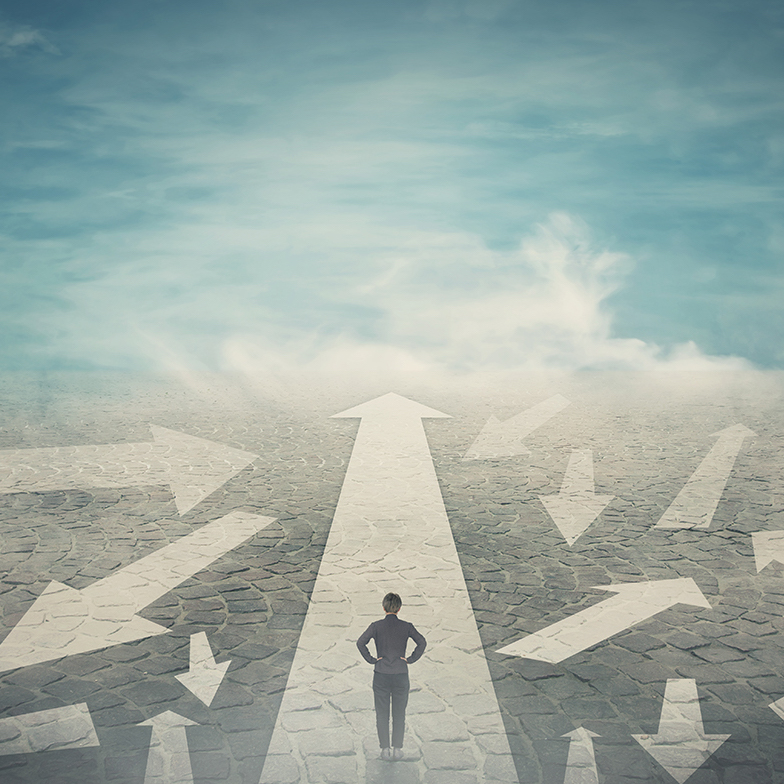
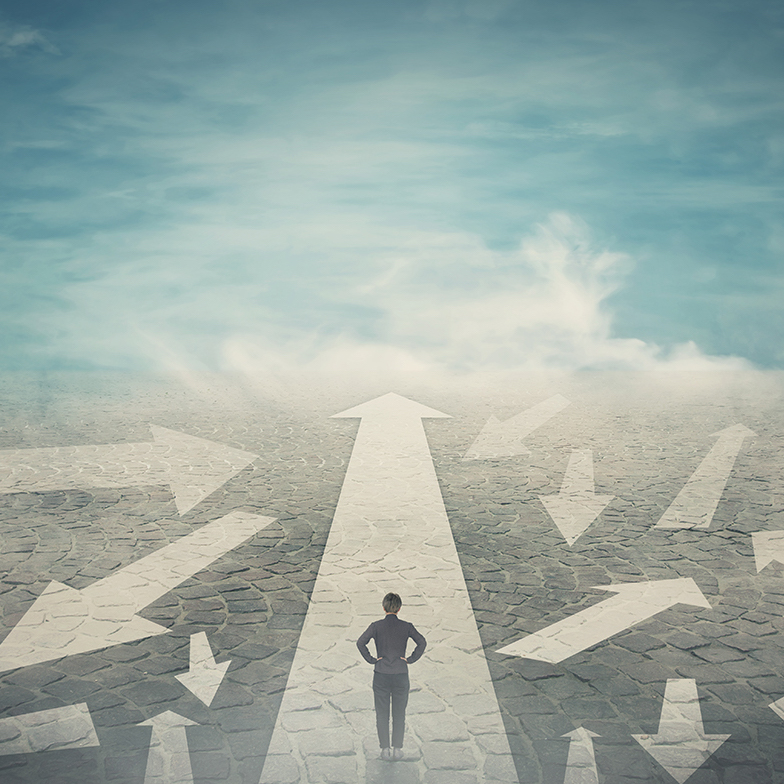
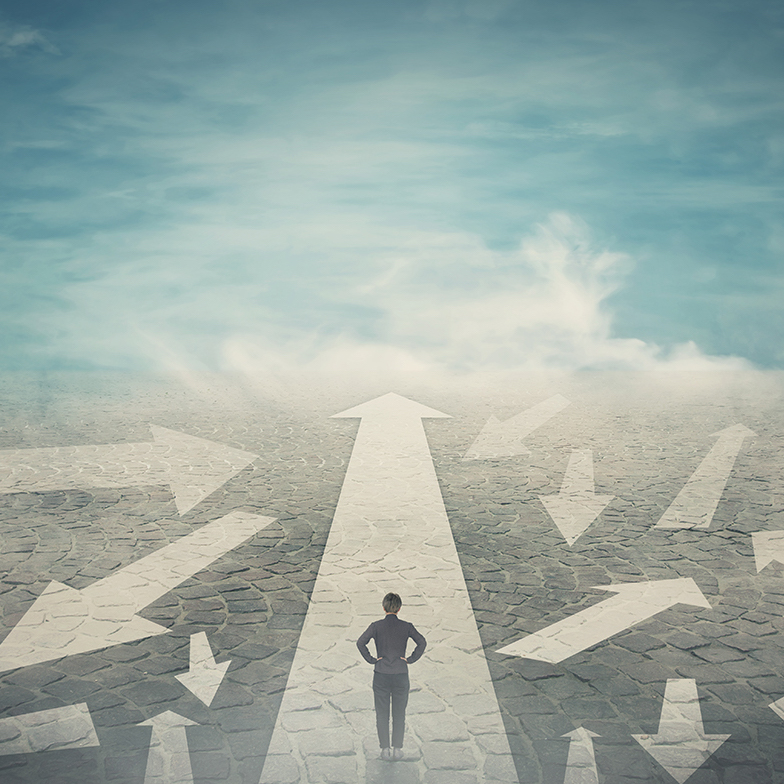
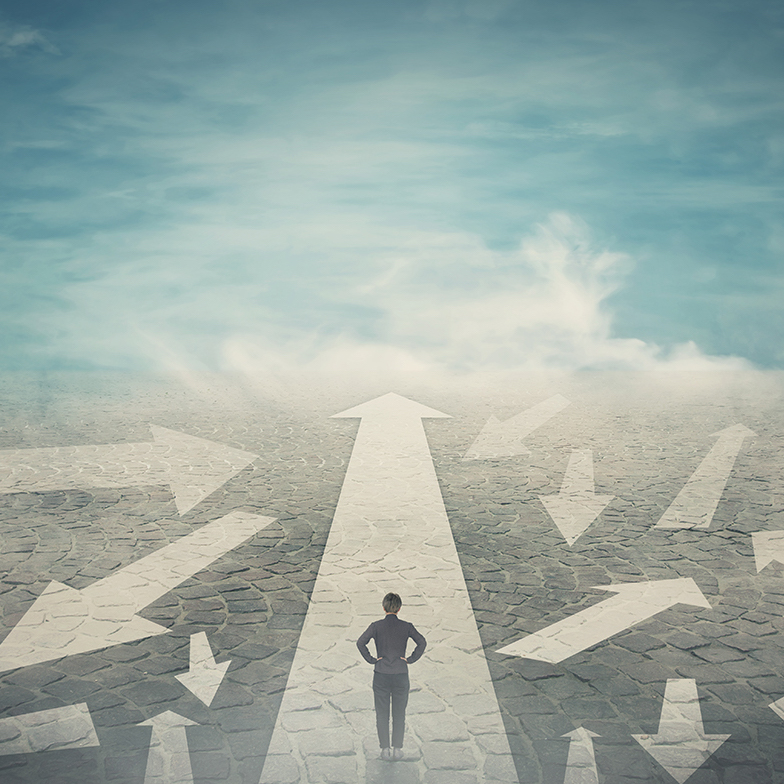
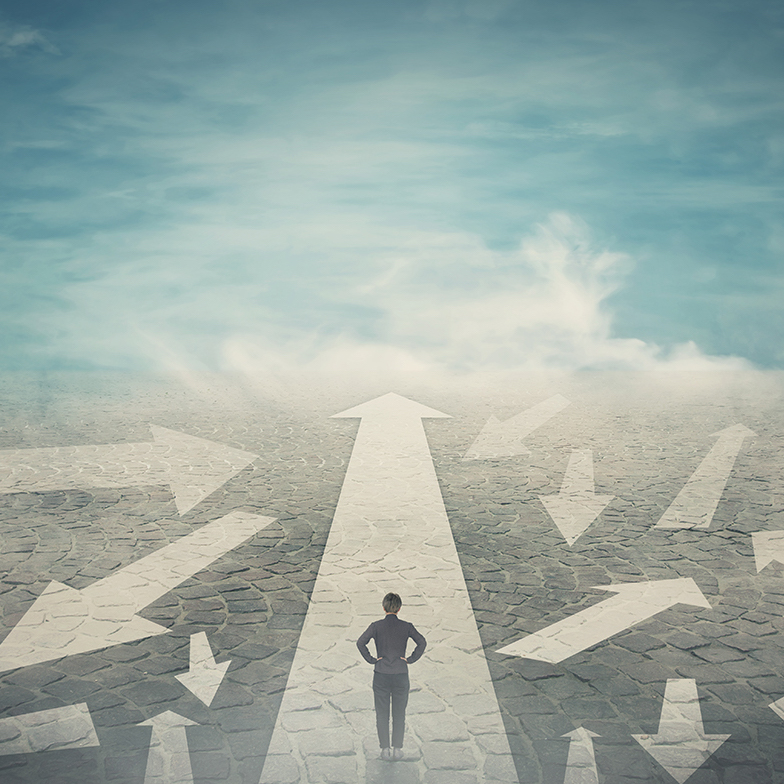
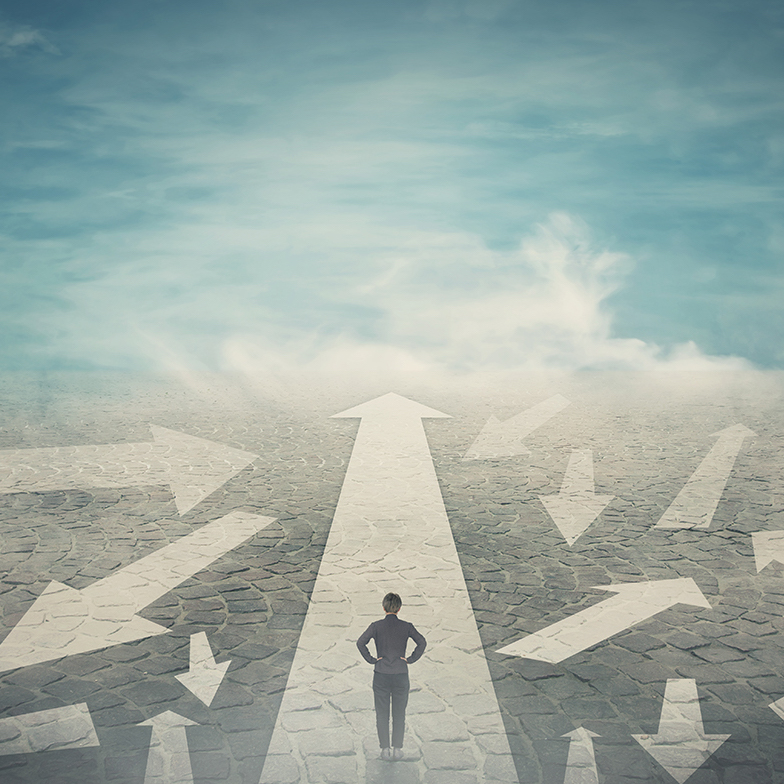
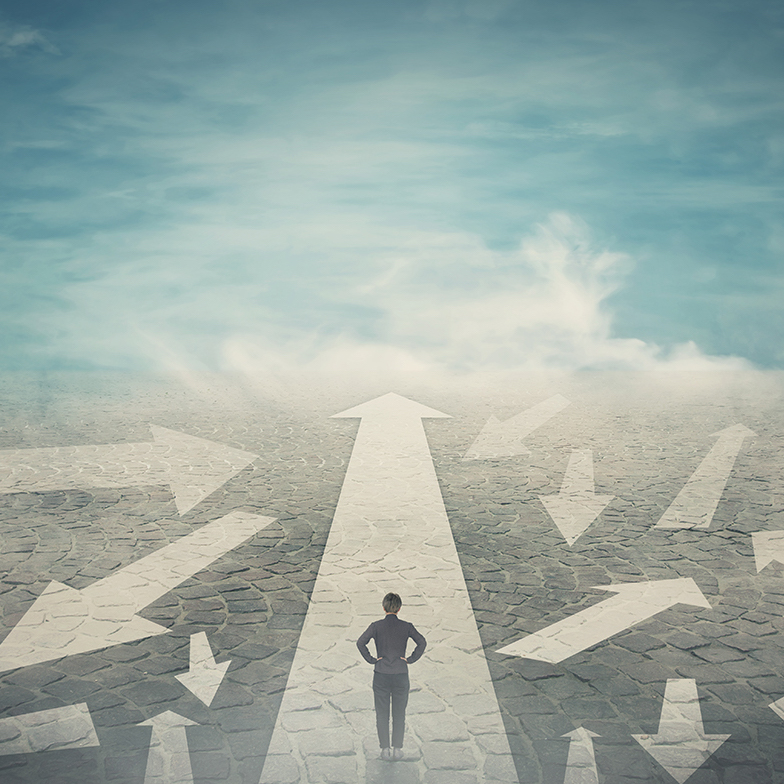
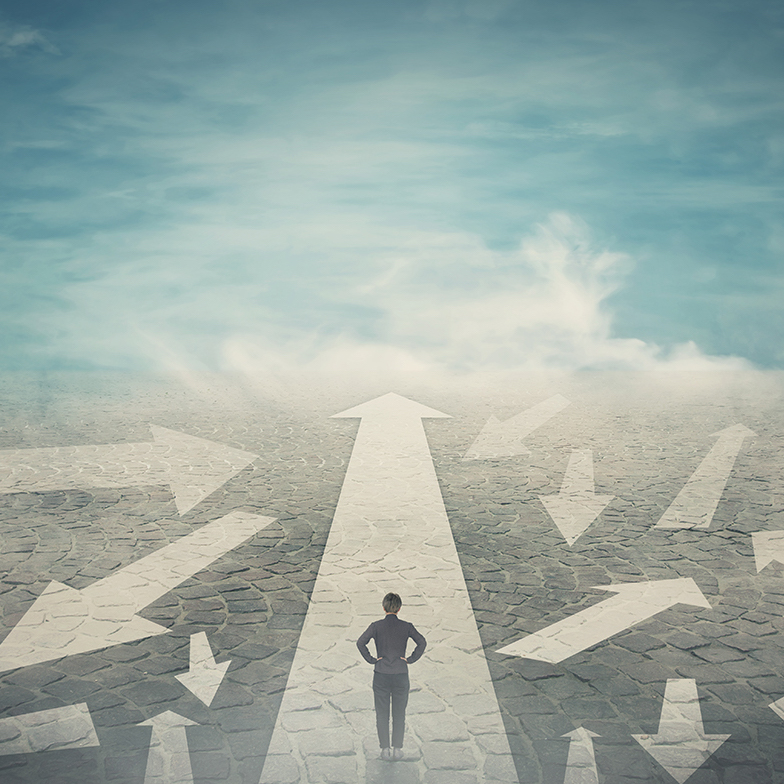
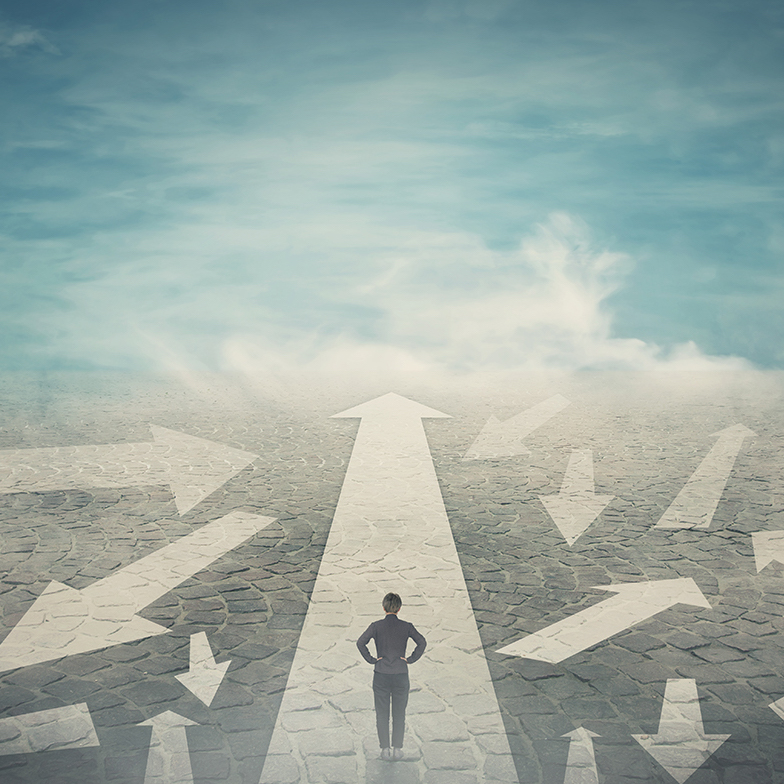
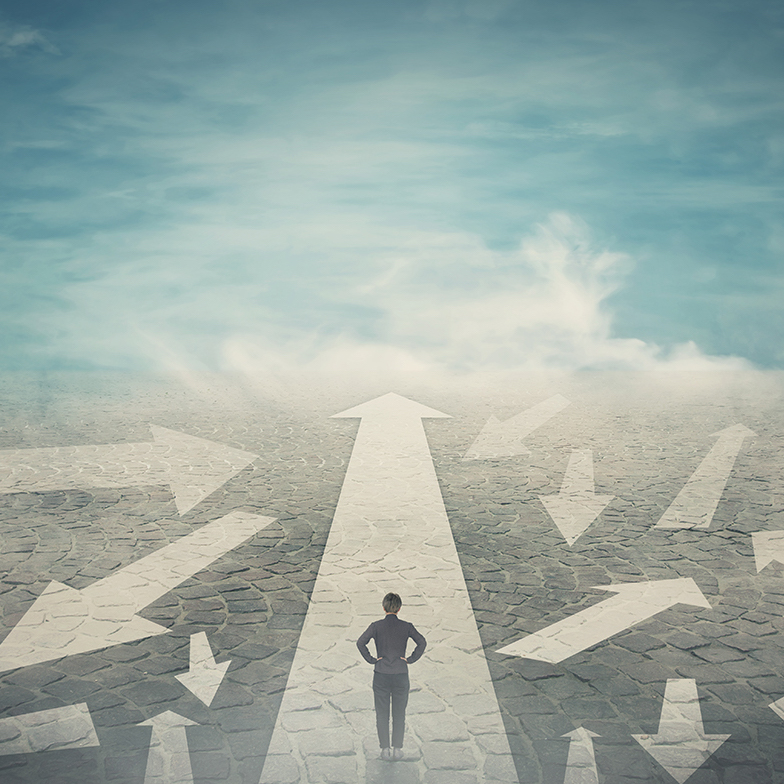
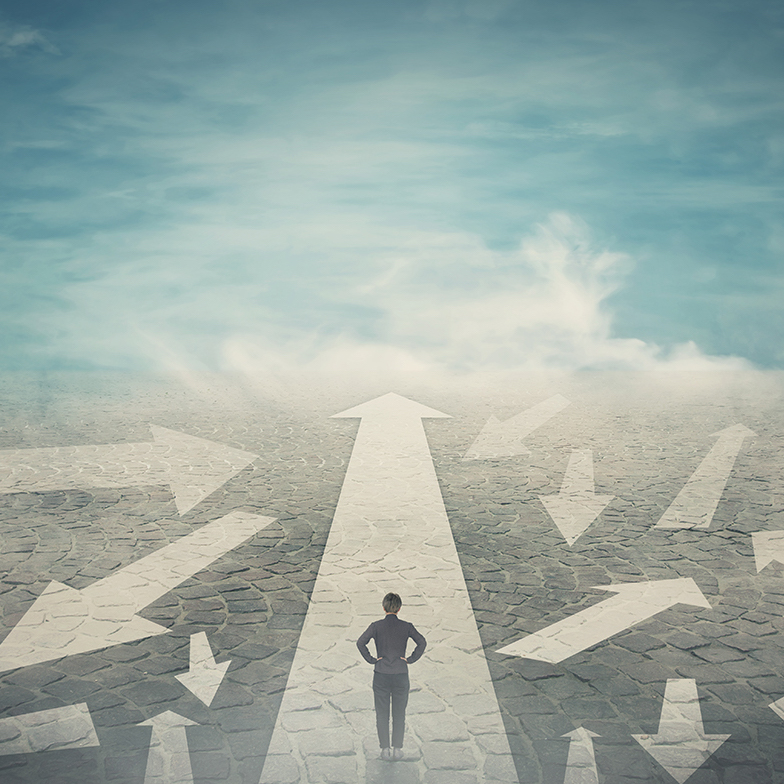
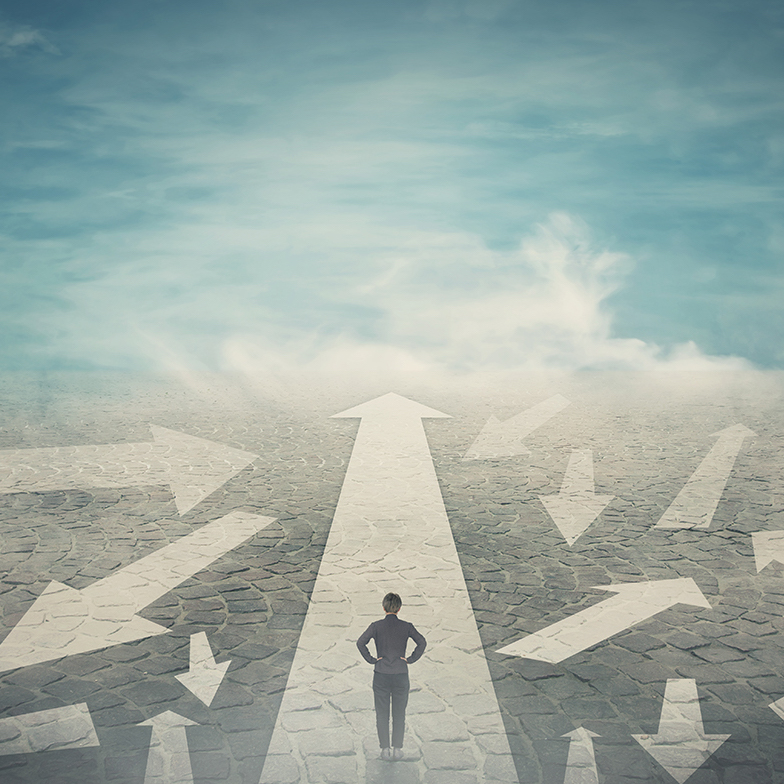
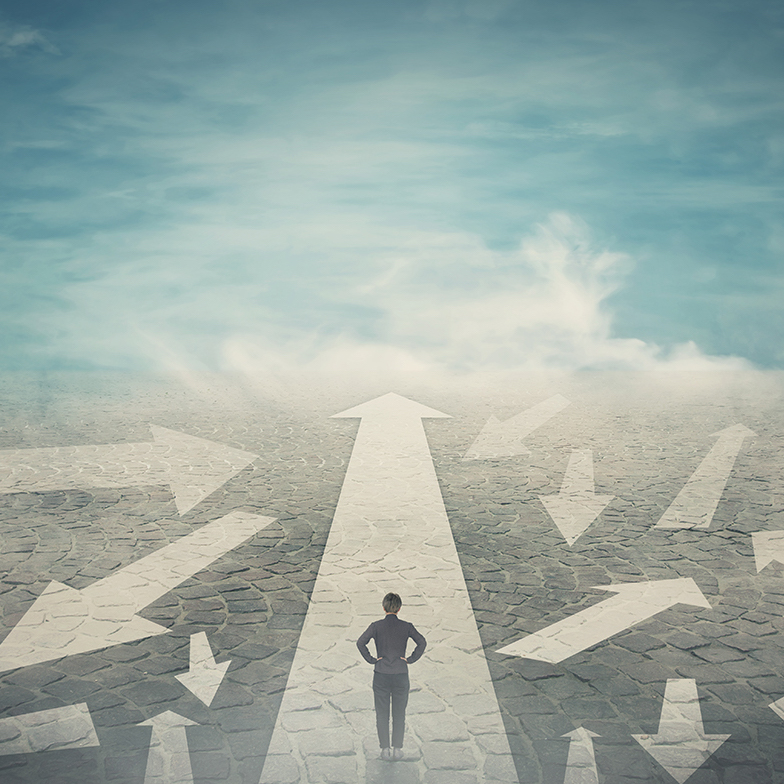
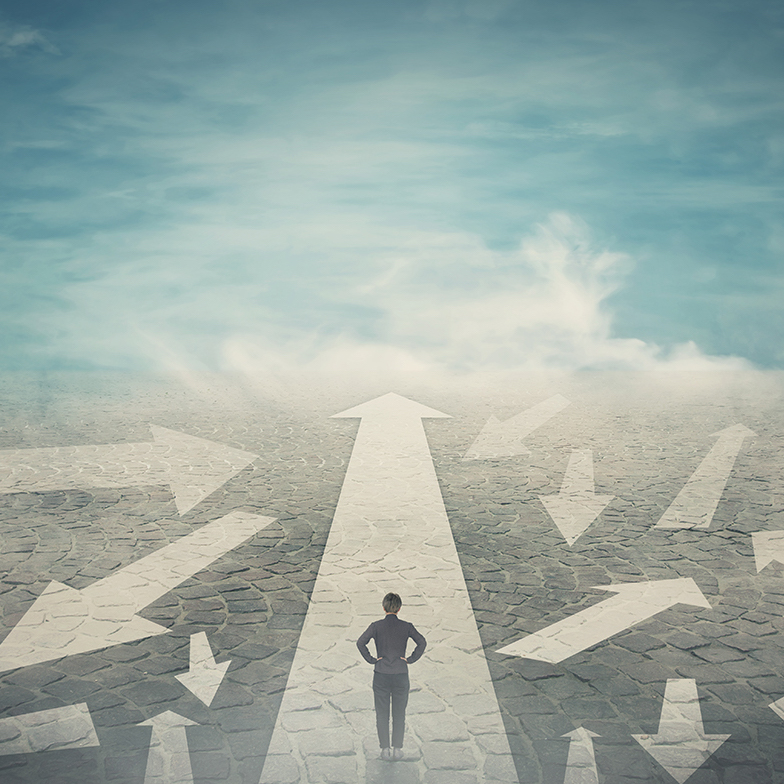
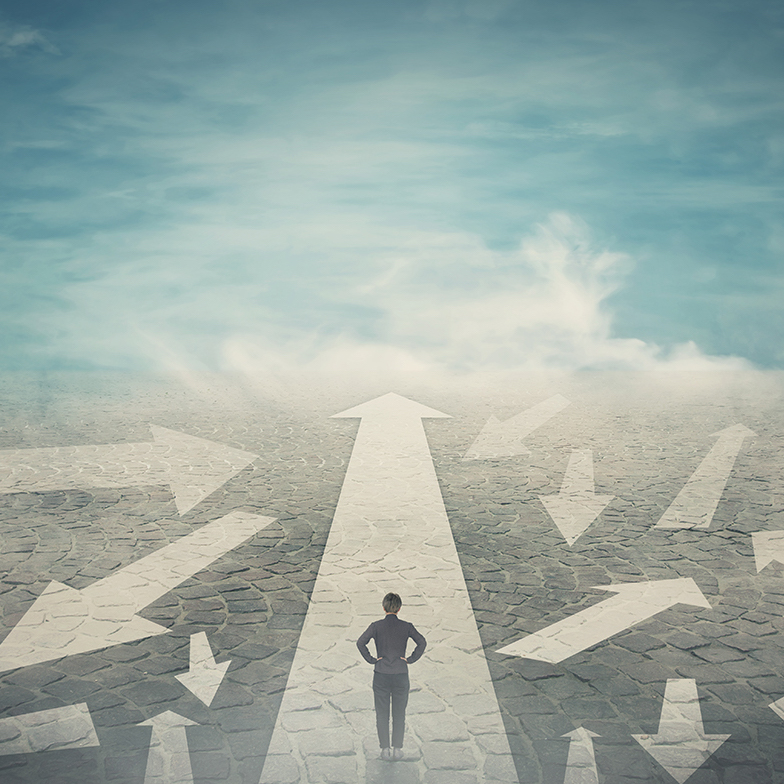
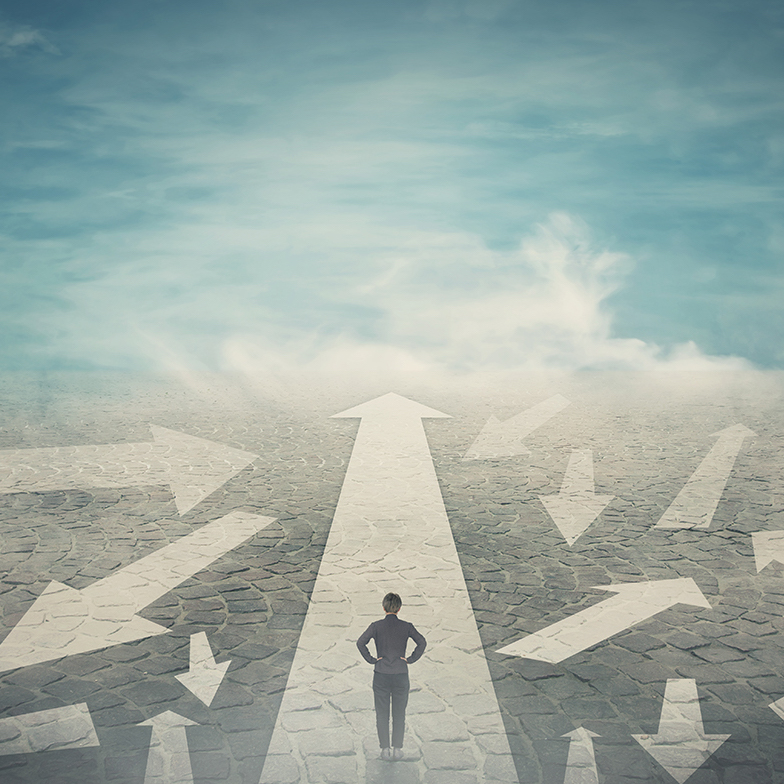
Guided by uncertainty
Machine learning
A new two-stage method addresses challenges in the natural language processing of long texts using transformers with self-attention mechanisms.
Uncertainty Guided Global Memory Improves Multi-Hop Question Answering
Meeting of the Association for Computational Linguistics (ACL), in press (2023)
Uncertainty Guided Global Memory Improves Multi-Hop Question Answering Transformers have become the gold standard for many natural language processing tasks, however, models with self-attention mechanisms struggle to process long sequences due to their quadratic complexity. Therefore, processing long texts remains a challenge. To address this issue, we propose a two-stage method that first collects relevant information over the entire document and then combines it with local context to solve the task. Our experimental results show that fine-tuning a pre-trained model with memory-augmented input, including the least uncertain global elements, improves the model's performance on multi-hop question answering task compared to the baseline. We also found that the content of the global memory correlates with the supporting facts required for the correct answer.
Meeting of the Association for Computational Linguistics (ACL), in press (2023)