What you see is not what you get: how sampling affects macroscopic features of biological networks
Methods from tailored random graph theory reveal the relation between true biological networks and the often-biased samples taken from them.
A. Annibale, A. Coolen
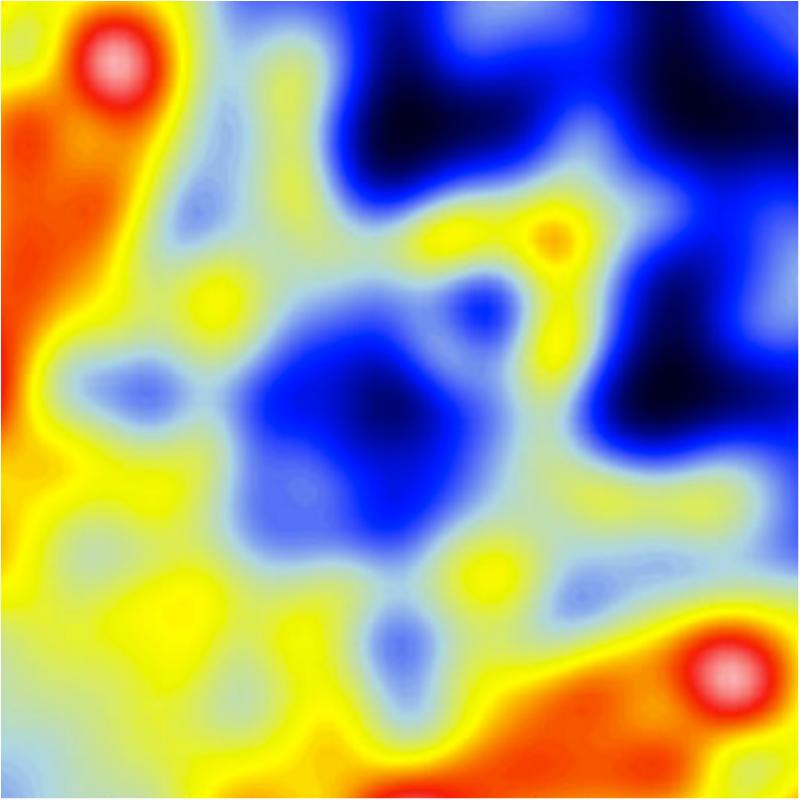
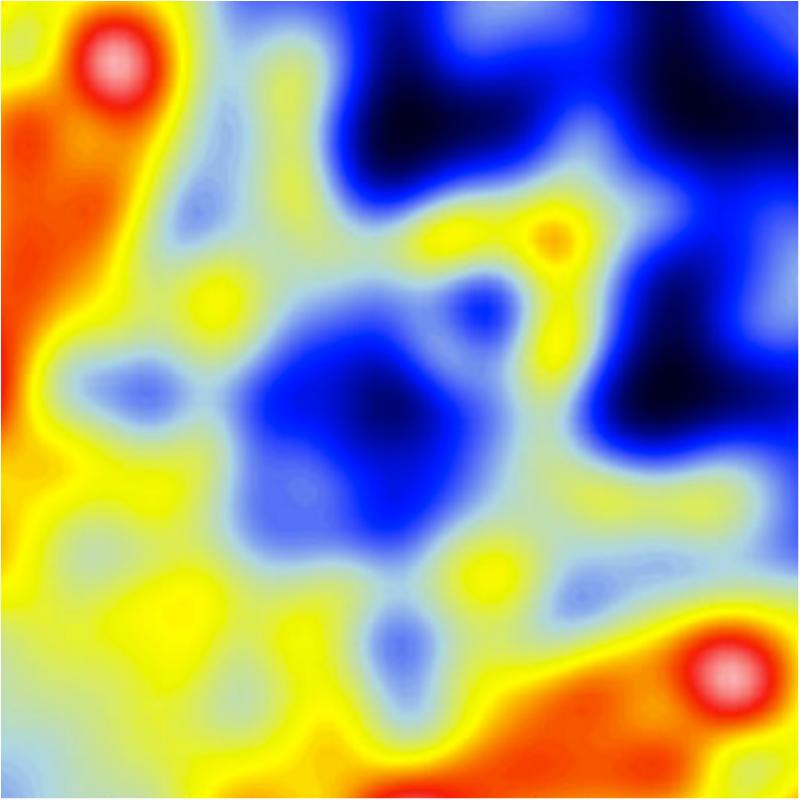
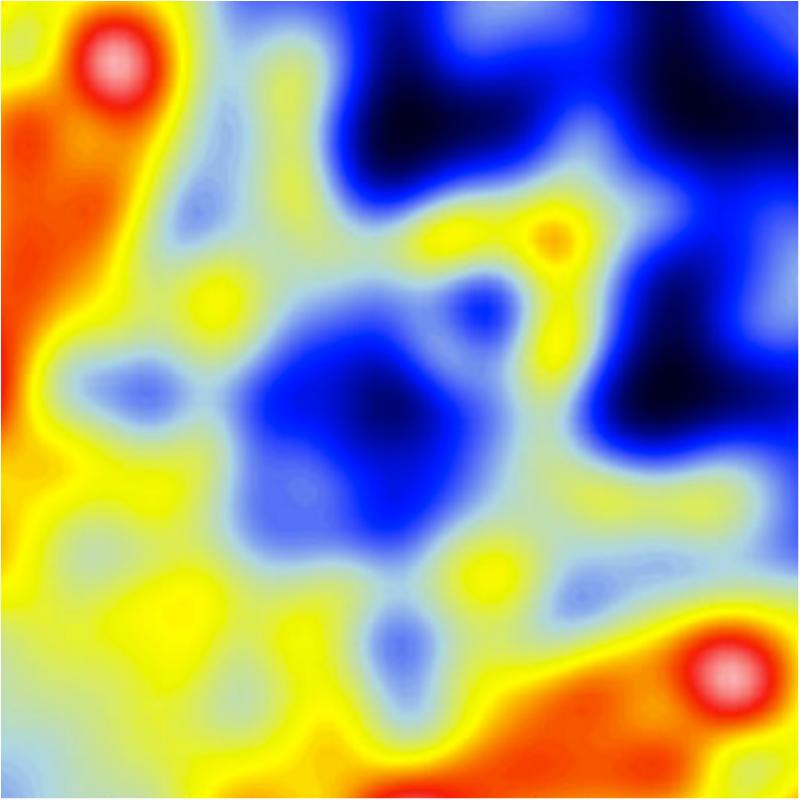
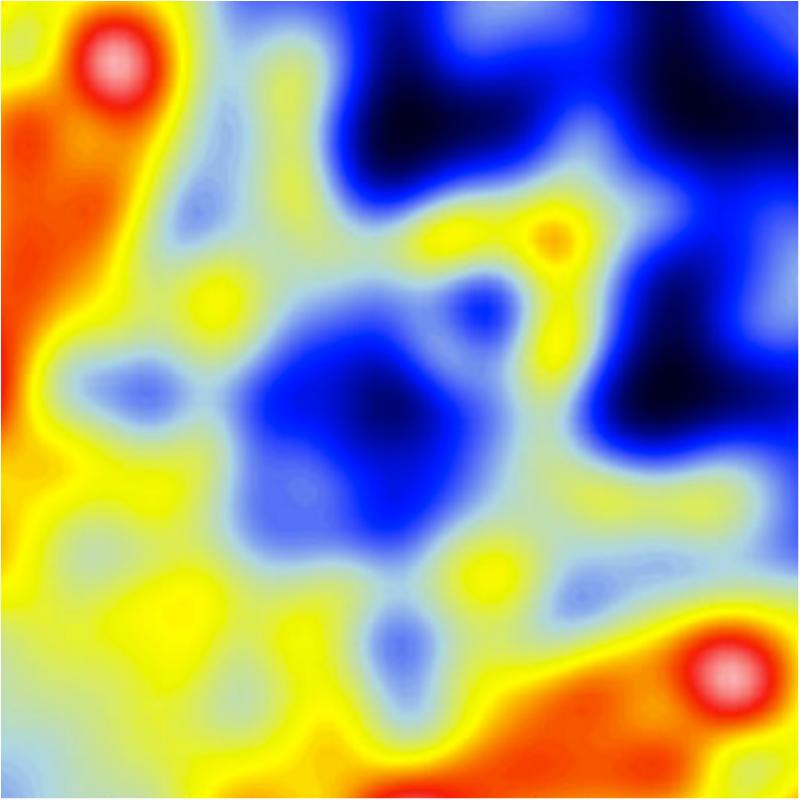
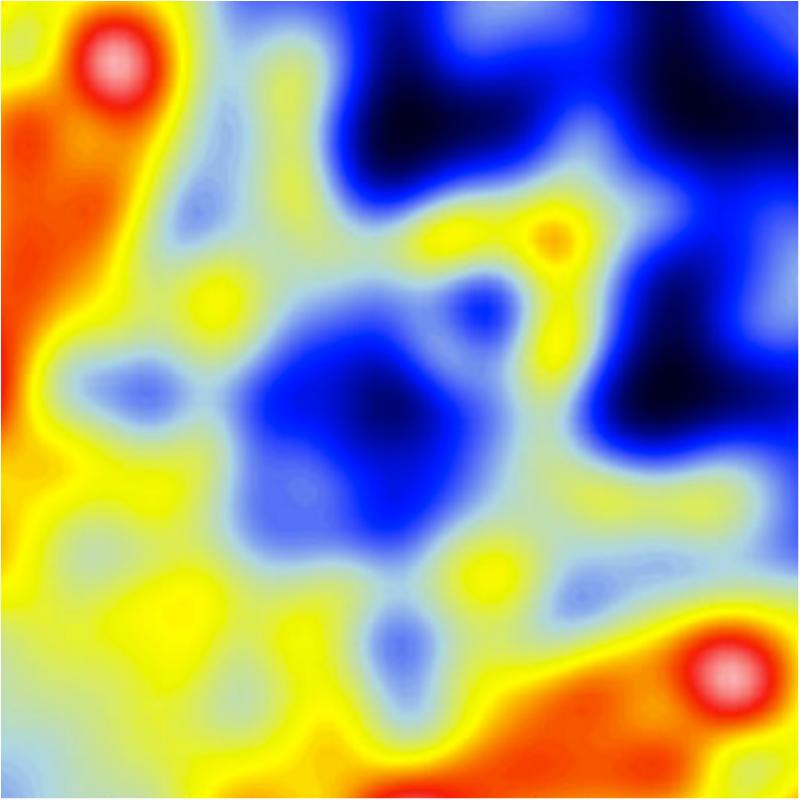
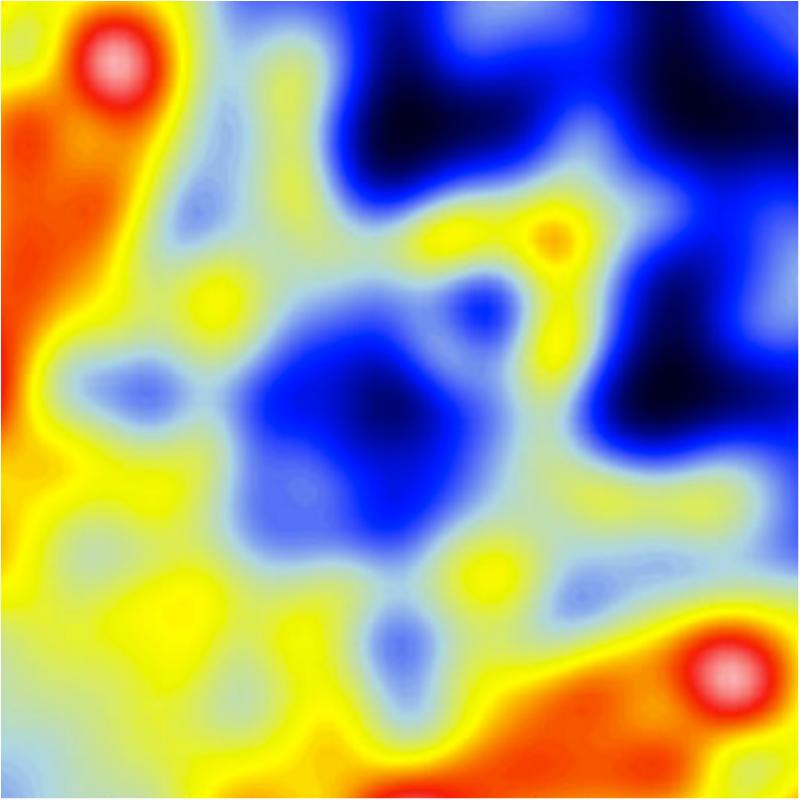
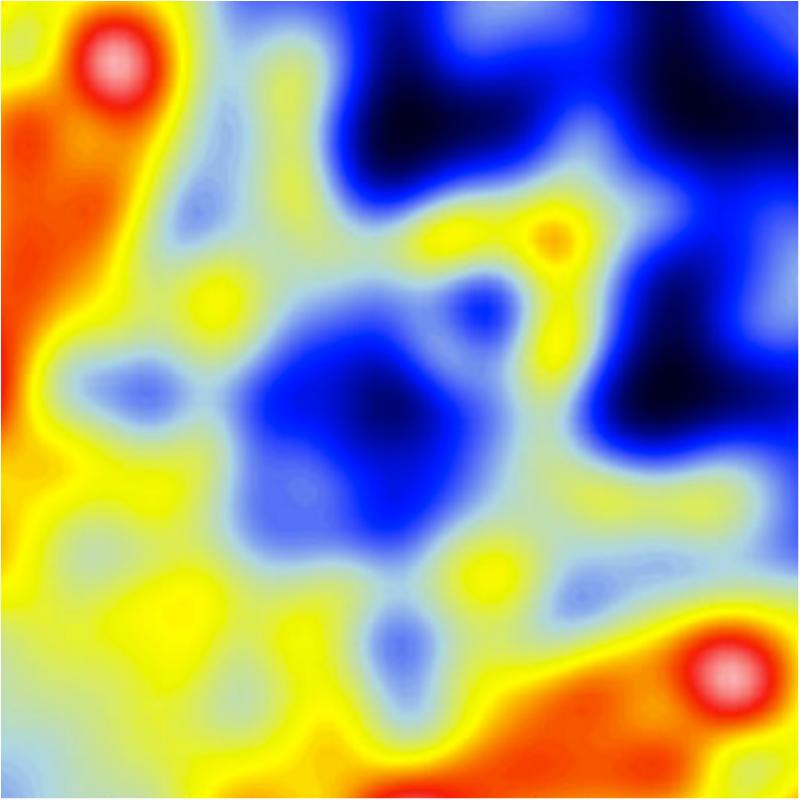
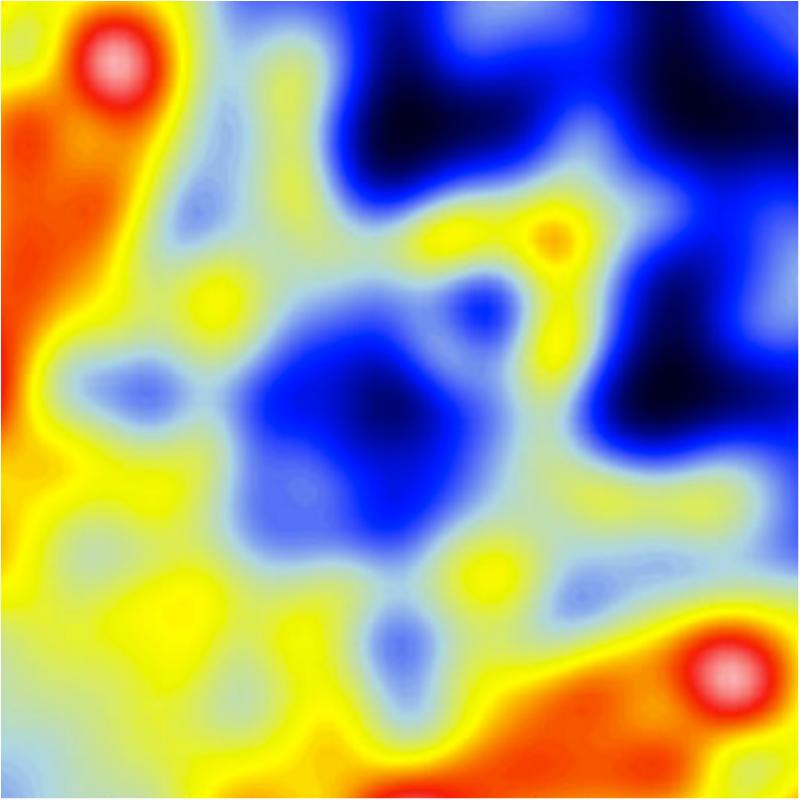
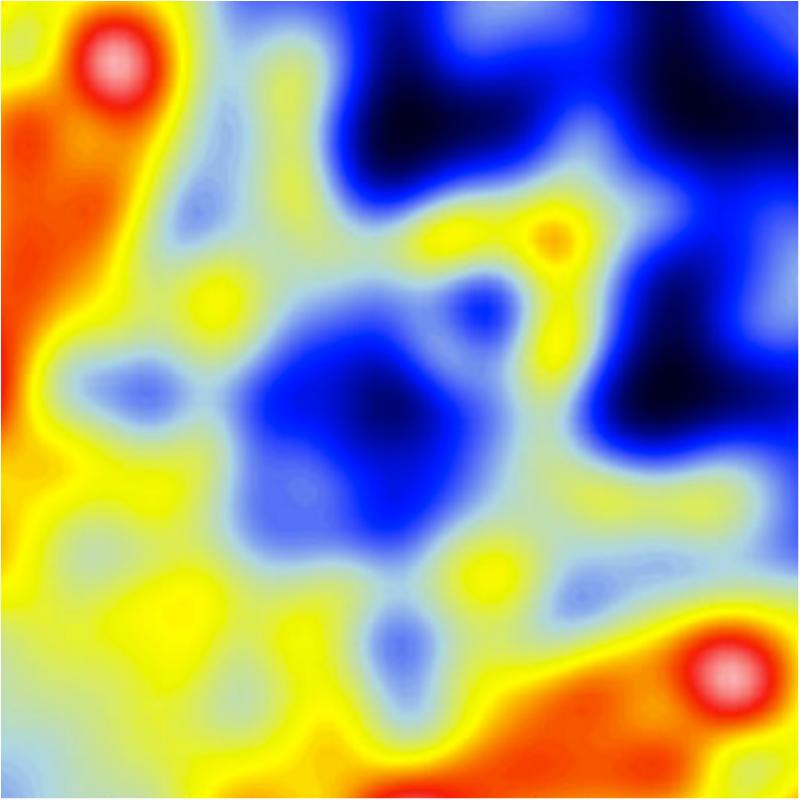
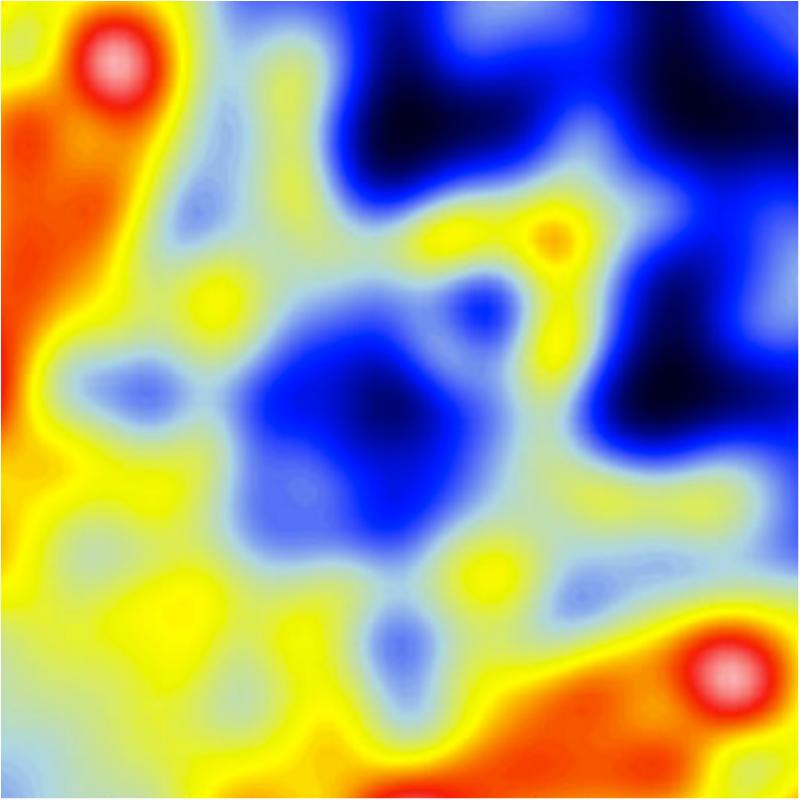
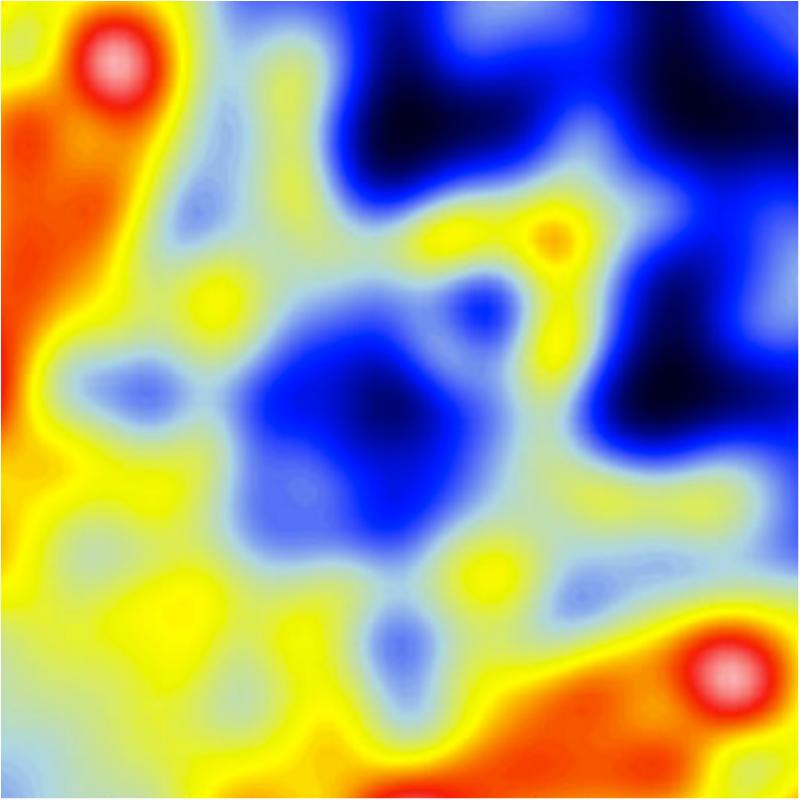
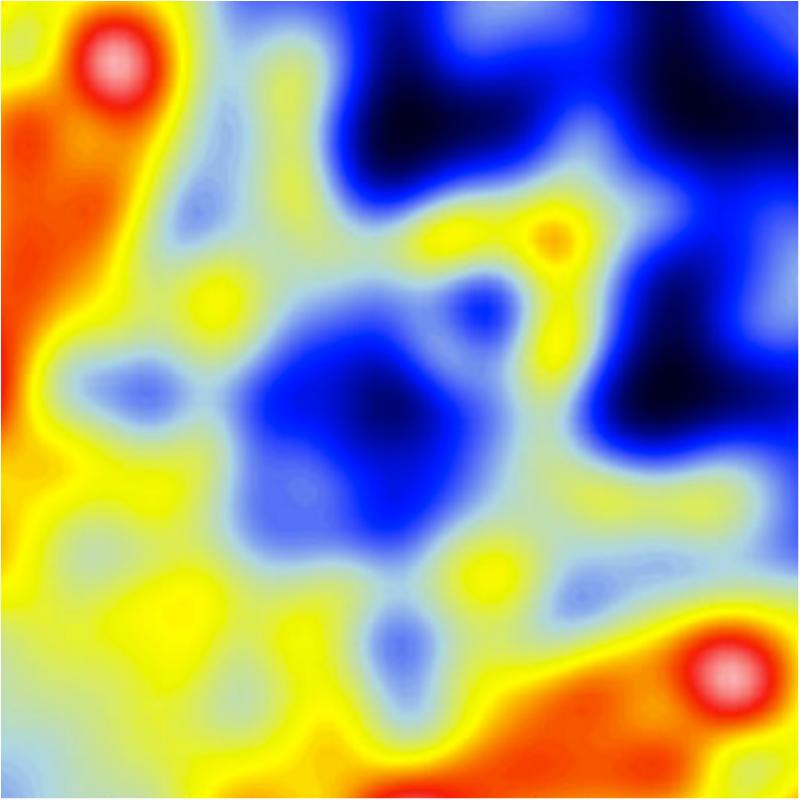
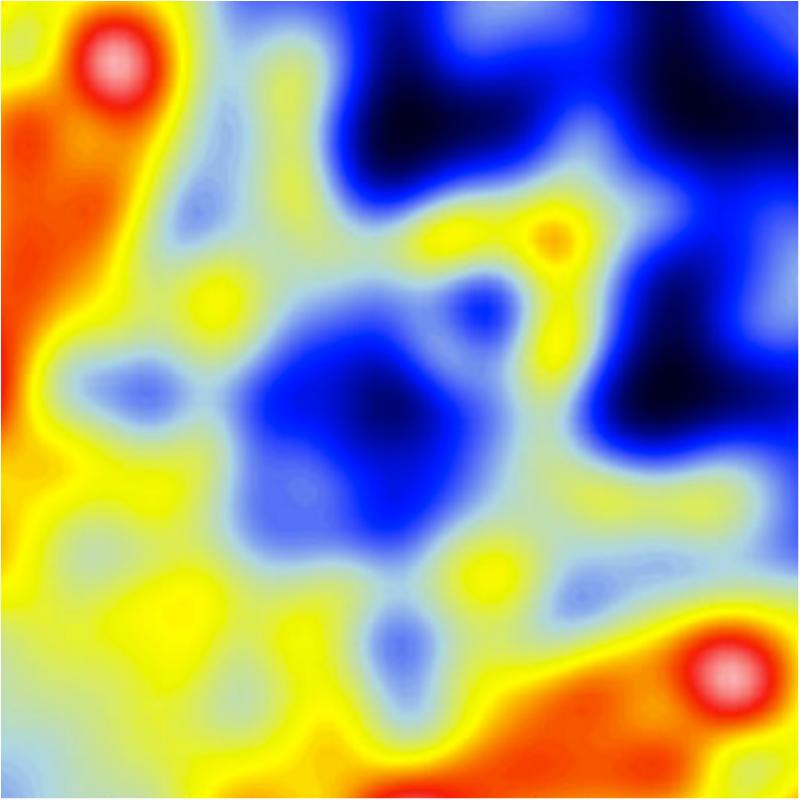
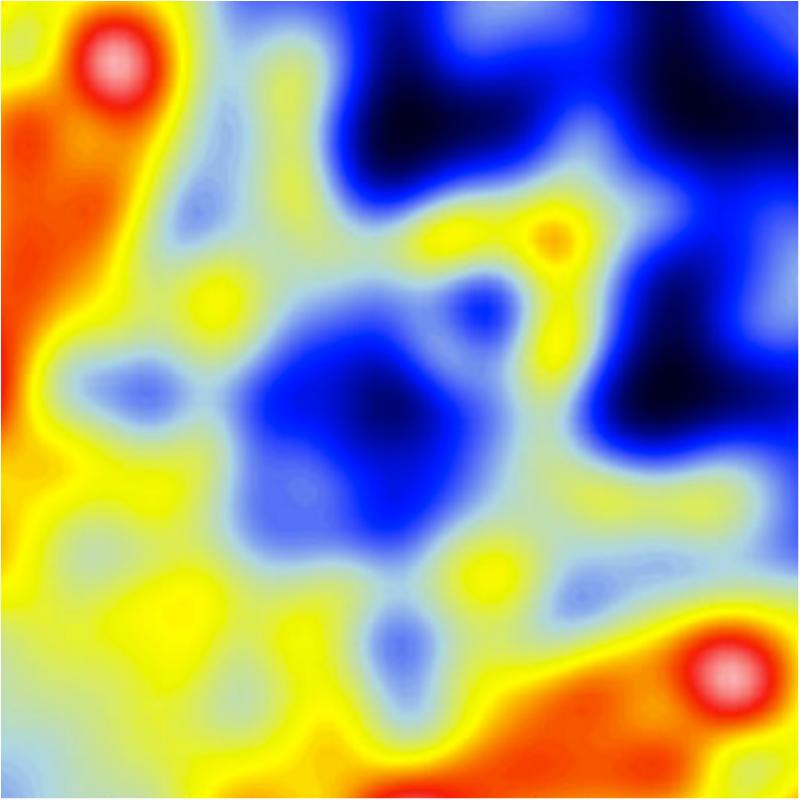
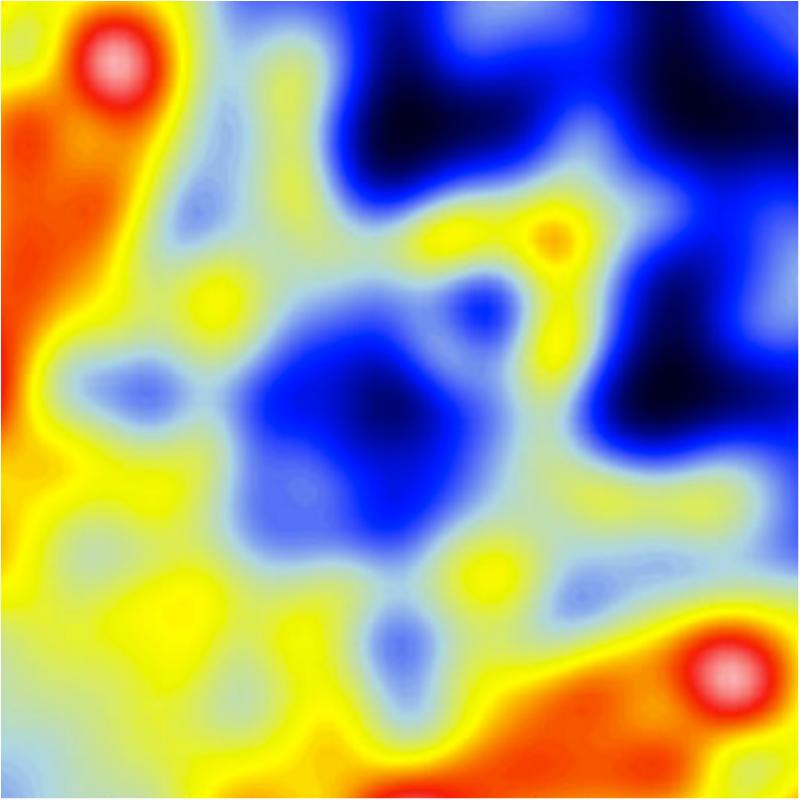
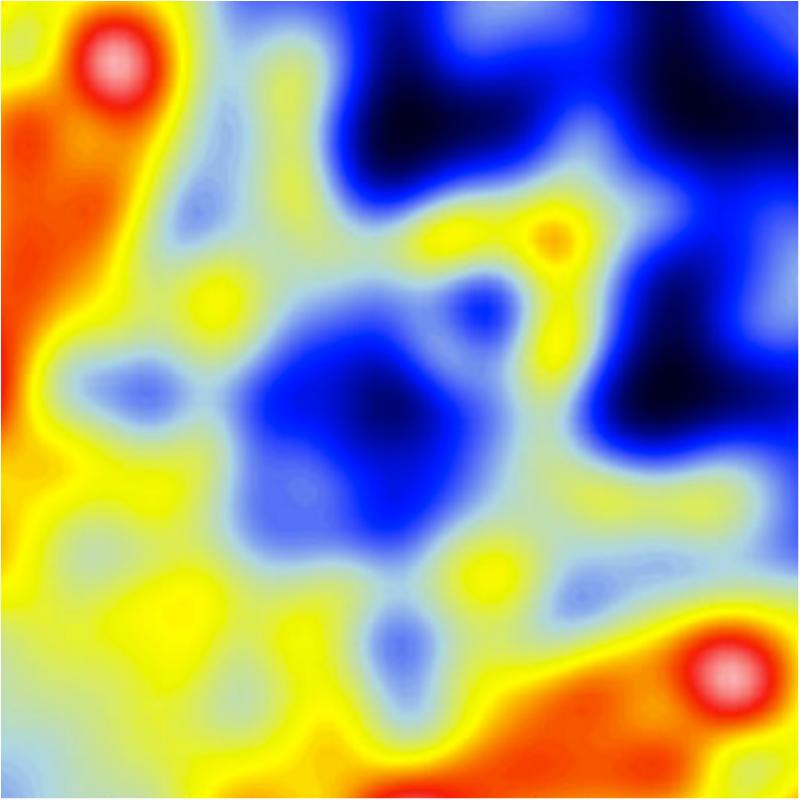
We use mathematical methods from the theory of tailored random graphs to study systematically the effects of sampling on topological features of large biological signalling networks. Our aim in doing so is to increase our quantitative understanding of the relation between true biological networks and the imperfect and often biased samples of these networks that are reported in public data repositories and used by biomedical scientists. We derive exact explicit formulae for degree distributions and degree correlation kernels of sampled networks, in terms of the degree distributions and degree correlation kernels of the underlying true network, for a broad family of sampling protocols that include random and connectivity-dependent node and/or link undersampling as well as random and connectivity-dependent link oversampling. Our predictions are in excellent agreement with numerical simulations.