Advancing finance with synthetic time series generation
9 AM, 24 Jan 2024
This workshop will focus on the latest advances in synthetic time series generation and the promise they hold for financial modelling.
The application of data-greedy machine-learning algorithms relies on access to large datasets. In the financial sector, data is often limited owing to privacy constraints and the complexity of financial time series. Models for synthetic time series generation have arisen as a promising approach to mitigate the issue of data availability while respecting privacy considerations. Moreover, these models may provide novel solutions for risk management and deal with imbalance problems in credit default prediction.
This workshop, which focuses on the latest advances in high-fidelity generative models for financial time series, will bring together academics and industry practitioners. It will feature presentations on state-of-the-art generative models, which are designed to tackle practical challenges in financial time series. There will also be a hands-on tutorial on how to use the python pipeline to empirical financial data. Finally, a panel discussion will focus on applications where synthetic time series is already starting to transform the finance sector and the future implications of generative AI.
Registration
Event information
The event takes place on Wednesday 24 January at the London Institute for Mathematical Sciences, which is on the second floor of the Royal Institution. Please come at 09:00 for coffee beforehand. We will move to Tyndall’s Parlour for the talks, tutorial and panel discussion, which will start at 09:30 and last until 13.30 or thereabouts.
Funding acknowledgement
The workshop is supported by the EPSRC under the program grant EP/S026347/1 (DataSig) and the Ecosystem Leadership Award under the EPSRC Grant OobfJ22100020 and The Alan Turing Institute.
Programme
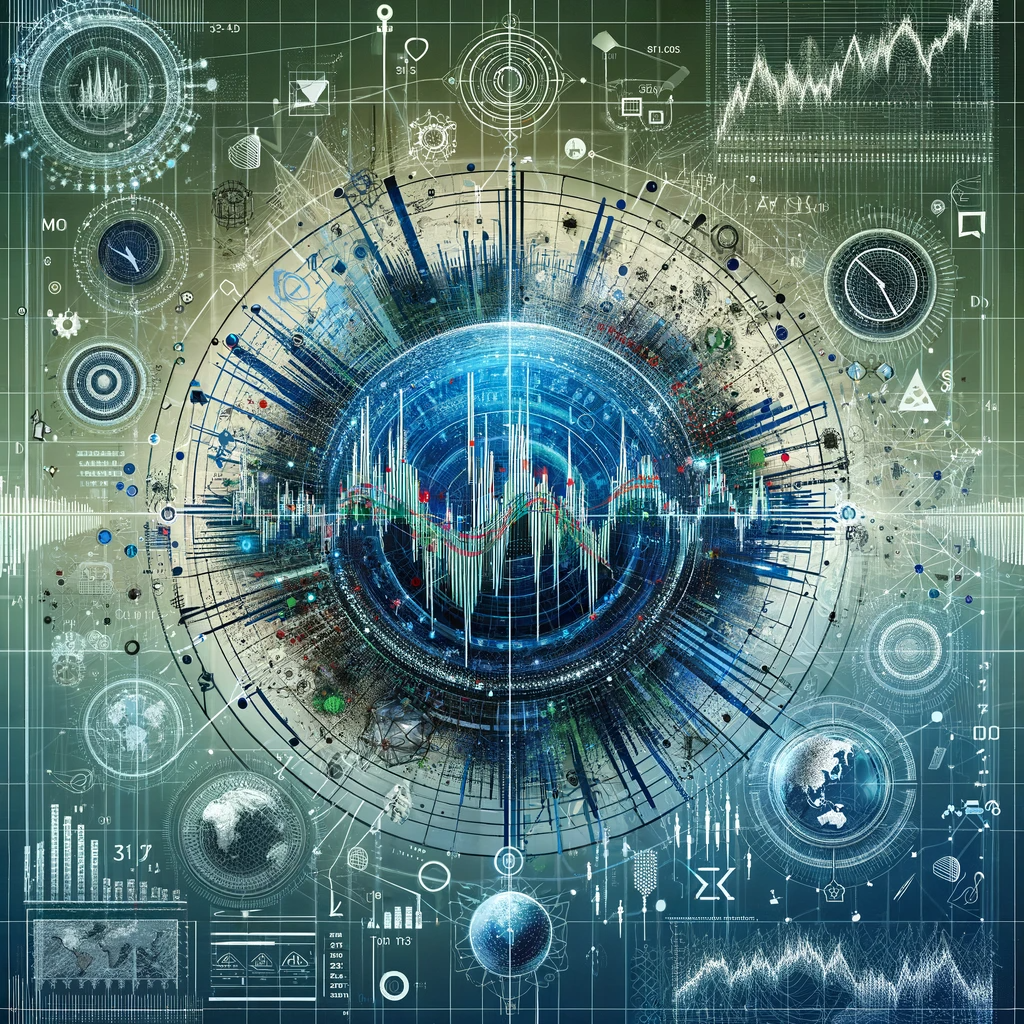
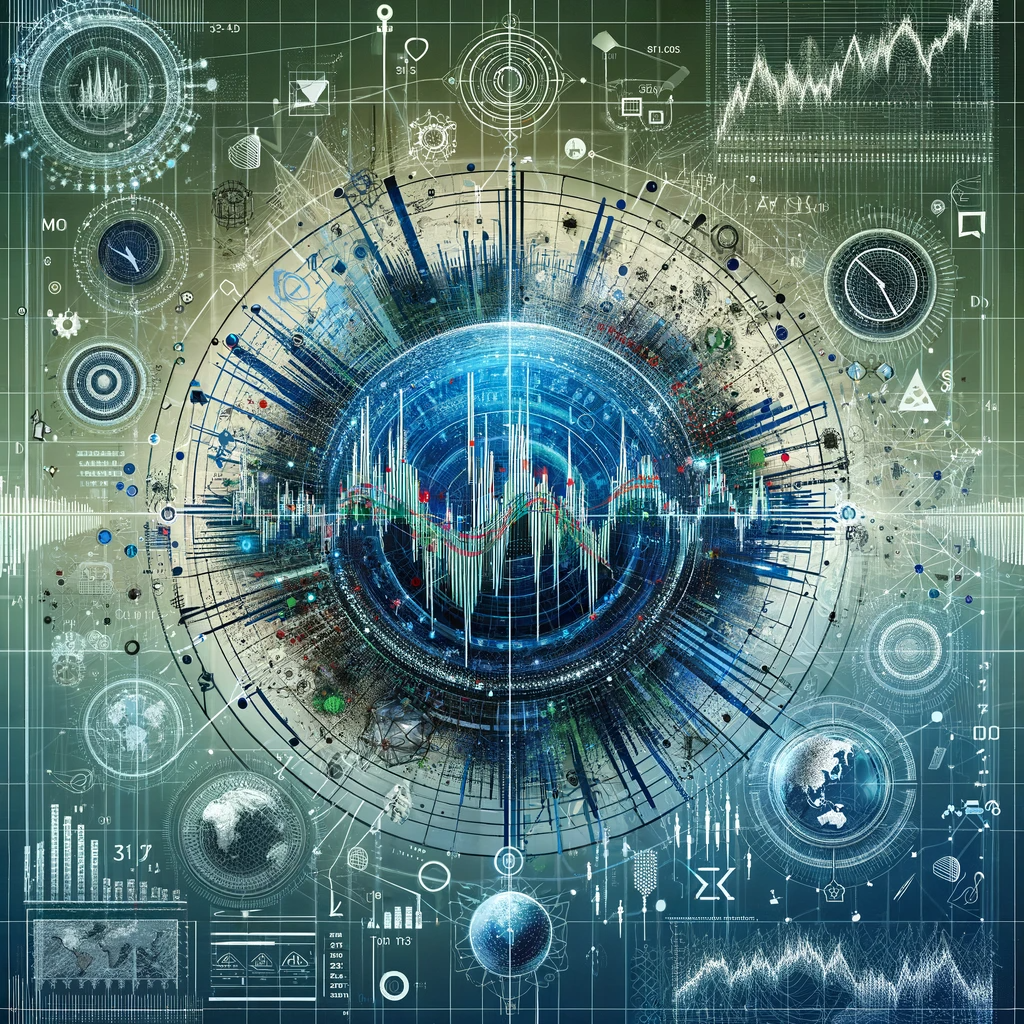
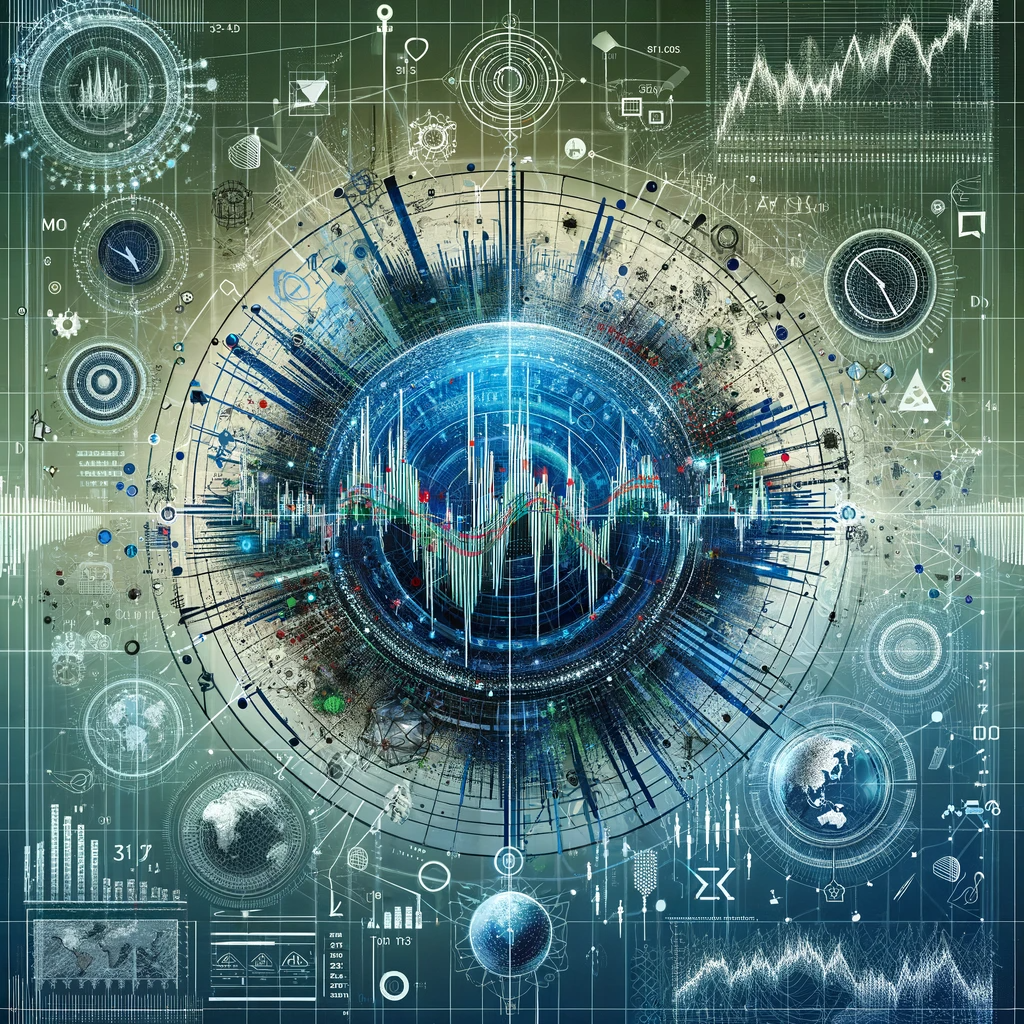
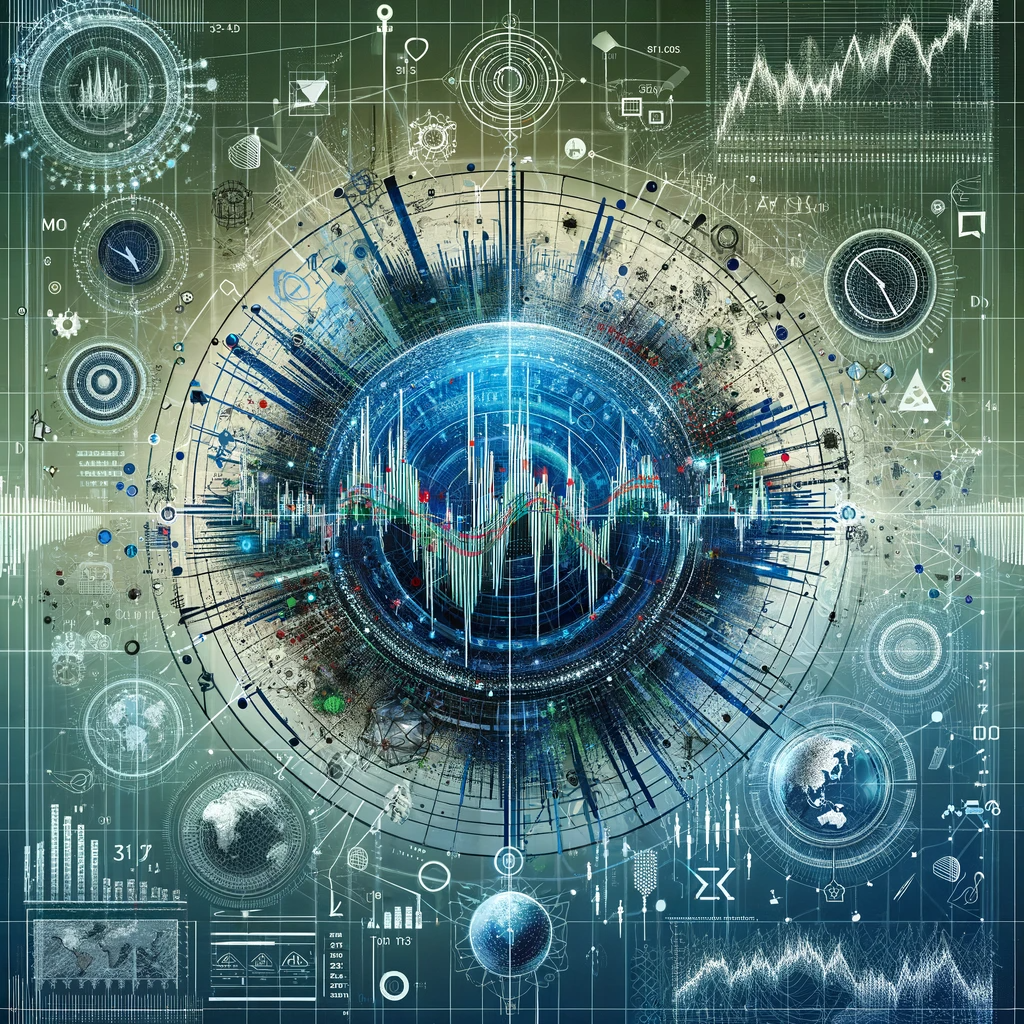
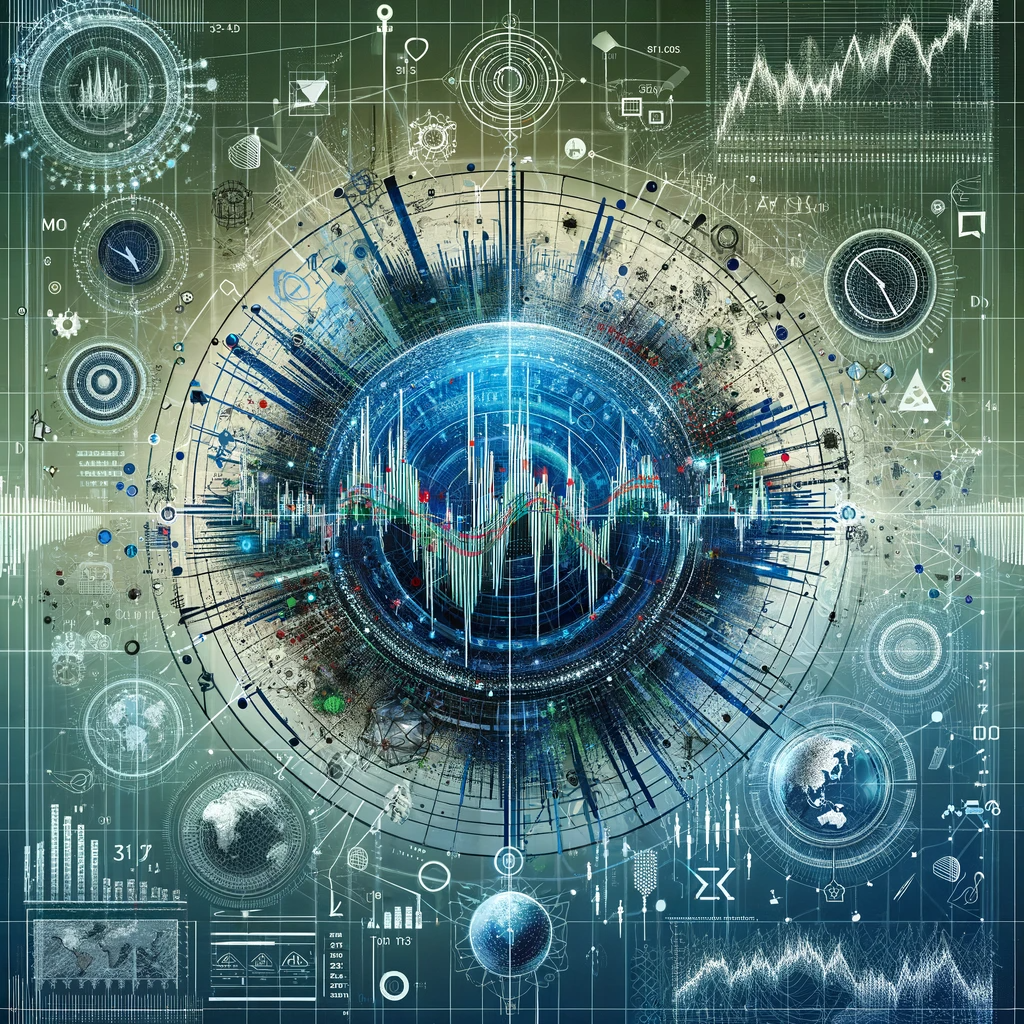
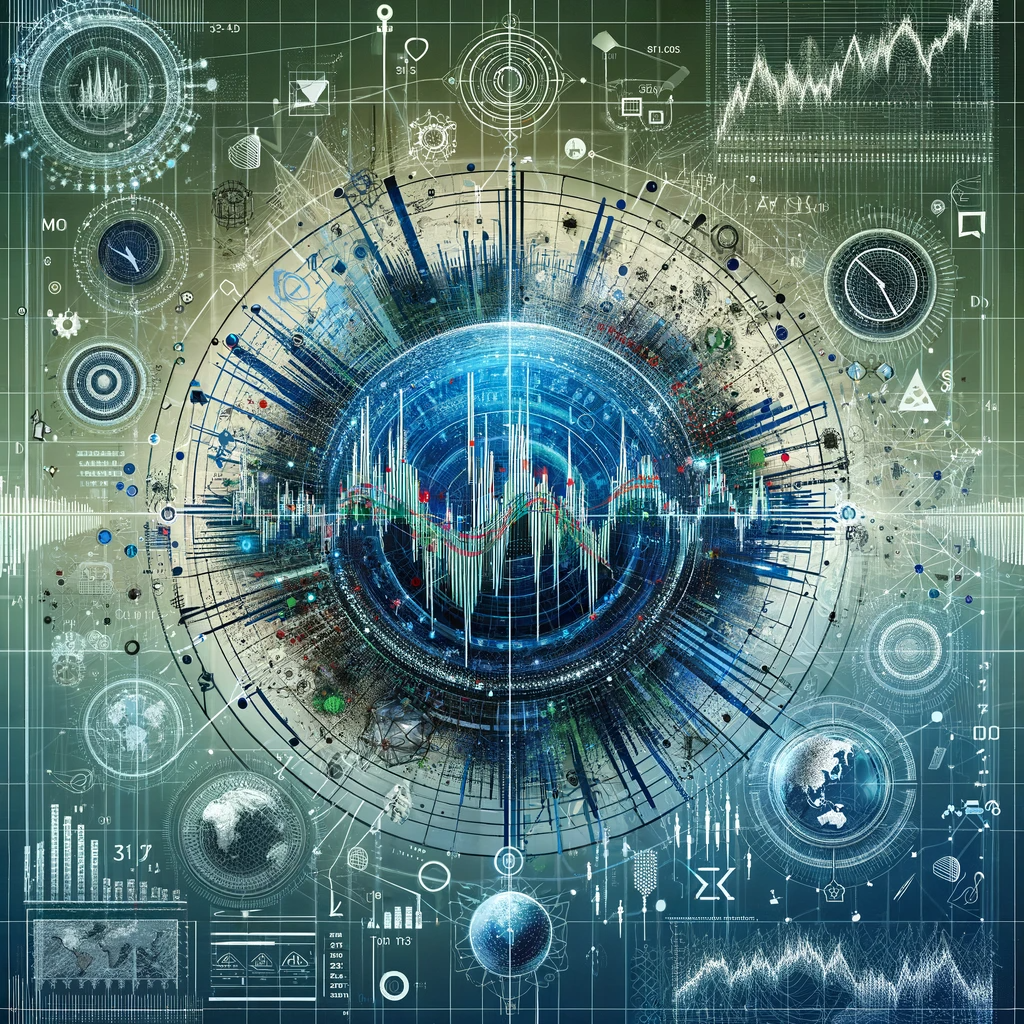
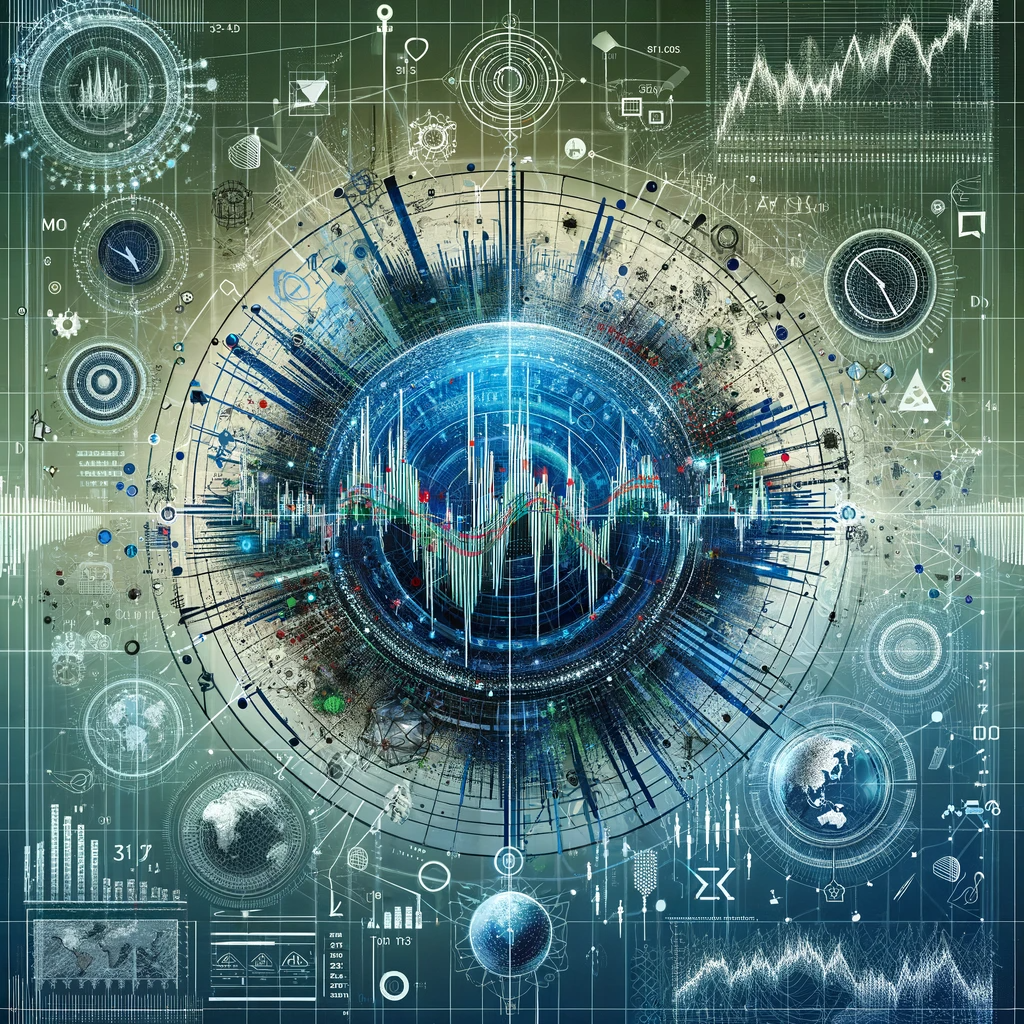
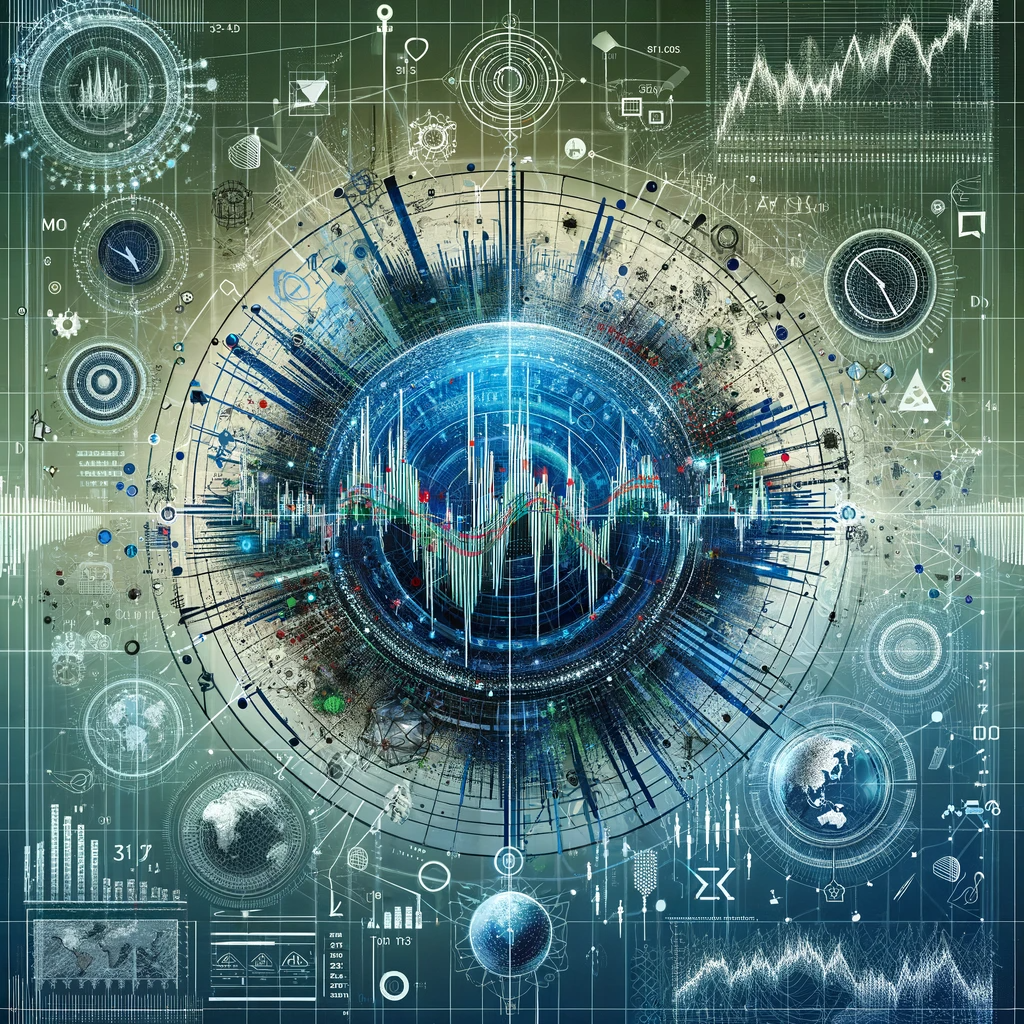
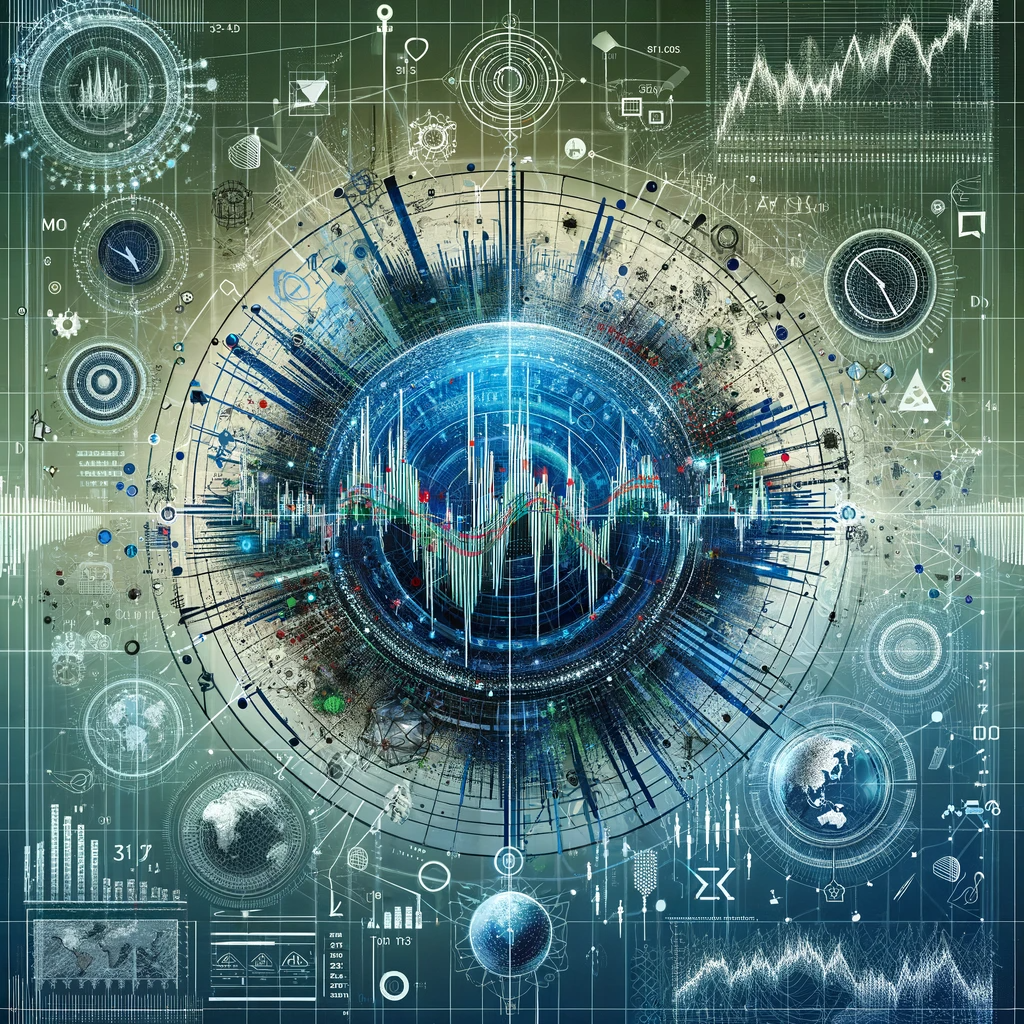
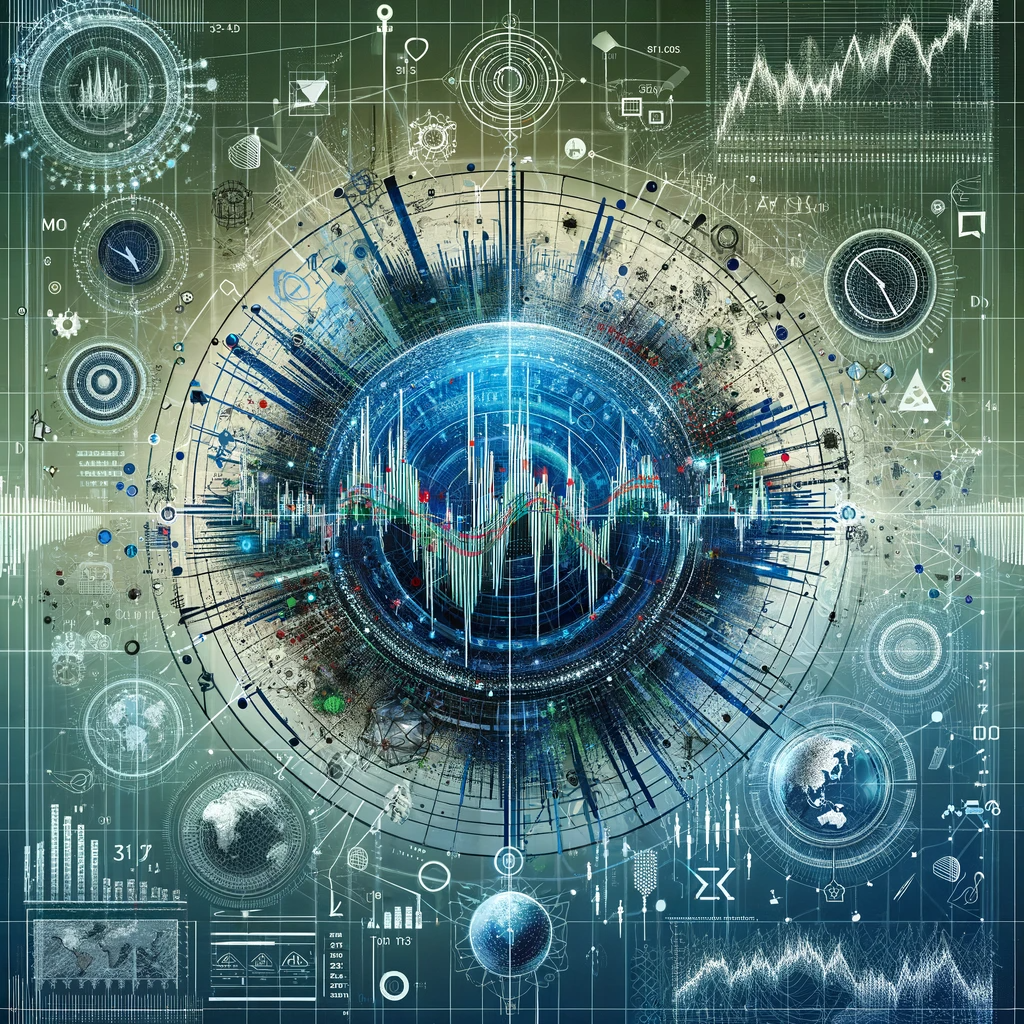
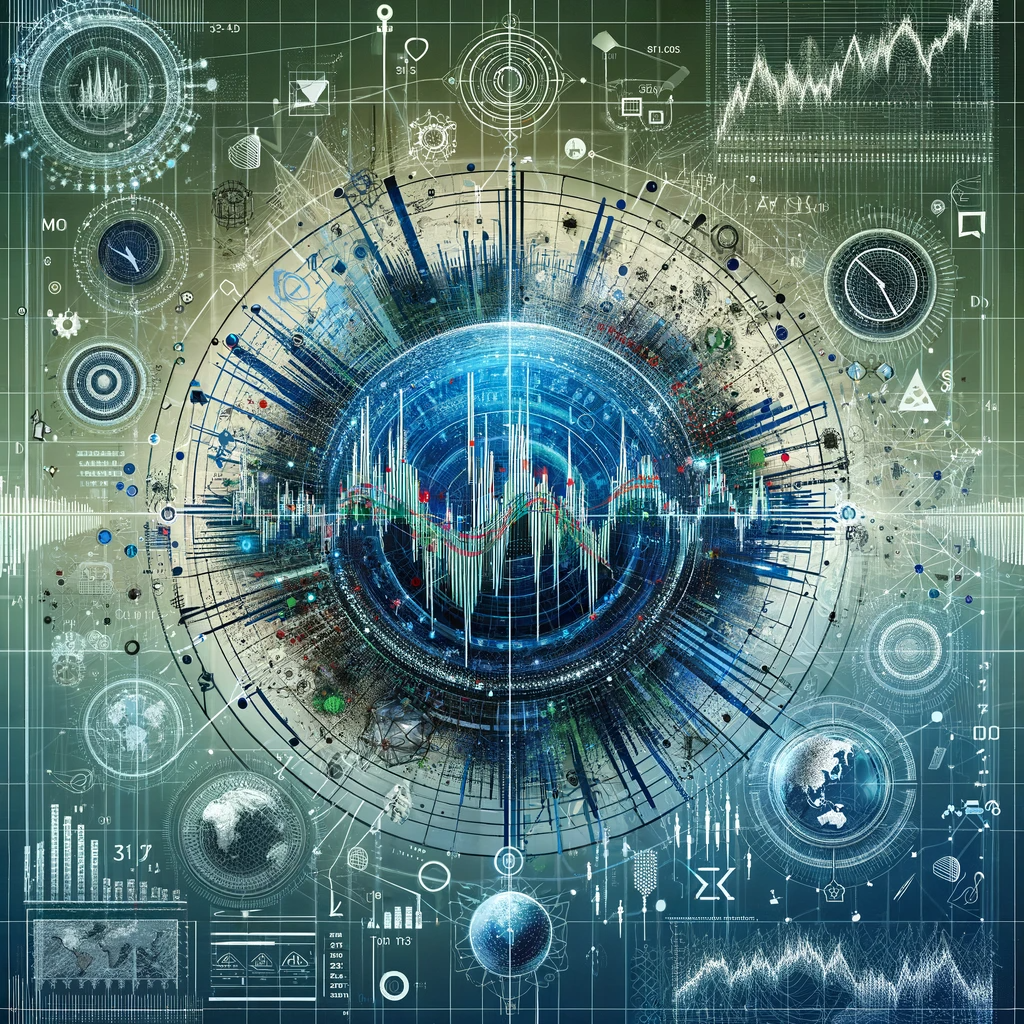
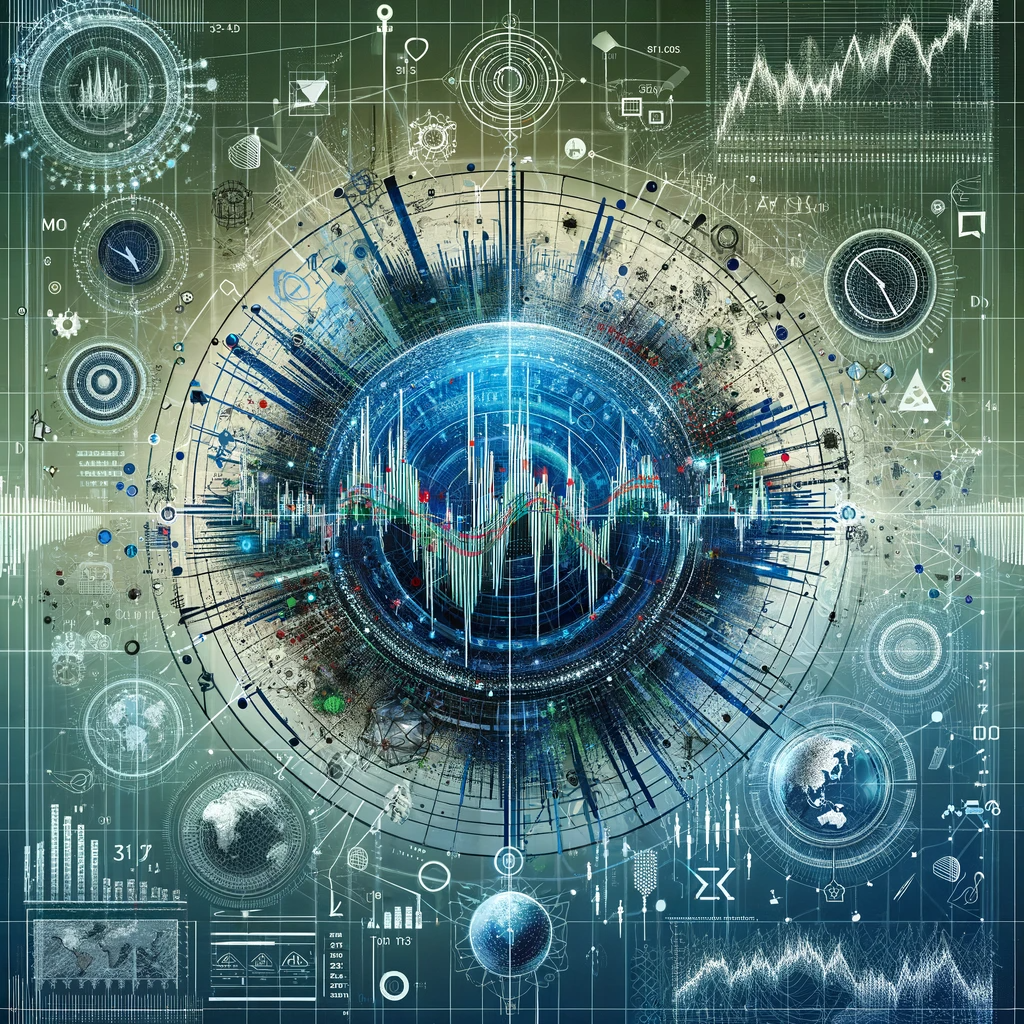
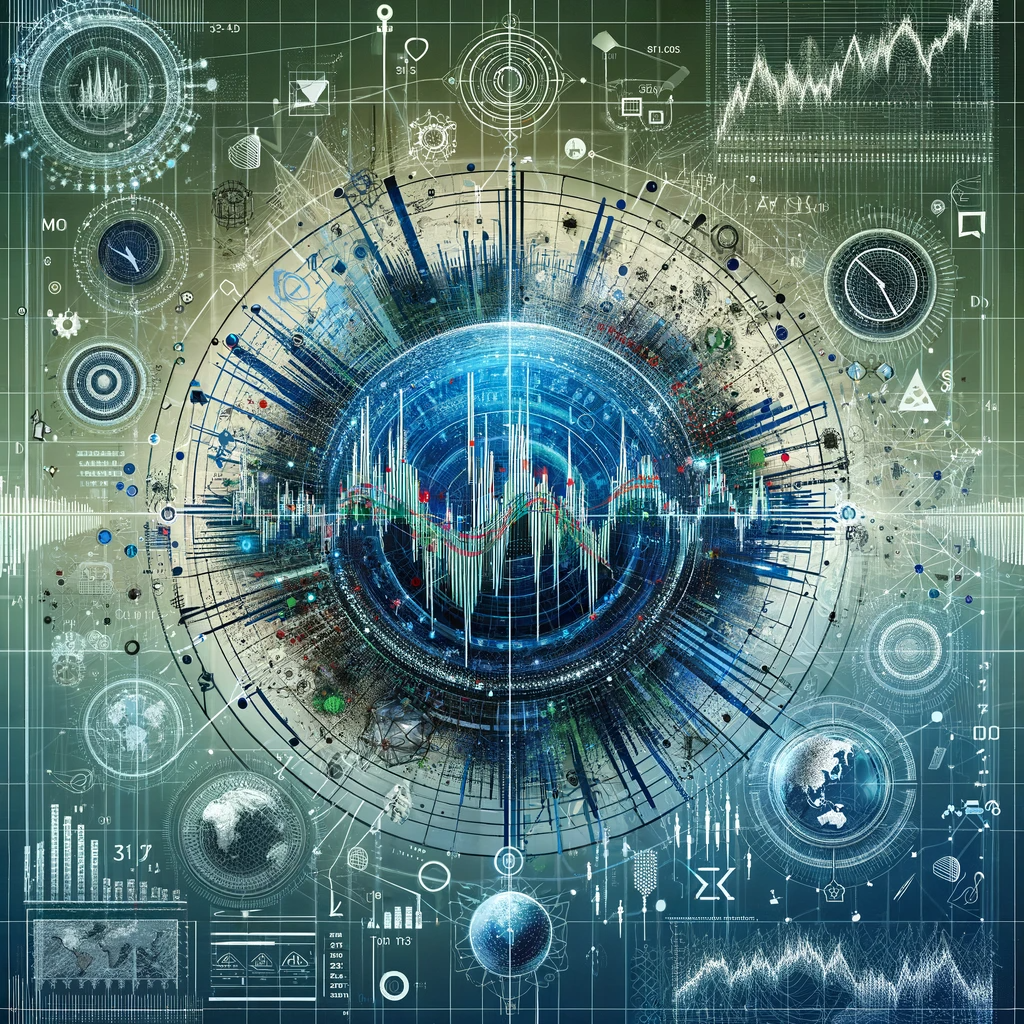
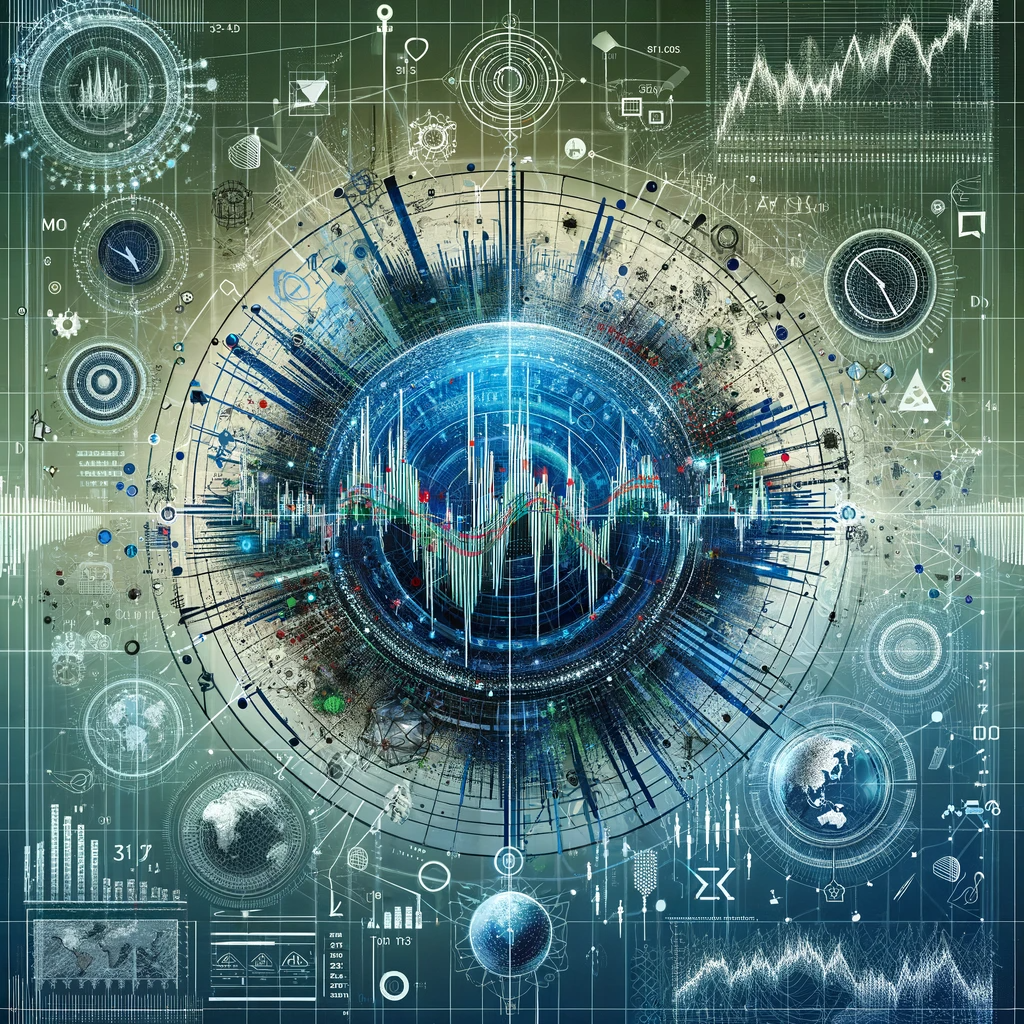
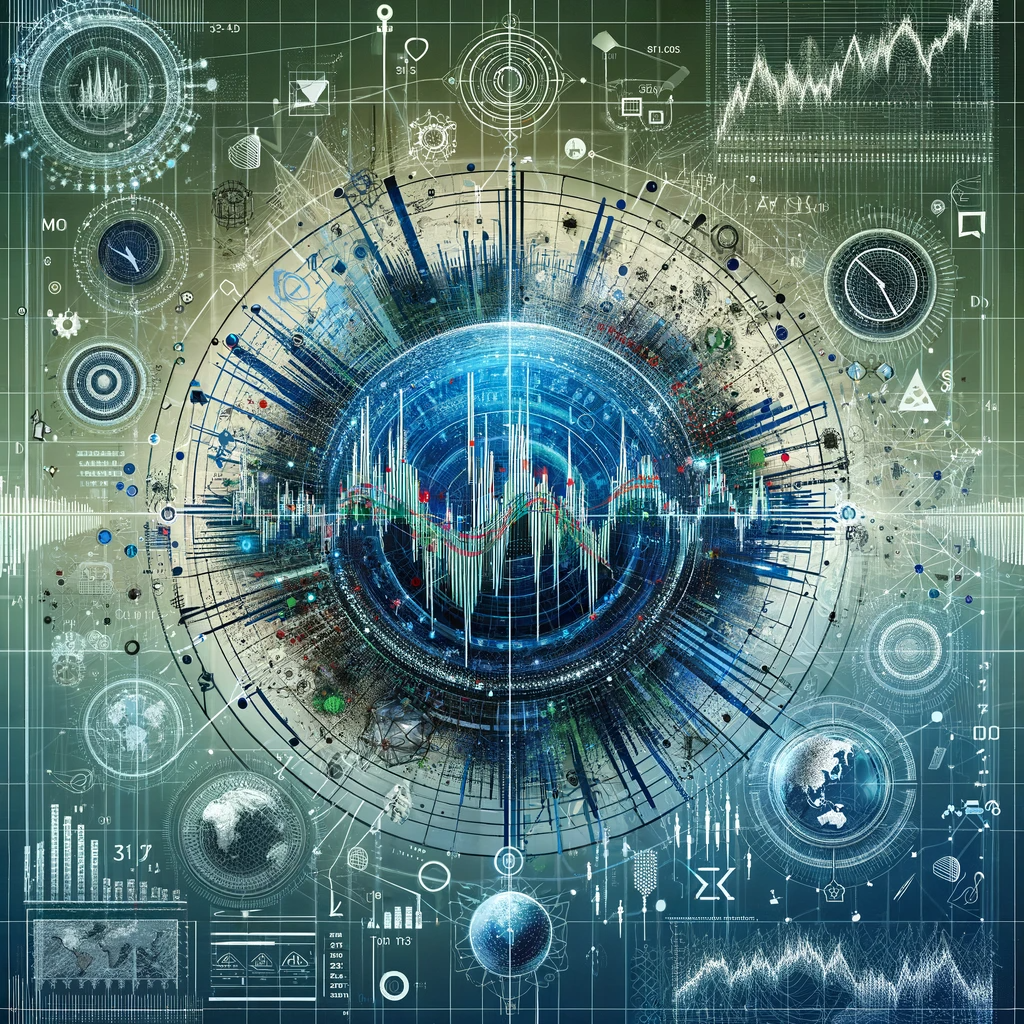
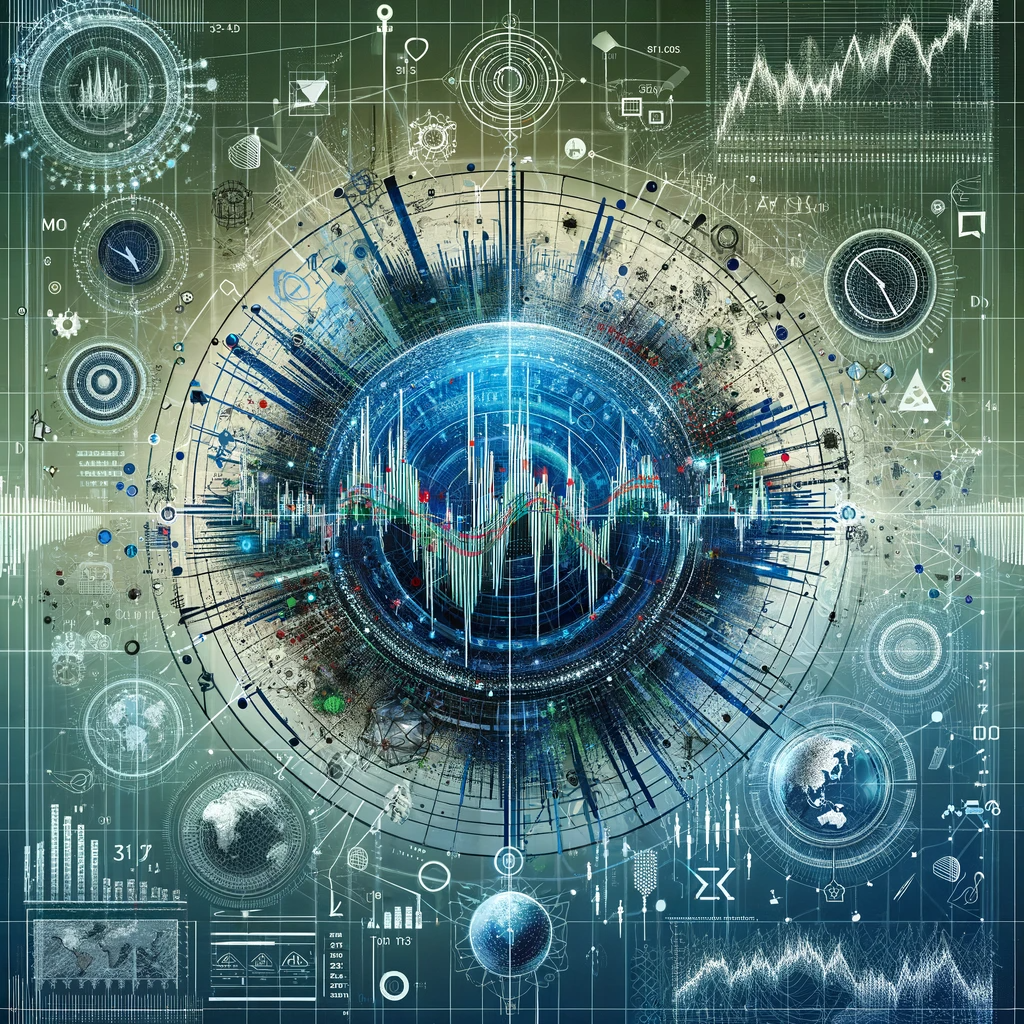
09:00
Arrivals
09:30
Opening remark
09:35
Tail-GAN: Learning to Simulate Tail Risk Scenarios
Simulation of scenarios is needed to estimate the loss distributions for dynamic portfolios; we use GANs and exploit the joint elicitability property of VaR and ES. We prove a universal approximation theorem and show that the training of GANs may be formulated as a max-min game.
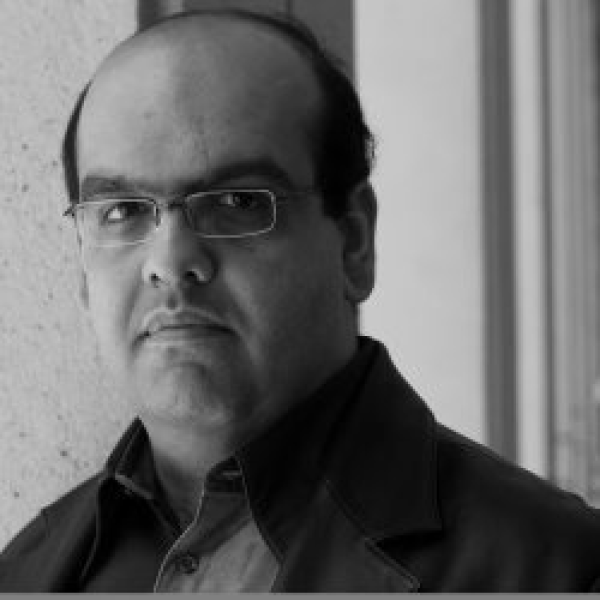
Rama Cont
Prof. Rama Cont is a Professor of Mathematics at Oxford, where he holds the Chair of Mathematical Finance. He has served as advisor to the IMF. His work focuses on stochastic processes and mathematical modeling in finance, with a focus on market instabilities and systemic risk.
10:00
Practical Considerations on Synthetic Data Generation in Quantitative Finance
Choosing and training (generative) models are only parts of an end-to-end machine learning pipeline. Other relevant considerations include deployment at scale, cost and modelling improvements through ensembling techniques. We explore tentative answers to some of these points.
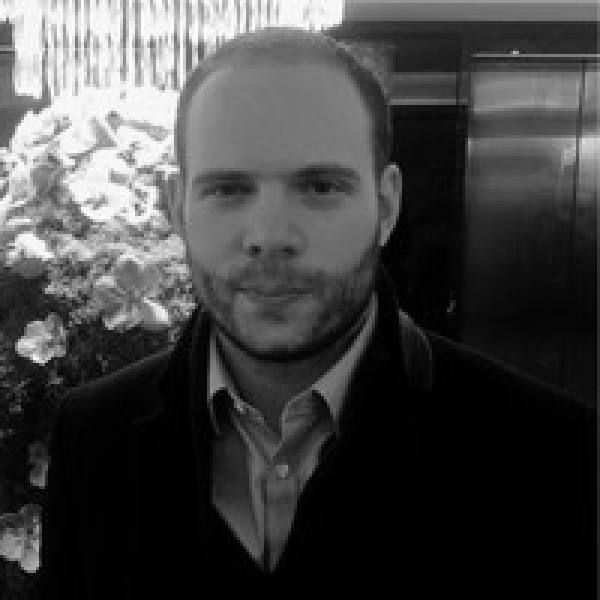
Francois Buet-Golfouse
Dr Francois Buet-Golfouse is Head of Decision Science at JP Morgan Chase & Co. He did his PhD in mathematics at UCL. He leads a team of quantitative researchers and machine learning scientists, working on natural language processing, reinforcement learning, recommender systems and AI ethics.
10:25
Coffee break
10:45
Synthetic Data Generation from Stochastic Differential Equation Models Calibrated with Signature Wasserstein 1 Metric
In the ICAIF Regime-Switching Financial Time Series Generation competition, we use a parameter estimation framework for SDE-based synthetic data generation, leveraging the Signature Wasserstein-1 metric. Implementation and model sensitivity to parameter tuning are also discussed.
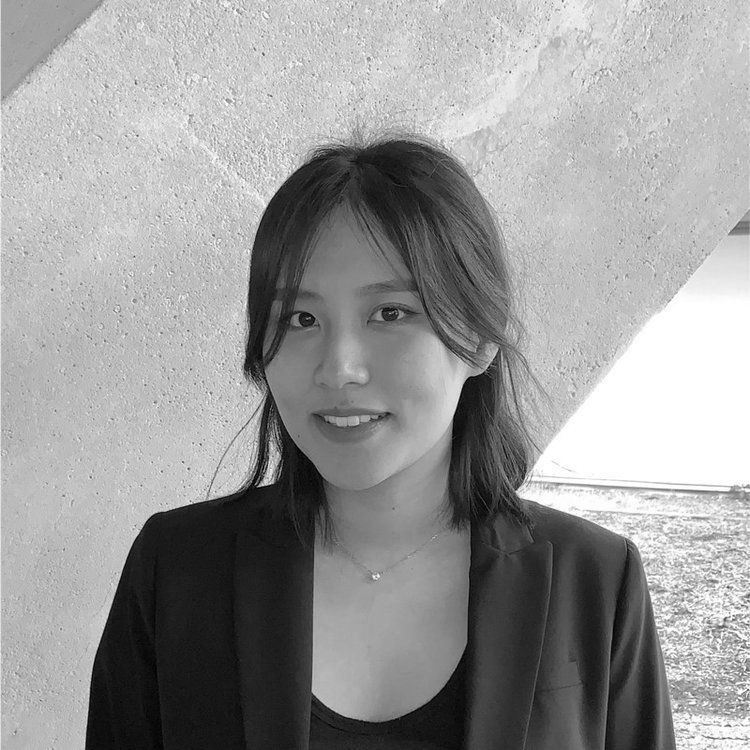
Michelle Ko
Michelle Ko is doing a master’s in statistics at the University of Waterloo. She has worked as a data scientist at Omnium, a consulting firm specialising in consumer packaged goods analytics. Her work focuses on particle methods for parameter inference of jump diffusion models.
11:15
Advanced Framework for Generating Financial Time Series Data
We present a pipeline tailored for financial time series generation encompassing preprocessing and training generative models. Model are evaluated with metrics that ensure accuracy & reliability in financial contexts. Finally, we showcase its application in real-world scenarios.
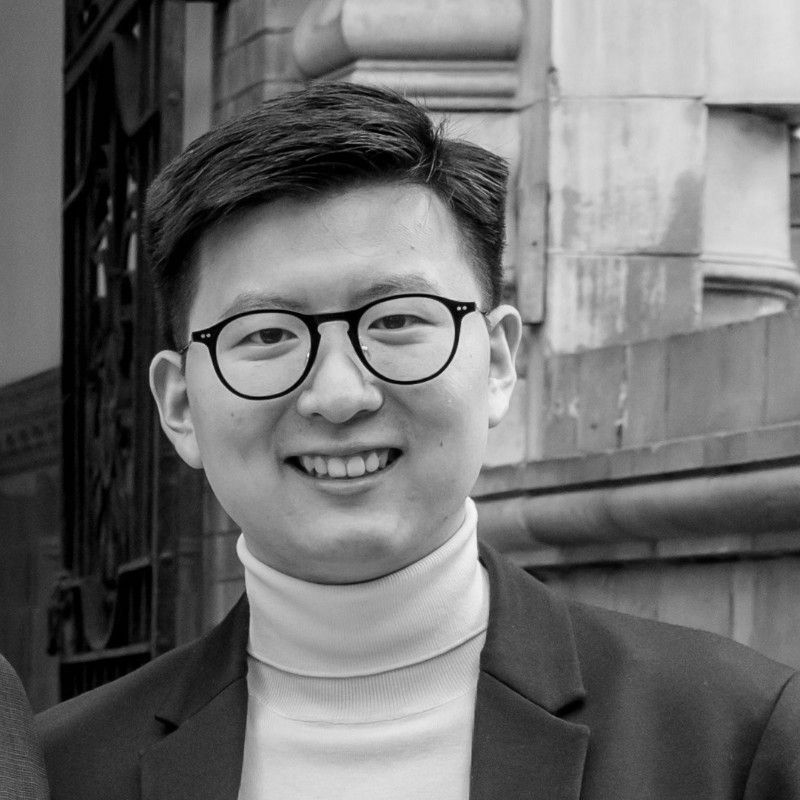
Jiajie Tao
Jiajie Tao is doing his PhD at UCL. He has a master’s in mathematics from Imperial College London. He works on rough path theory and machine learning, especially the use of mathematical models in real-world scenarios such as finance, medical science and human action recognition.
11:45
Coffee break
12:05
Panel discussion
Panel chair: Lingyi Yang (Oxford); Panellists: Terry Lyons (Oxford), Rama Cont (Oxford), Jian Chen (Point 72), Francois Buet-Golfouse (JPMorgan Case), Mikhail Burtsev (London Institute)
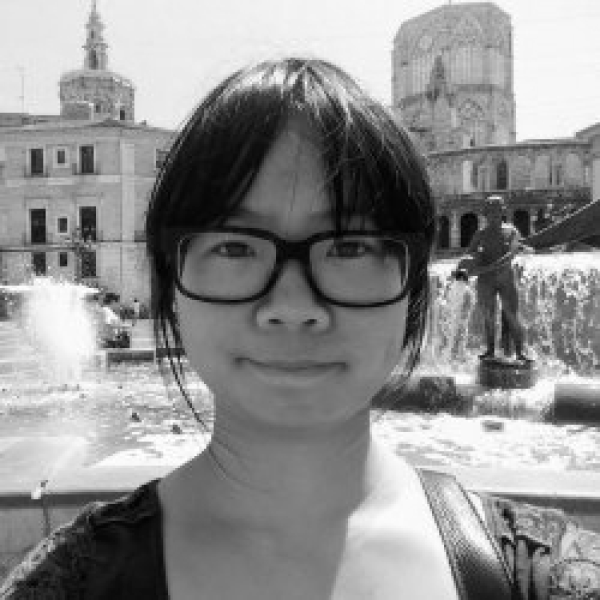
Lingyi Yang
Lingyi Yang is doing a postdoc at Oxford and the Alan Turing Institute. As well as anomaly detection in time series and differential-equation-inspired neural networks, she works on economic nowcasting, developing ways to improve inferences of key economic indicators such as GDP.
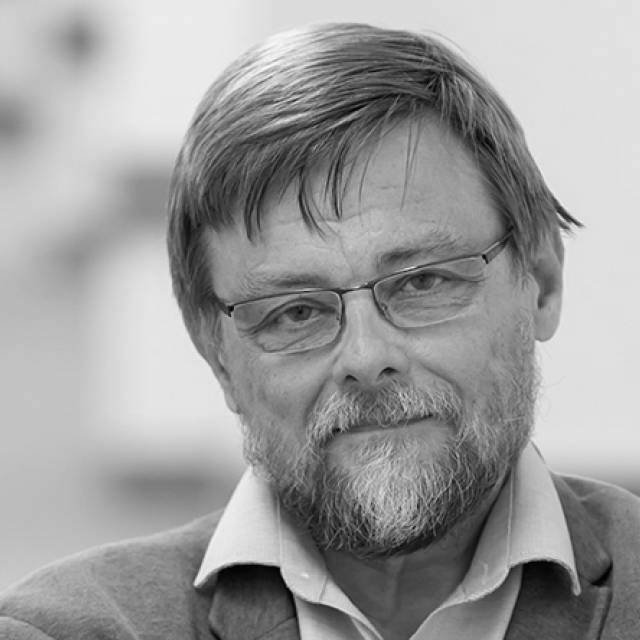
Terry Lyons
Terry Lyons is the Wallis Professor Emeritus and Professor of Mathematics at Oxford. He works on rough paths, stochastic analysis, and their applications in finance, developing mathematical tools to effectively model and describe high dimensional systems that exhibit randomness.
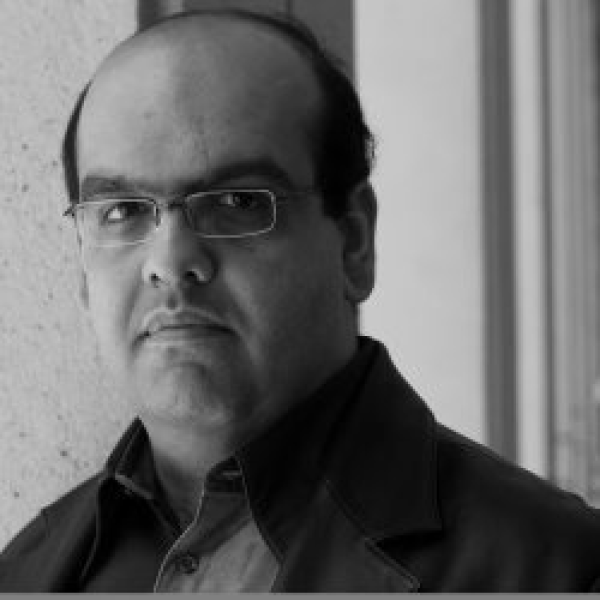
Rama Cont
Prof. Rama Cont is a Professor of Mathematics at Oxford, where he holds the Chair of Mathematical Finance. He has served as advisor to the IMF. His work focuses on stochastic processes and mathematical modeling in finance, with a focus on market instabilities and systemic risk.
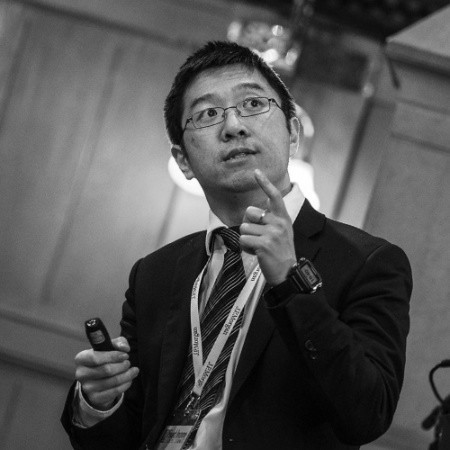
Jian Chen
Jian Chen is a research analyst at Point72 focusing on developing and trading FX vol strategies. He was the Head of Data Science of FairXChange and Head of Quantitative Solutions and Innovations at Morgan Stanley. He holds a DPhil in Computer Science from University of Oxford.
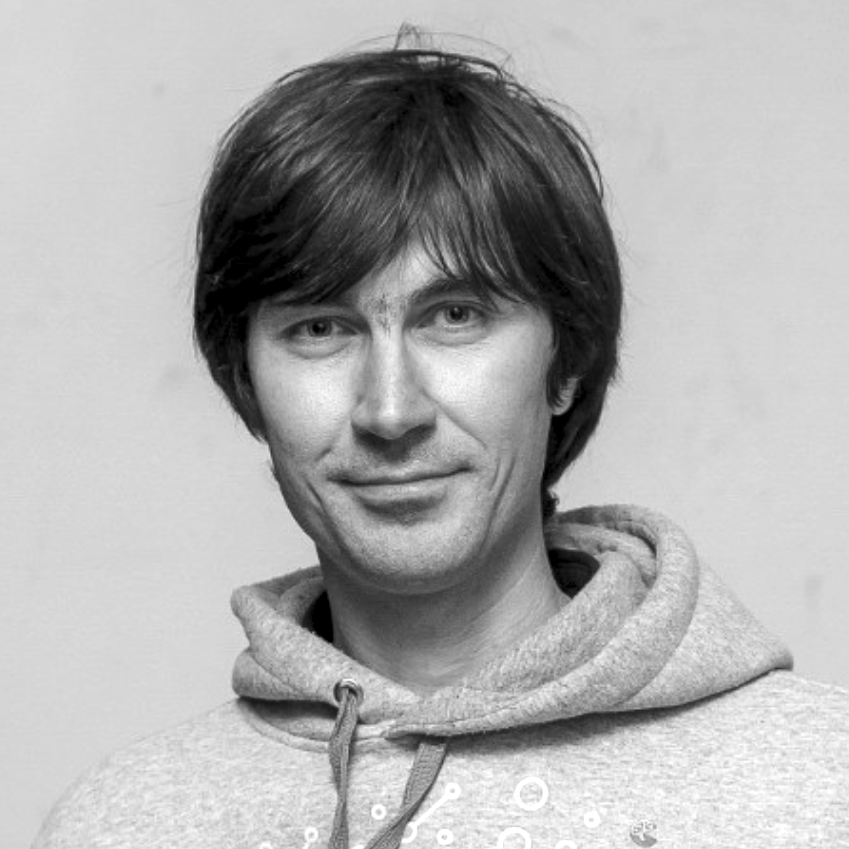
Mikhail Burtsev
Dr Mikhail Burtsev is a Landau AI Fellow at LIMS. He did his PhD at the Keldysh Institute, and has worked at the Anokhin and Kurchatov Institutes and Cambridge. He set up and led the Neural Nets and Deep Learning Lab at MIPT, which created the award-winning framework, DeepPavlov.
12:40
Ending remarks
12:45