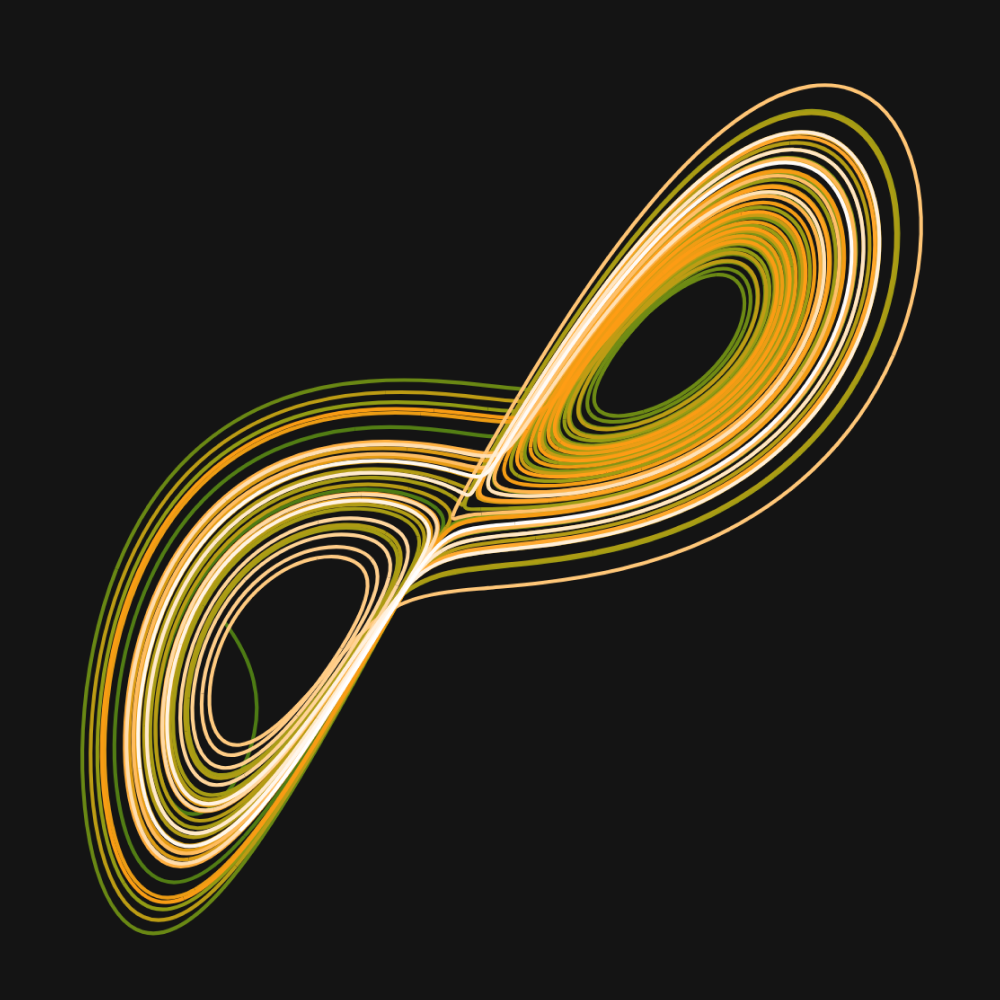
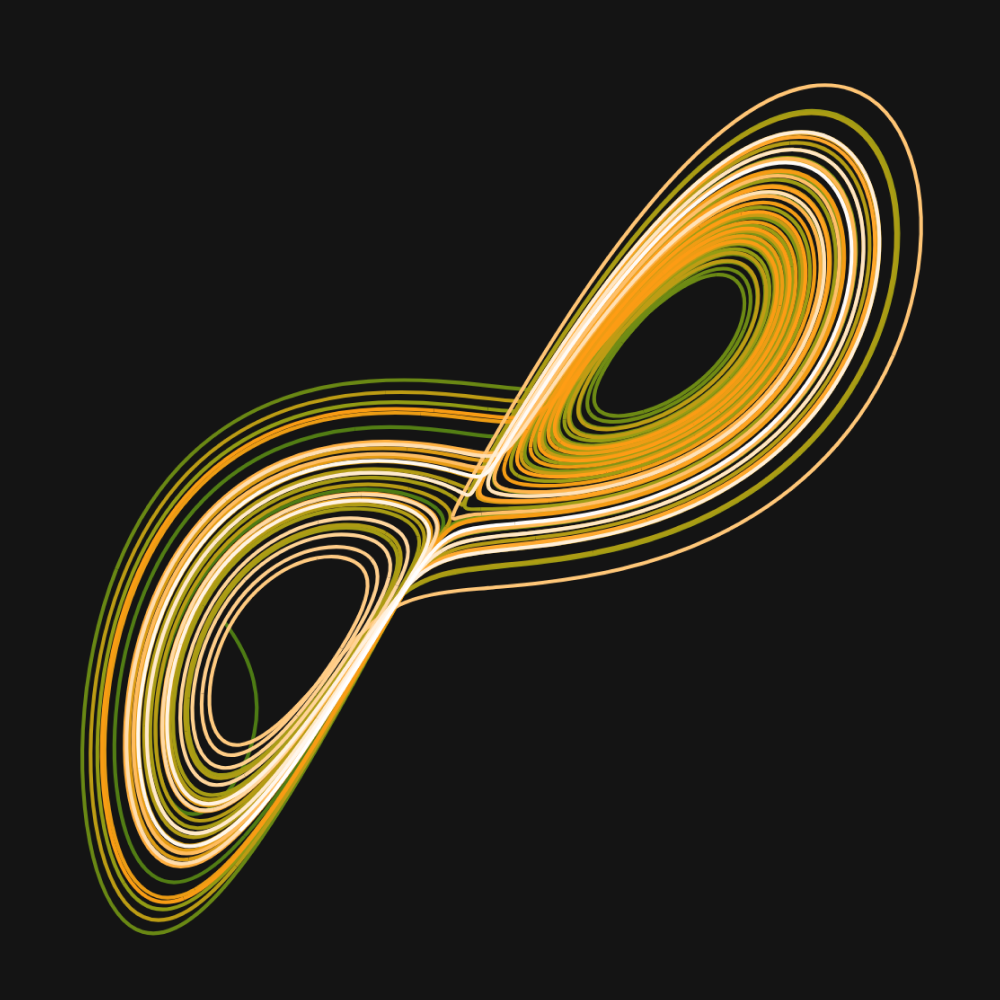
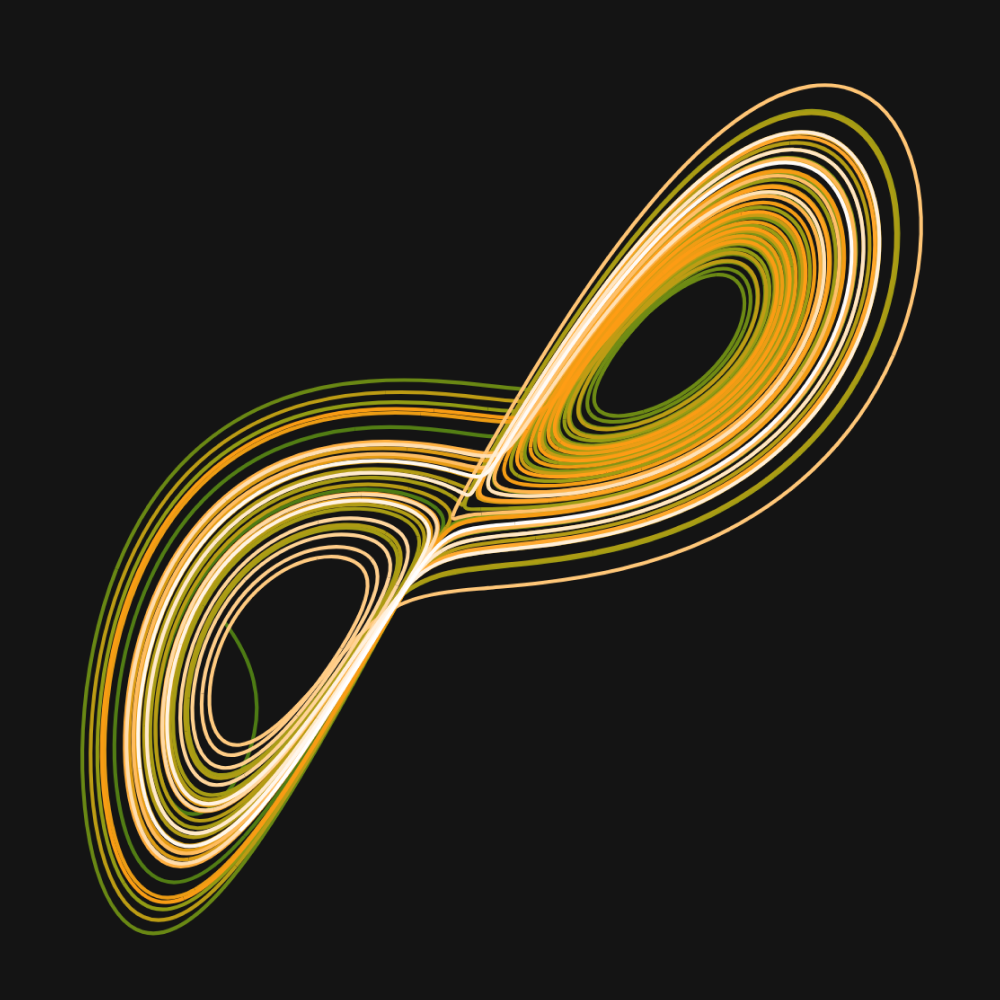
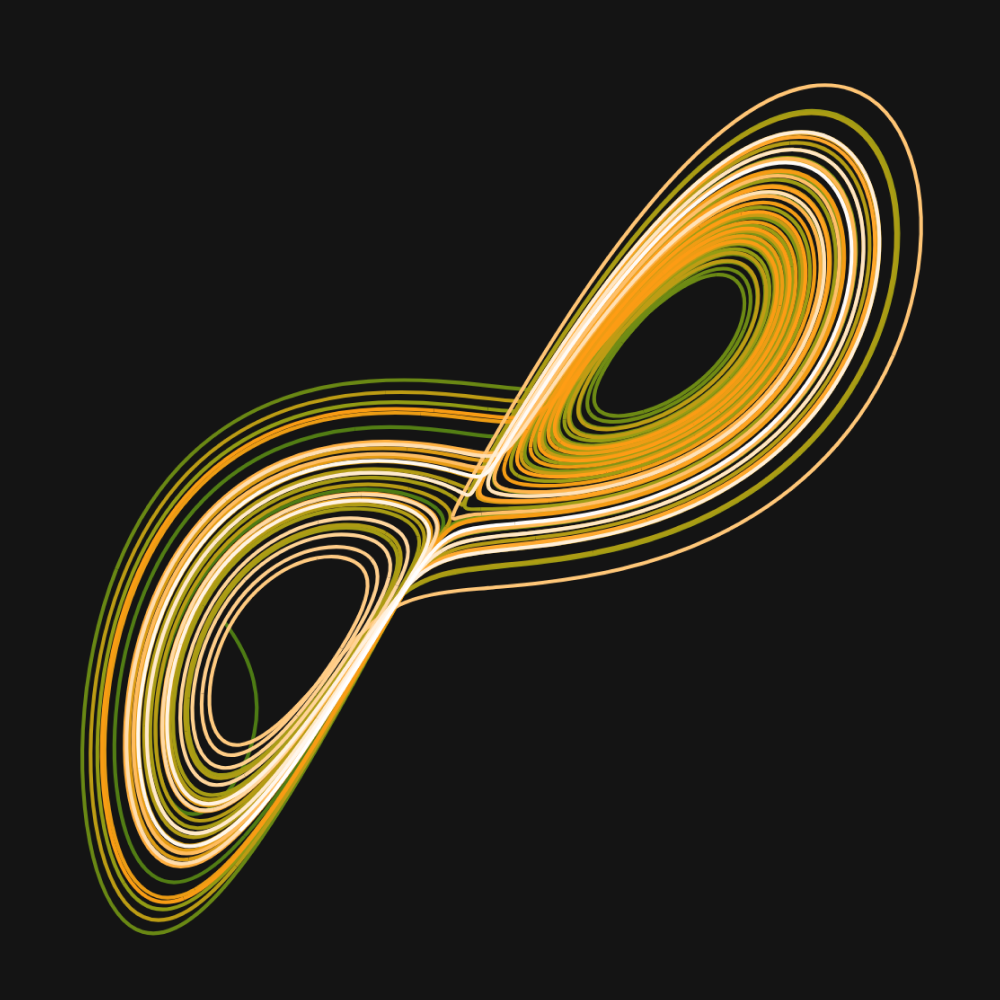
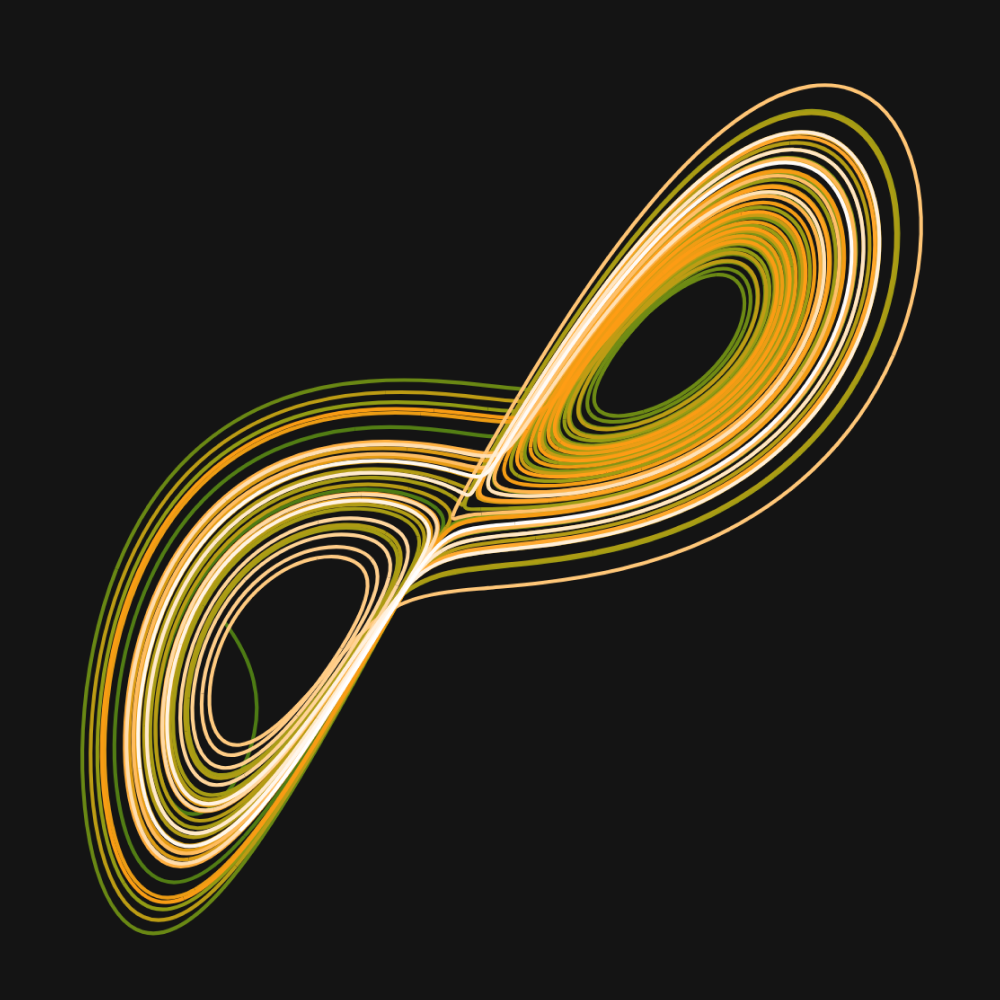
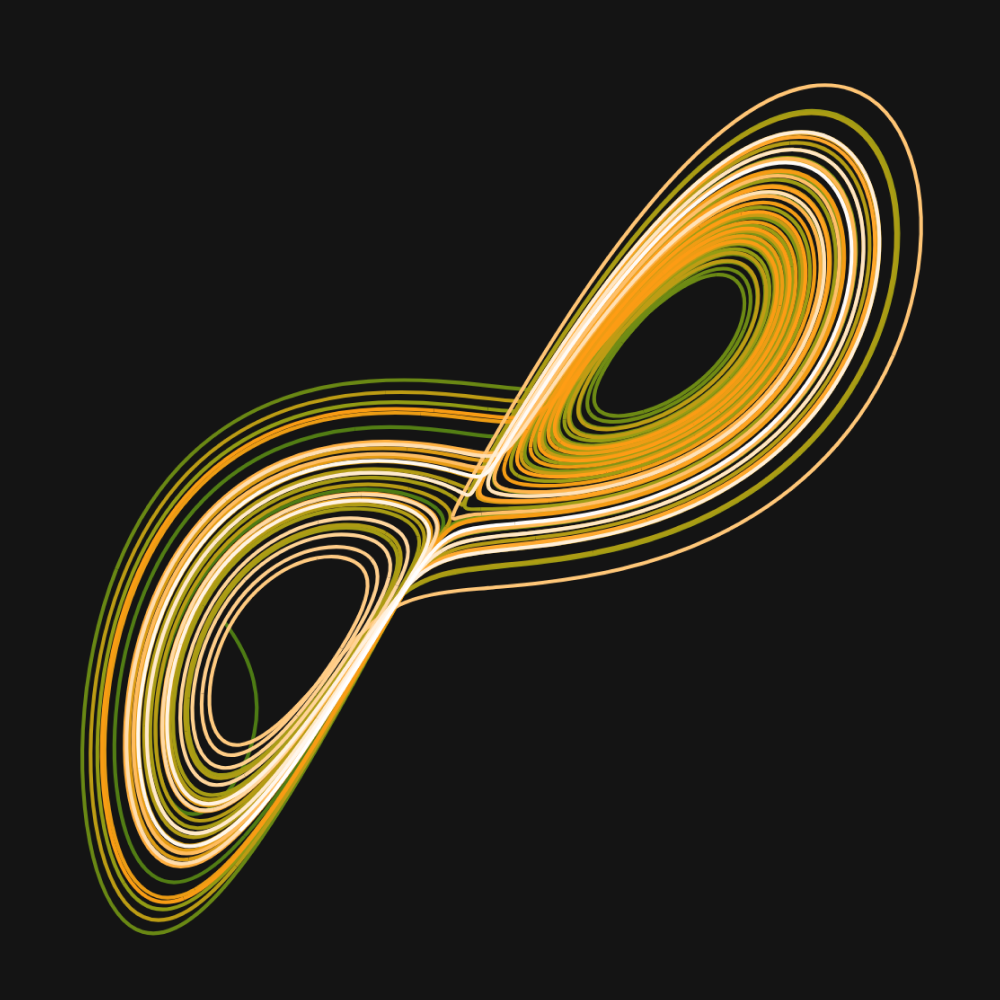
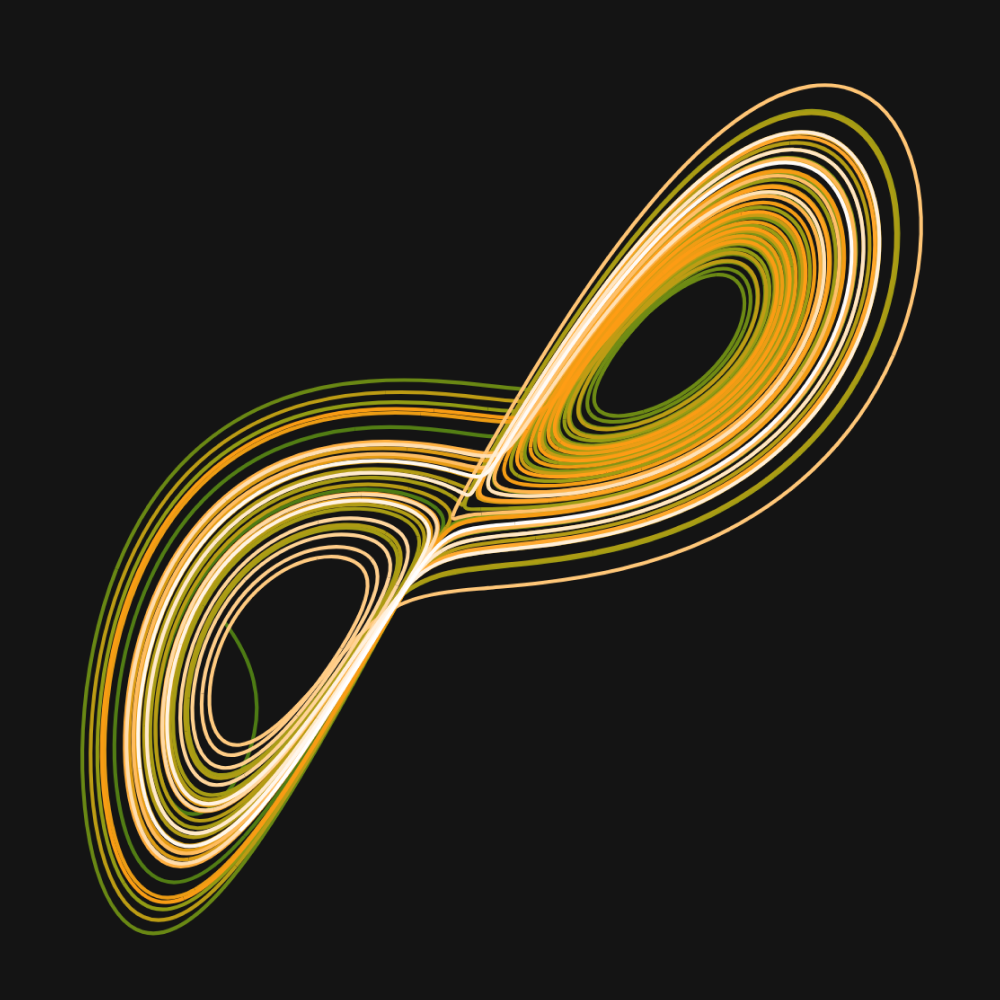
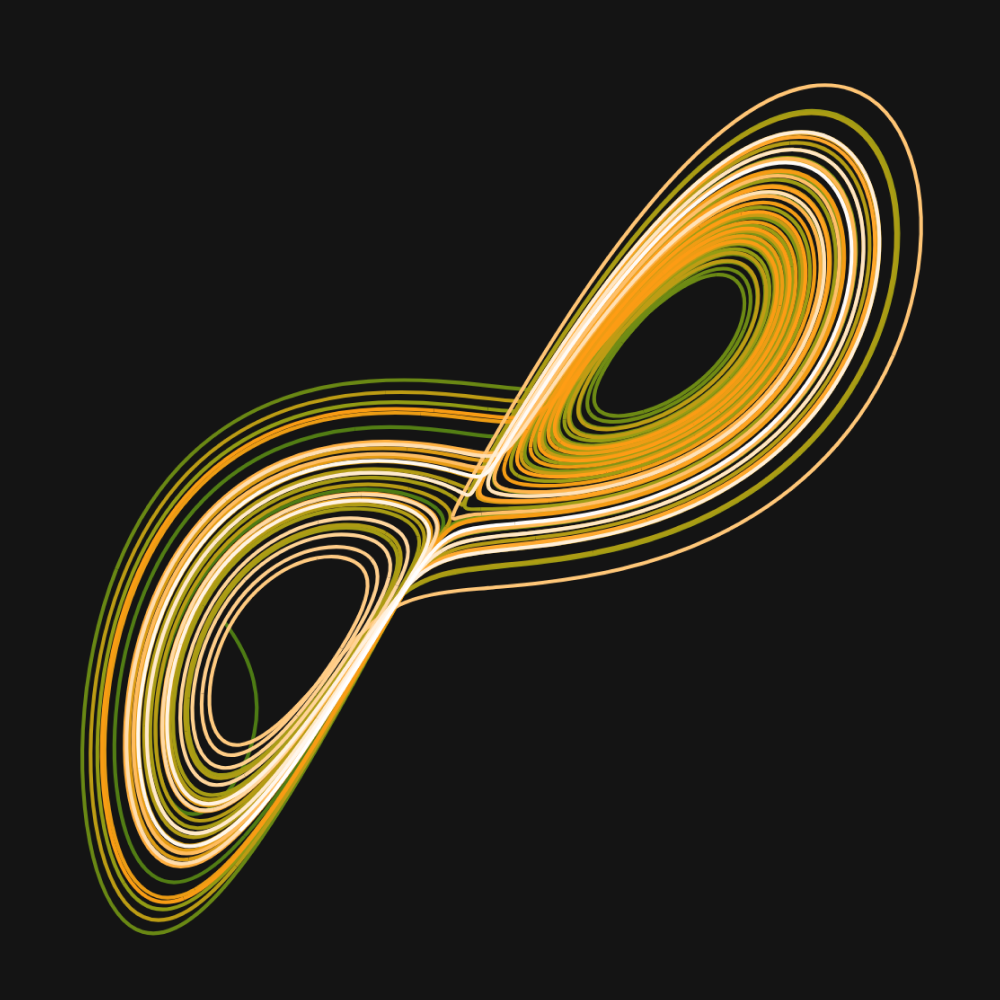
We discuss the properties of the dynamics of purely memristive circuits using a recently derived consistent equation for the internal memory variables of the involved memristors. In particular, we show that the number of independent memory states in a memristive circuit is constrained by the circuit conservation laws, and that the dynamics preserves these symmetries by means of a projection on the physical subspace. Moreover, we discuss other symmetries of the dynamics under various transformations of the internal memory, and study the linearized and strongly non-linear regimes of the dynamics. In the strongly non-linear regime, we derive a conservation law for the internal memory variables. We also provide a condition on the reality of the eigenvalues of Lyapunov matrices describing the linearized dynamics close to a fixed point. We show that the eigenvalues ca be imaginary only for mixtures of passive and active components. Our last result concerns the weak non-linear regime. We show that the internal memory dynamics can be interpreted as a constrained gradient descent, and provide the functional being minimized. This latter result provides another direct connection between memristors and learning.