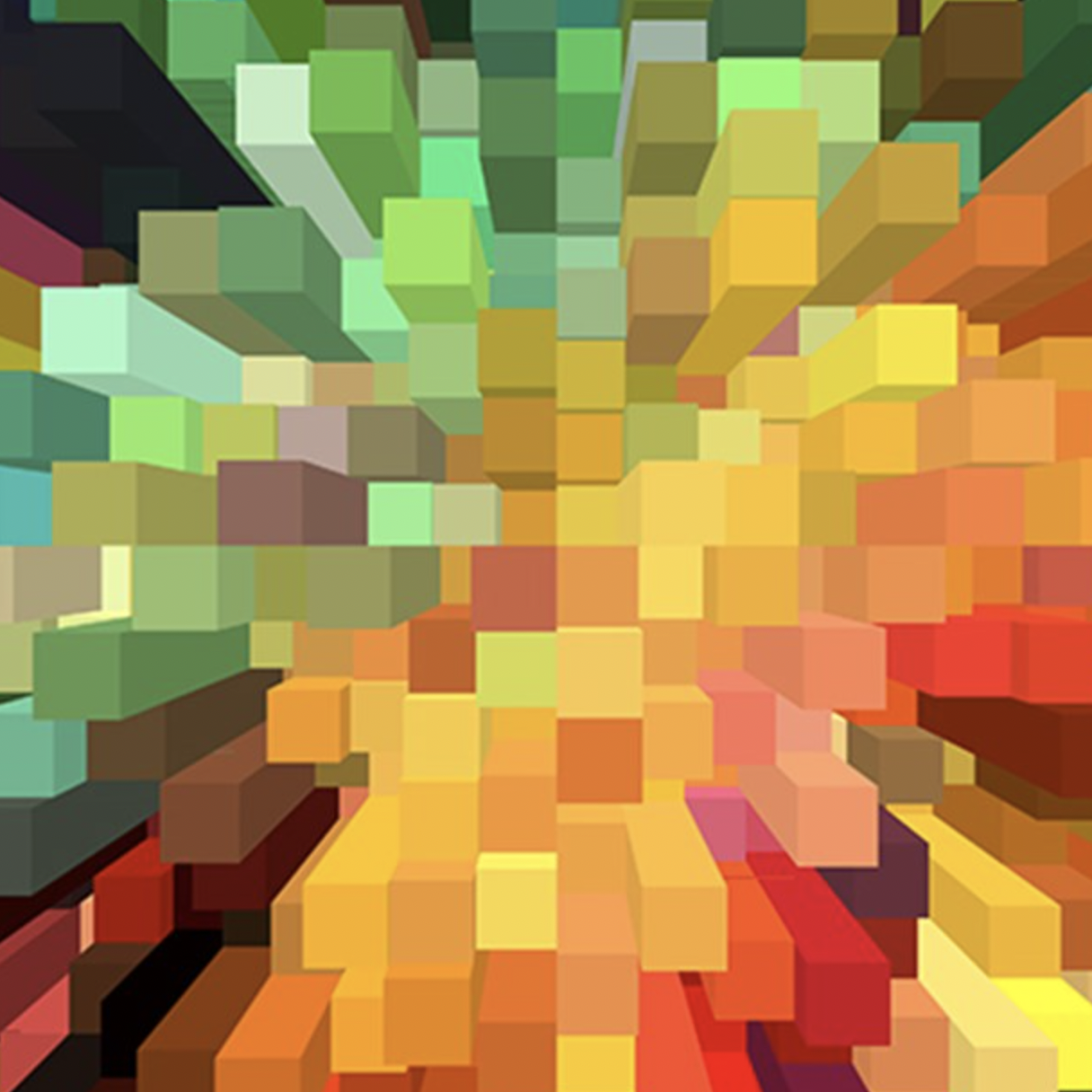
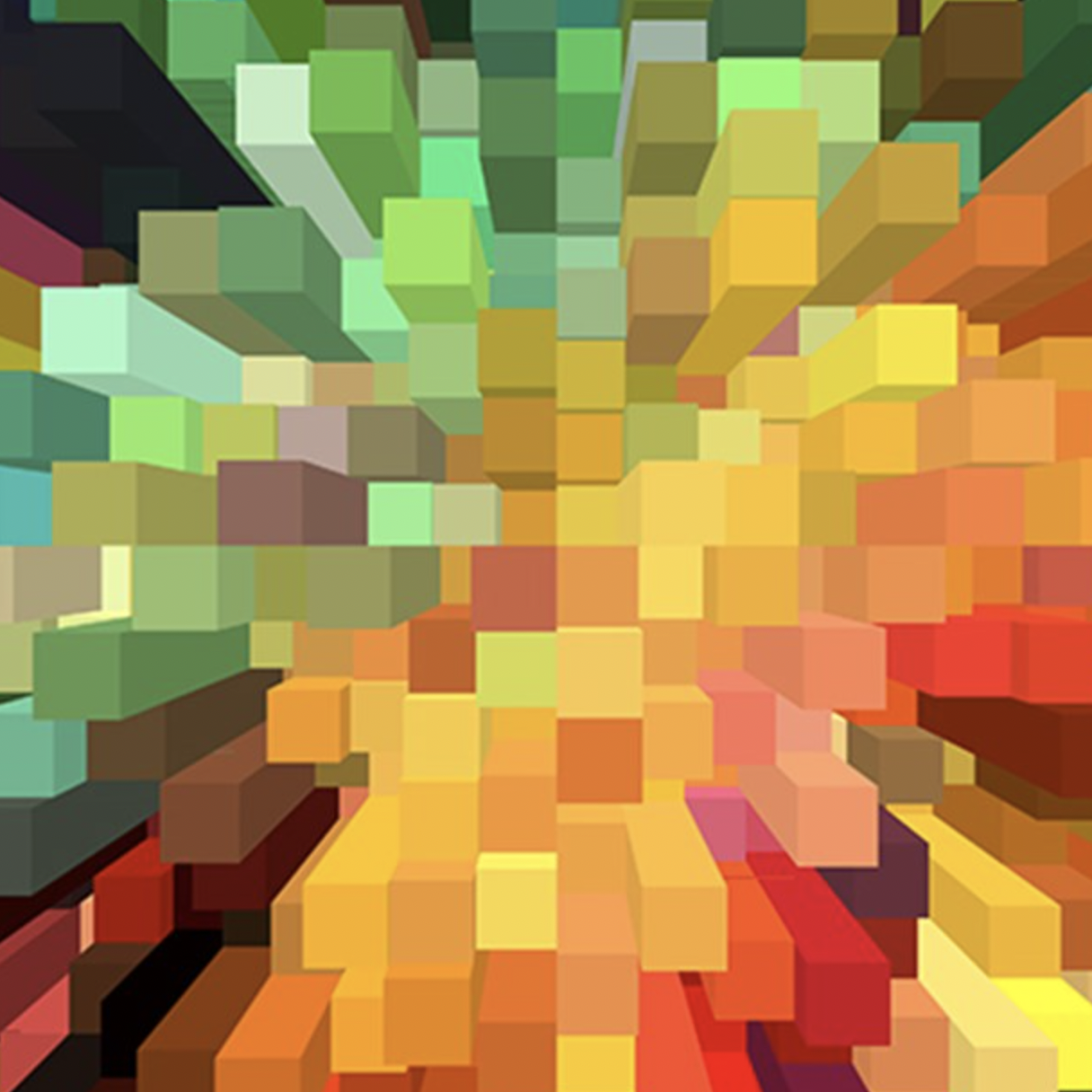
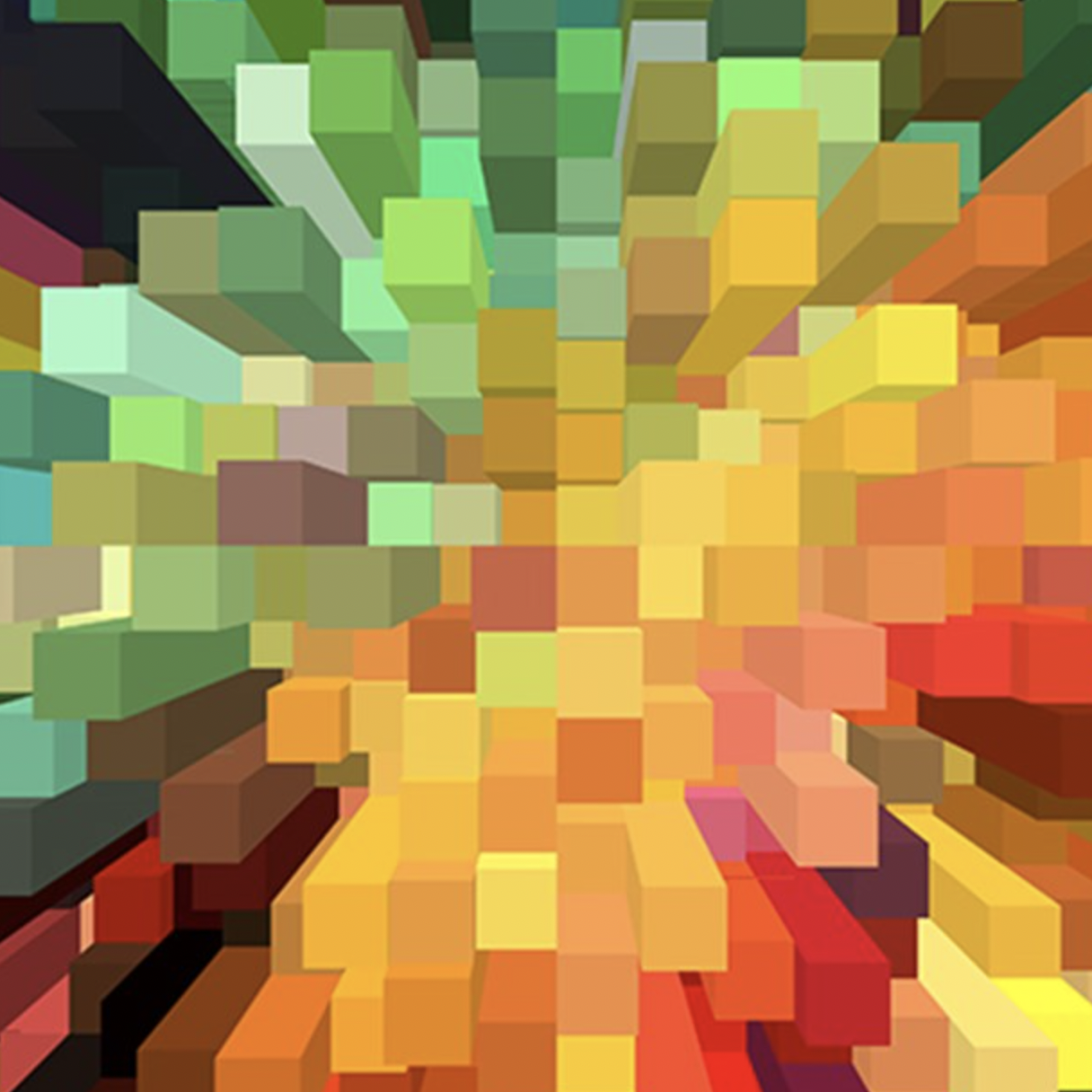
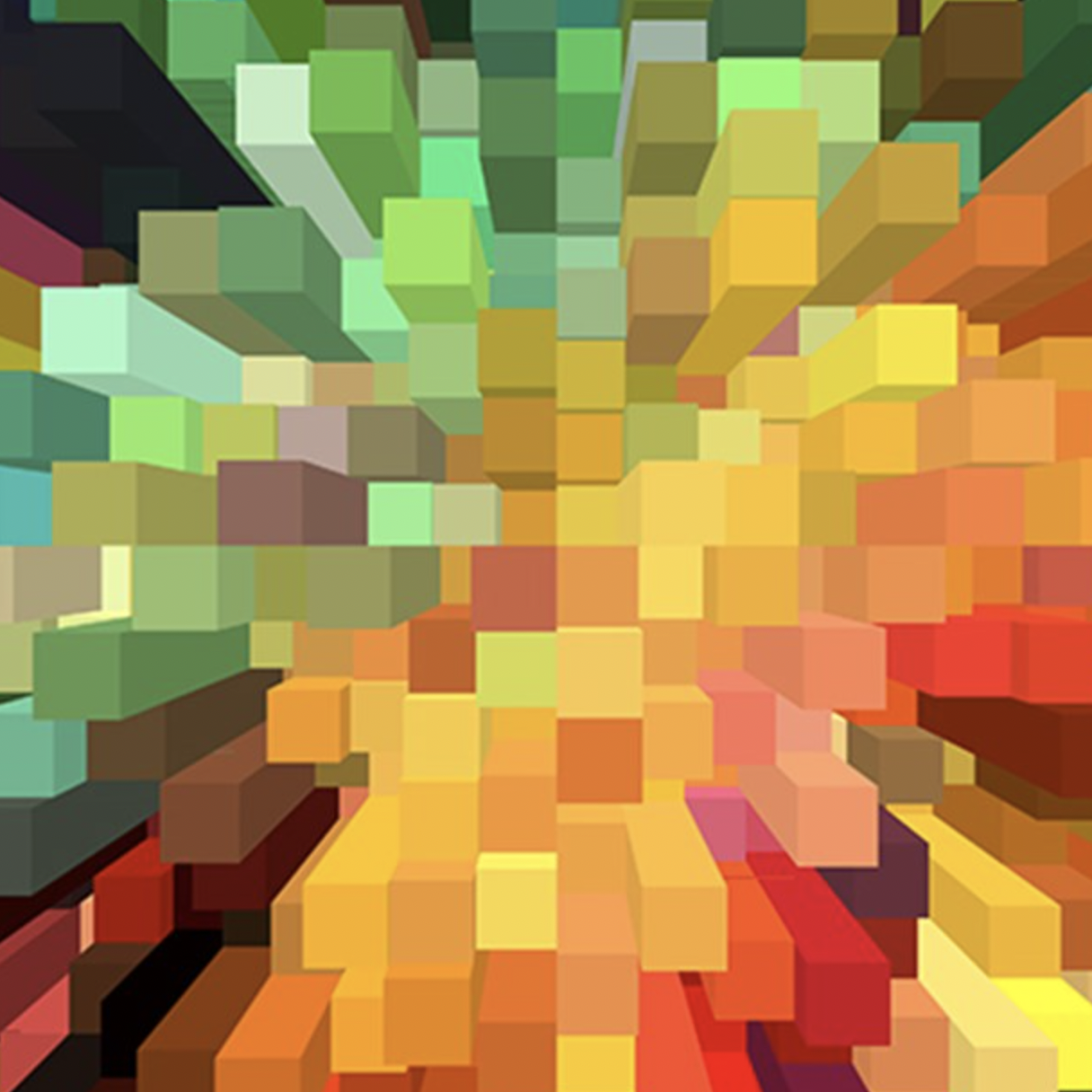
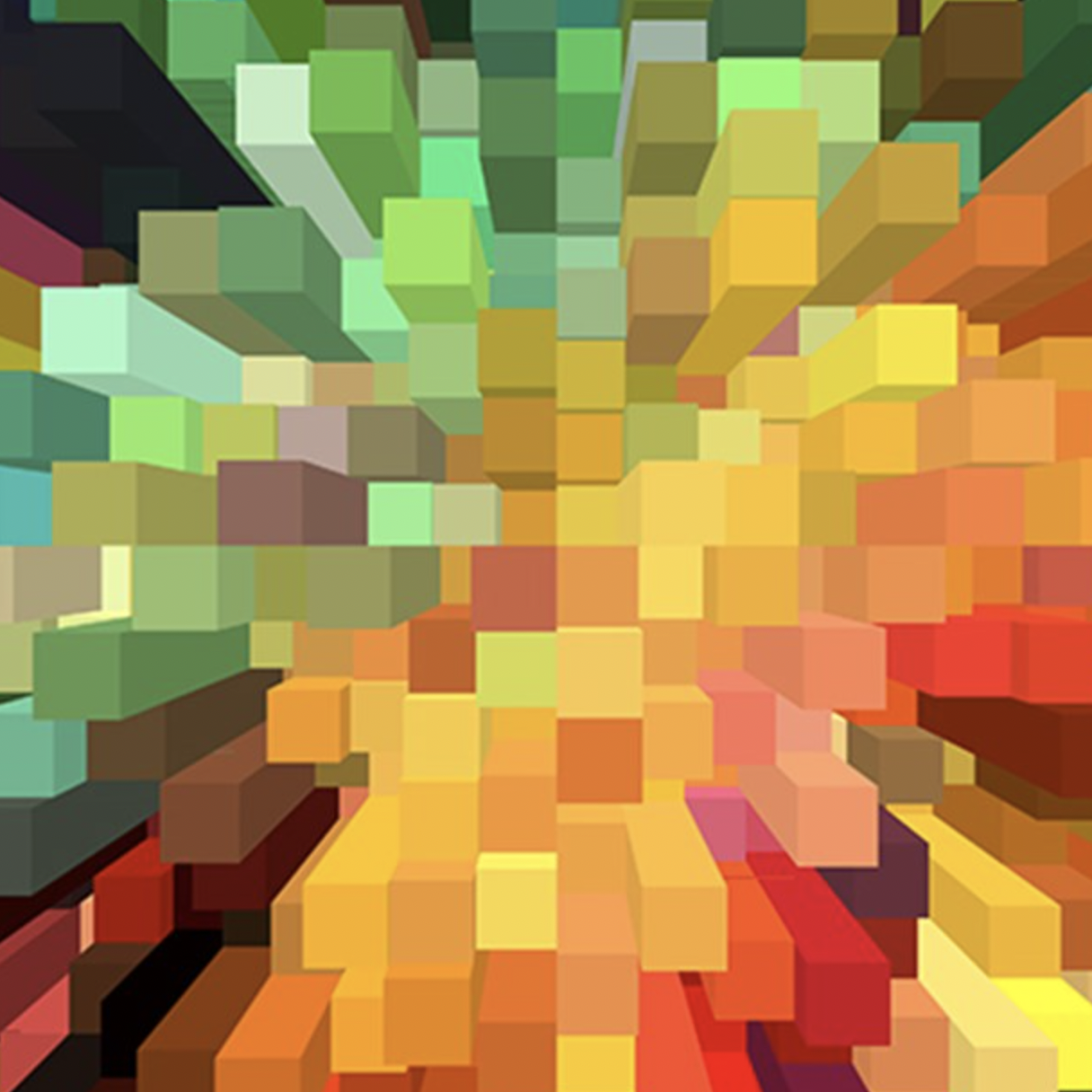
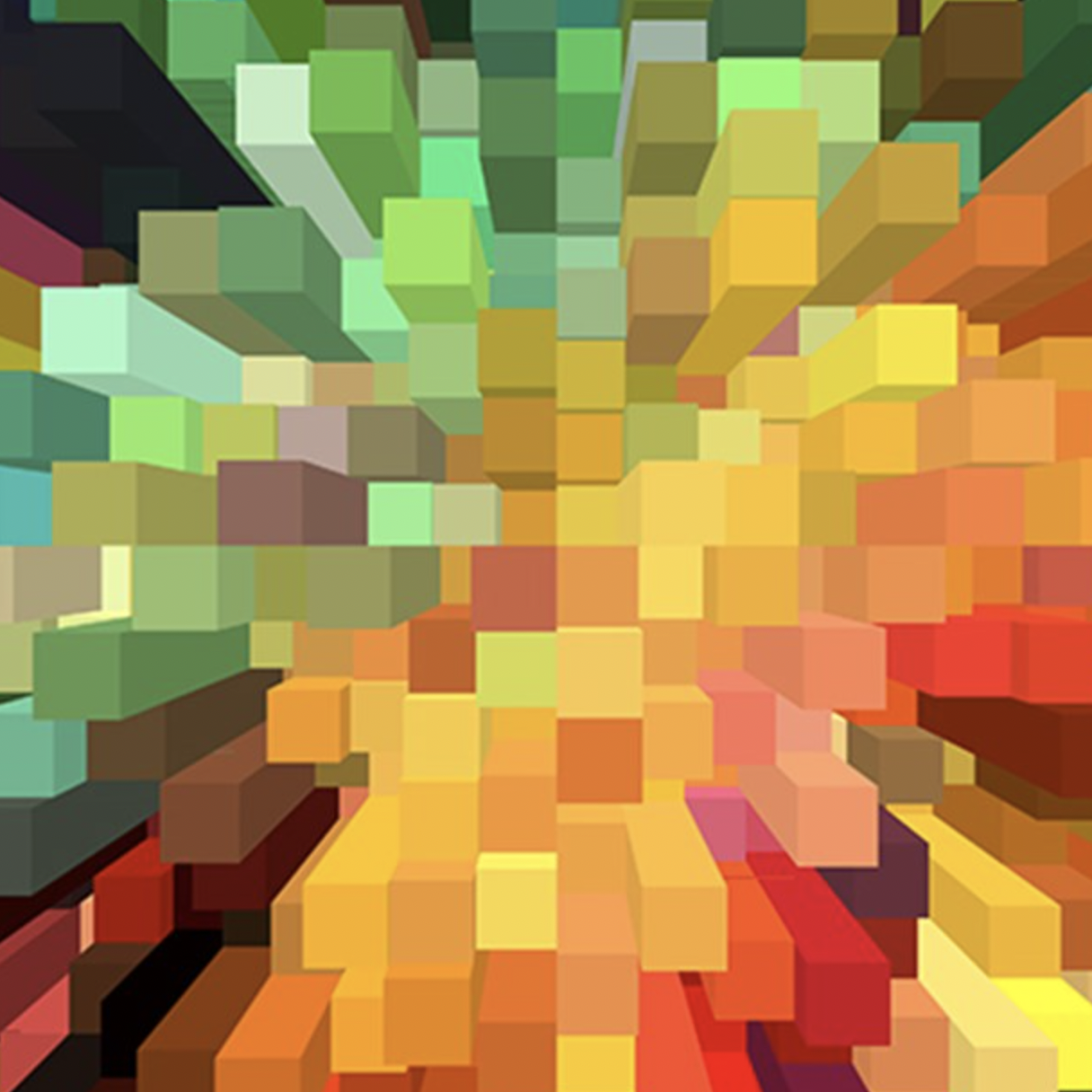
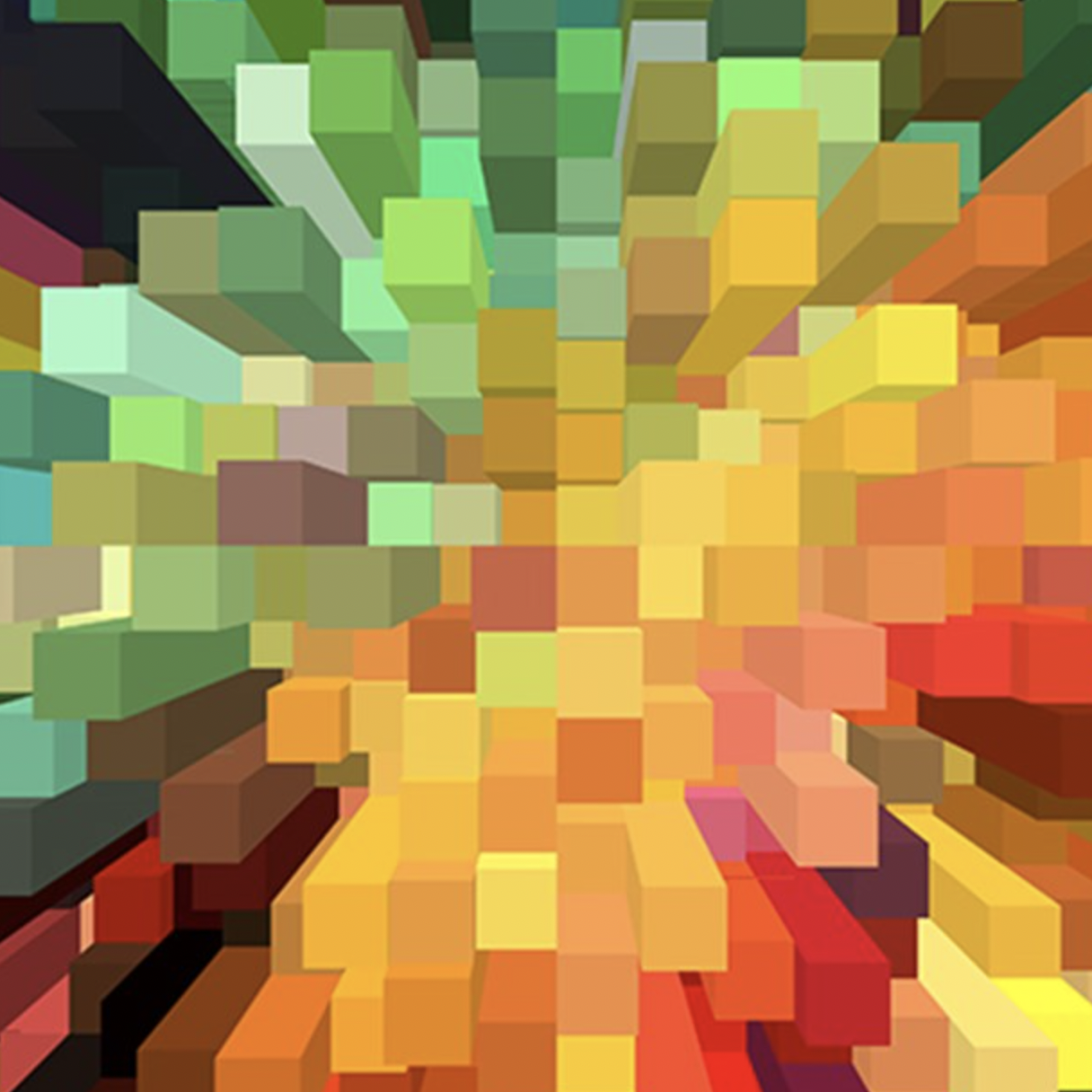
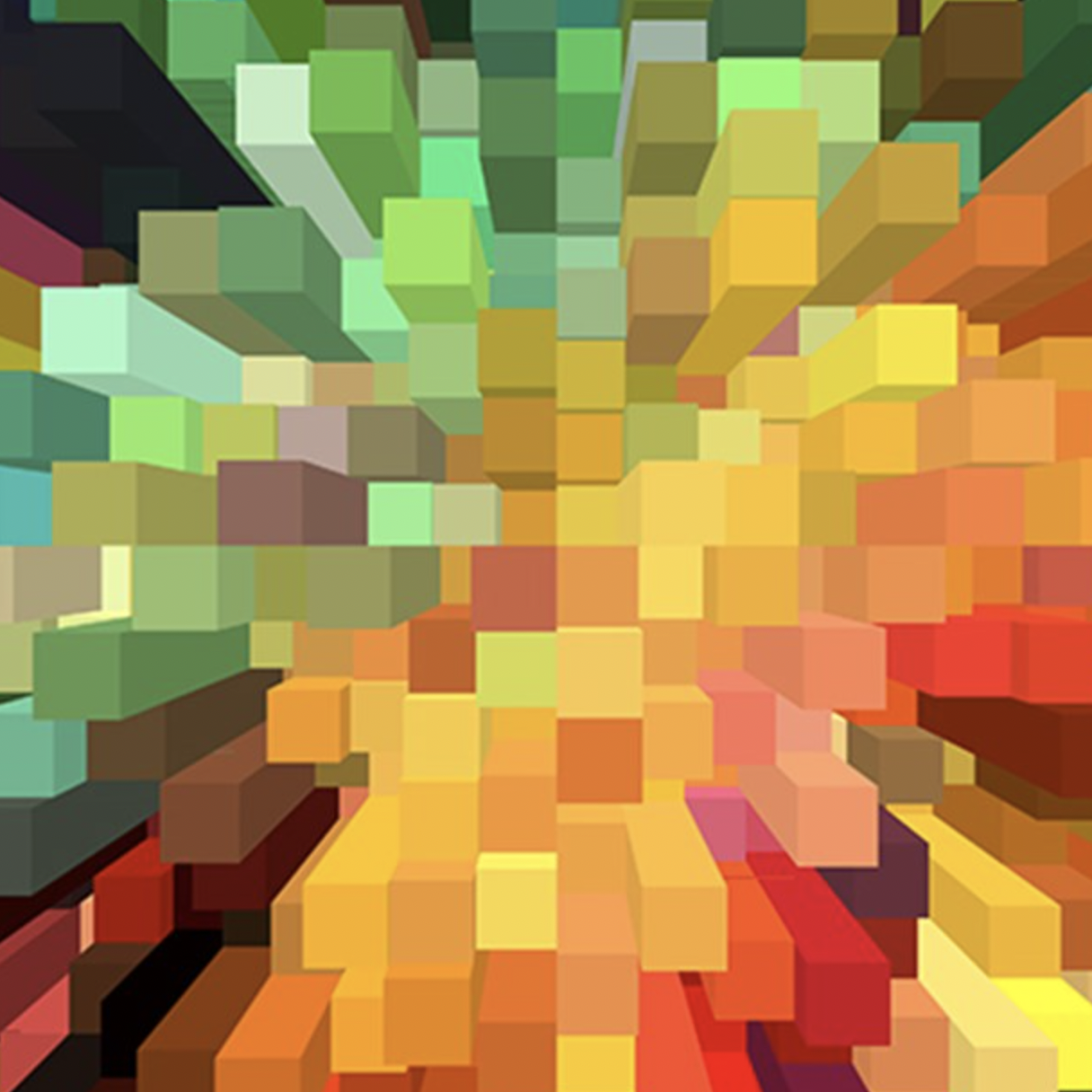
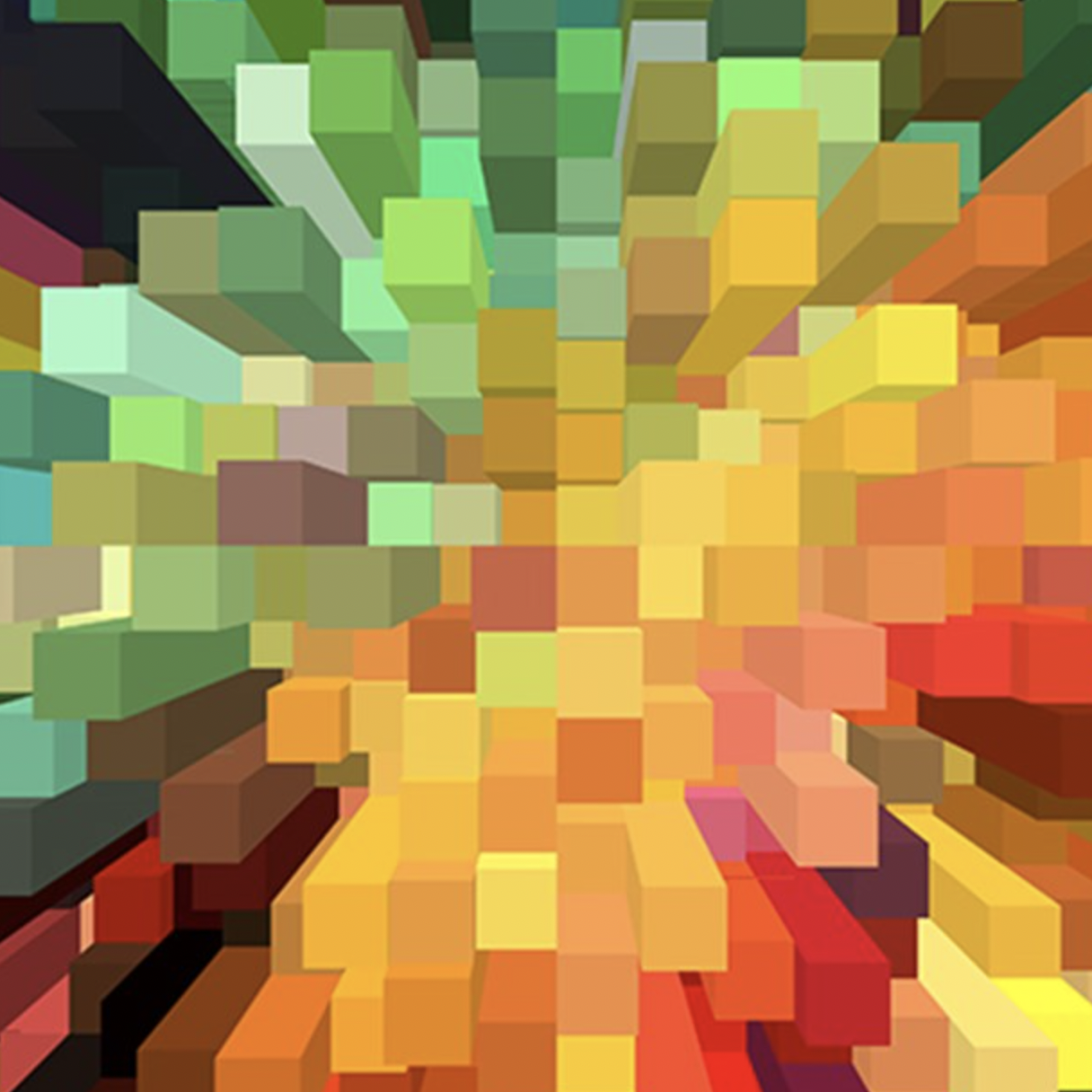
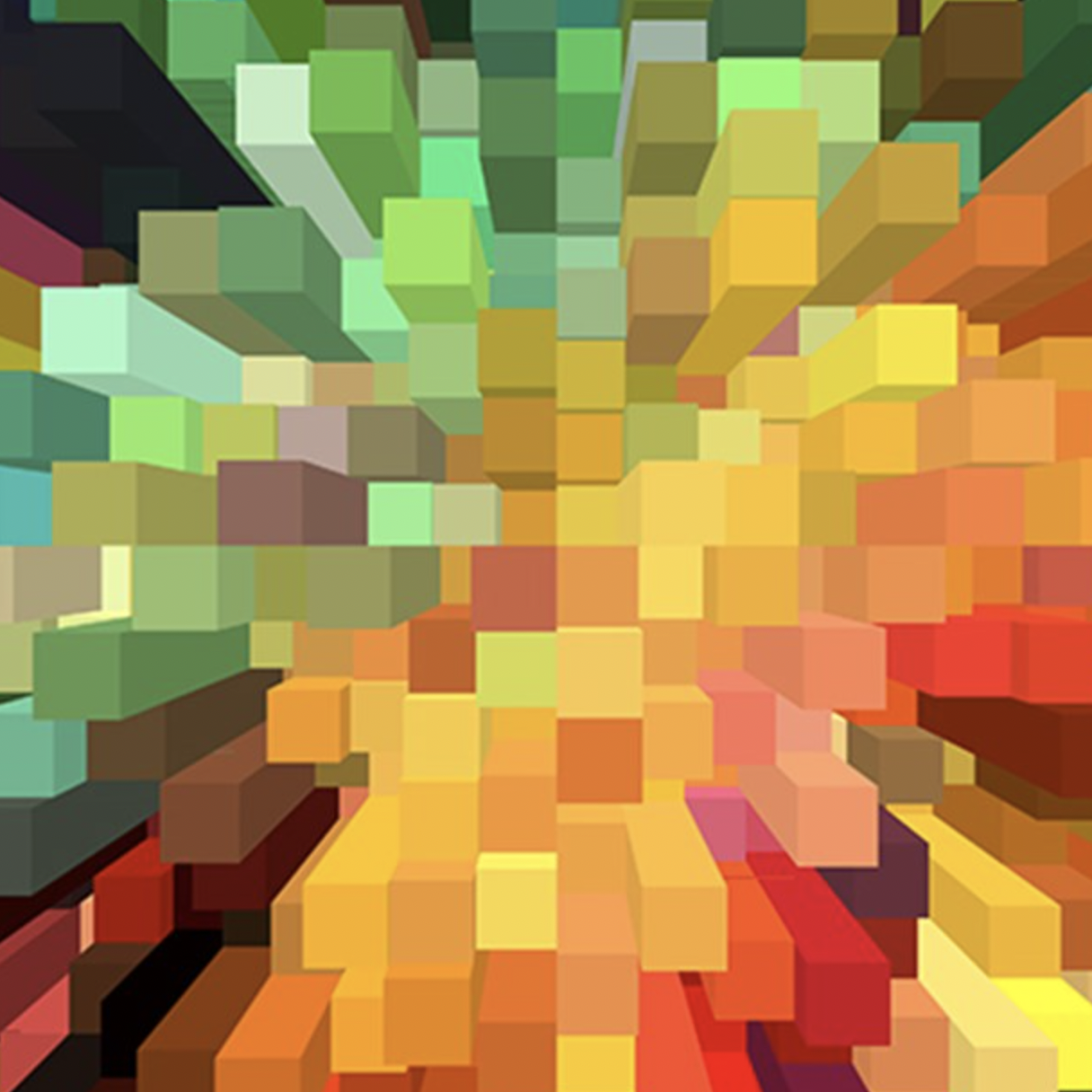
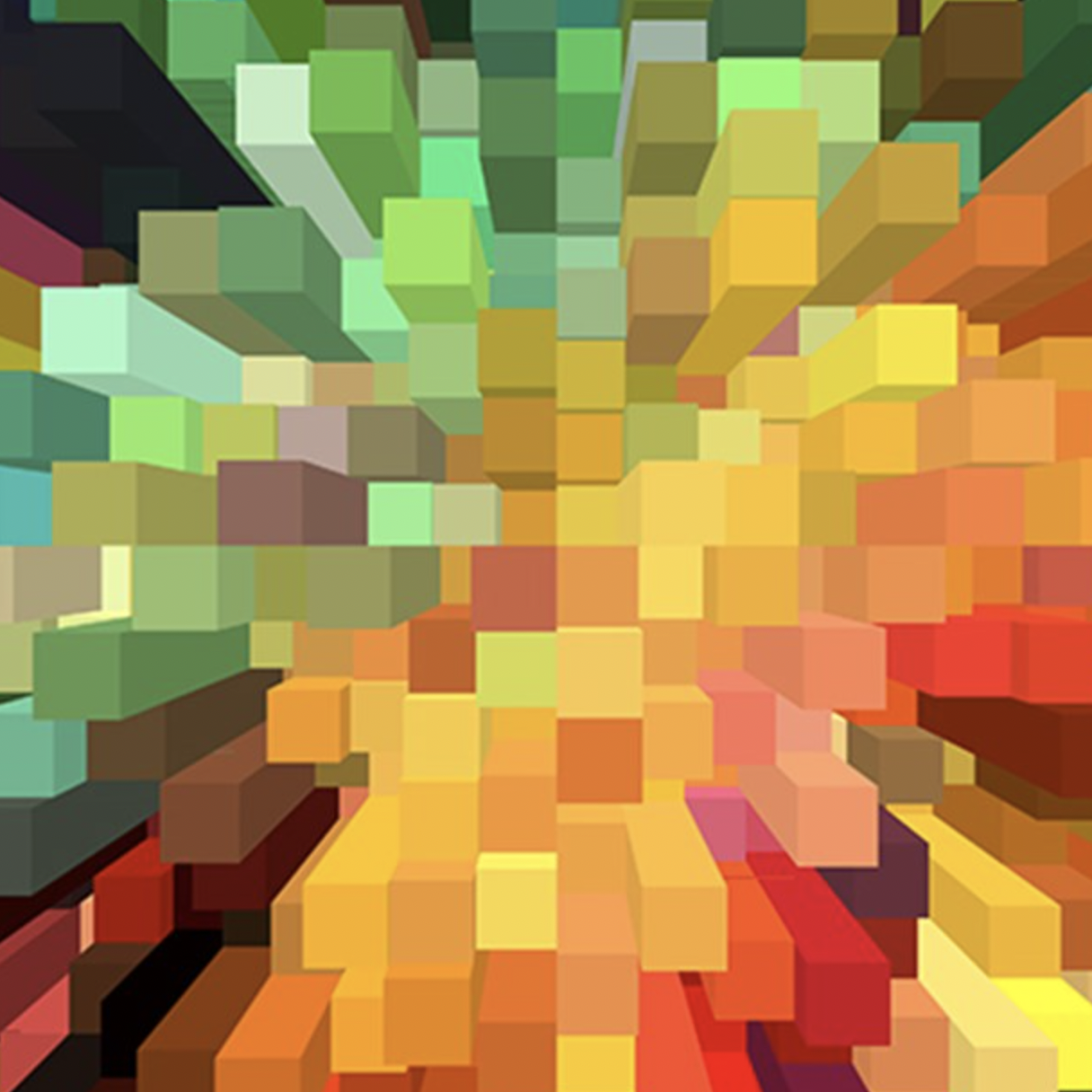
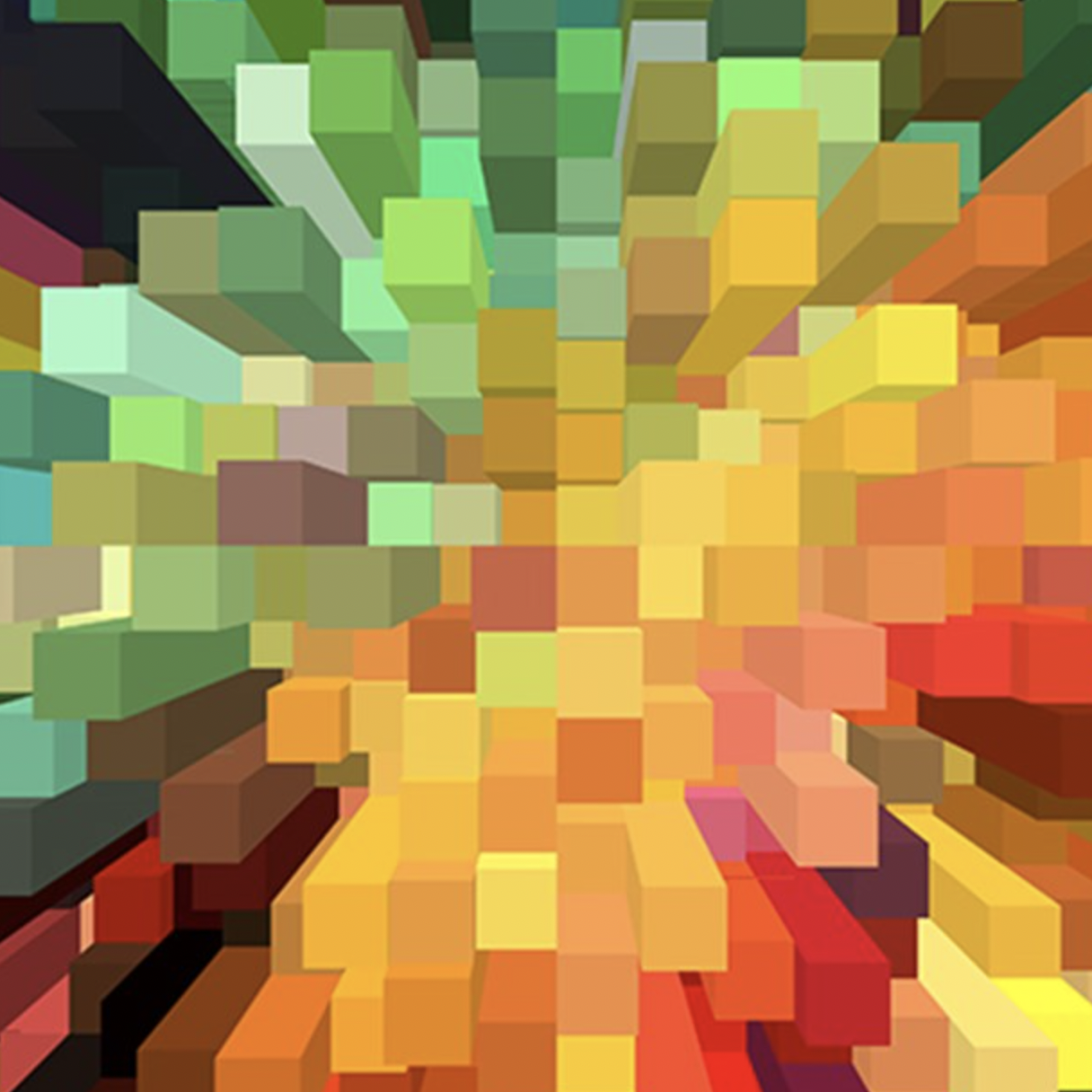
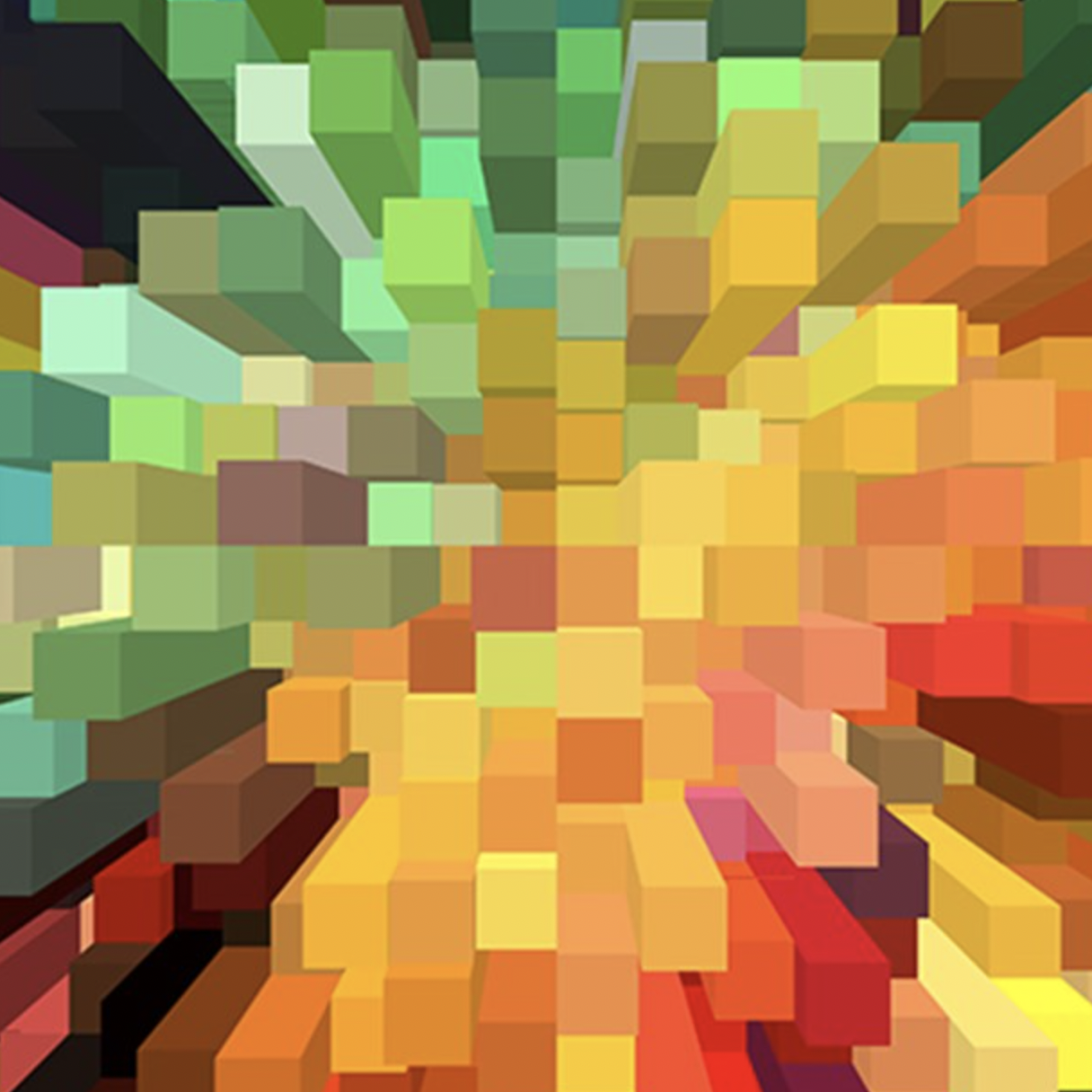
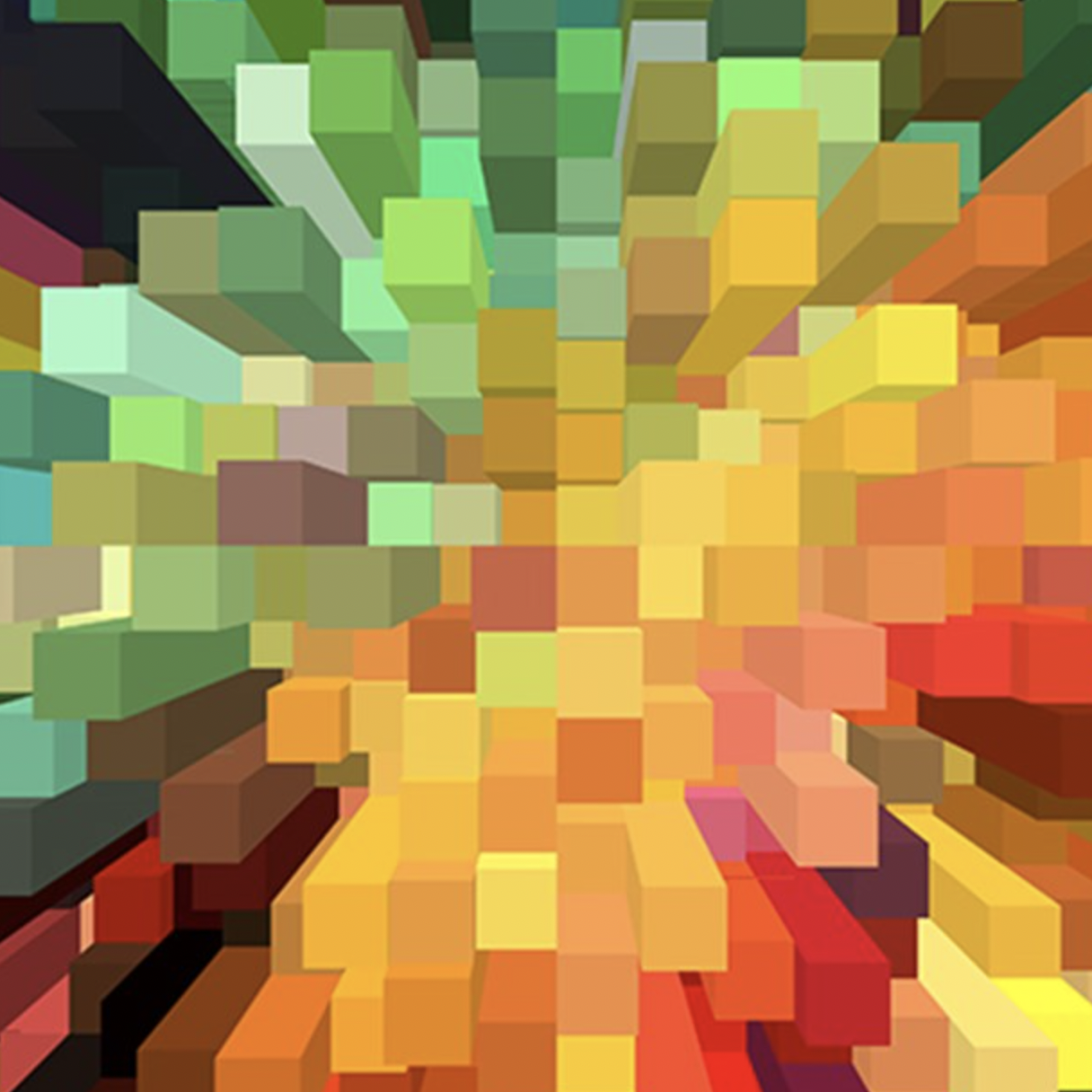
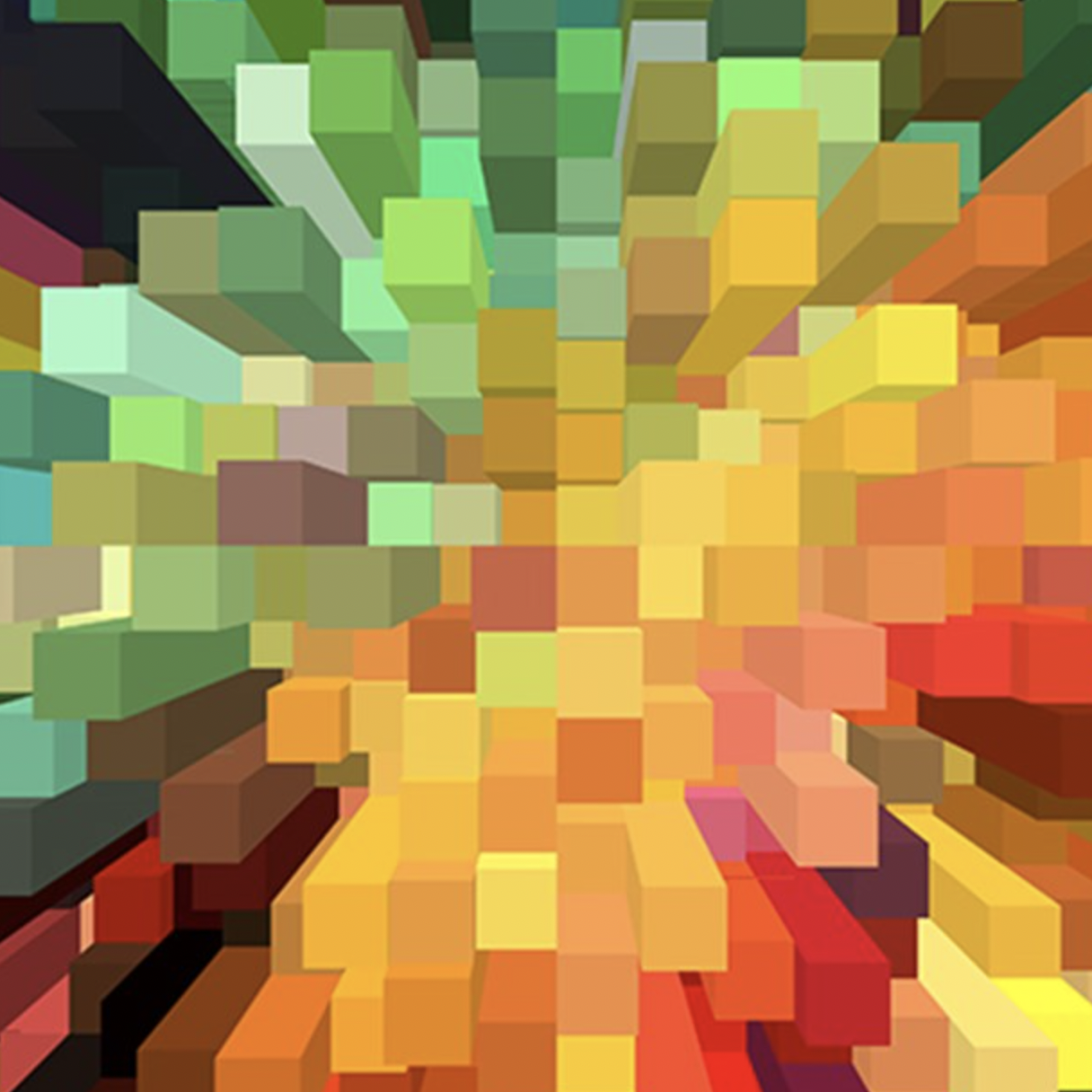
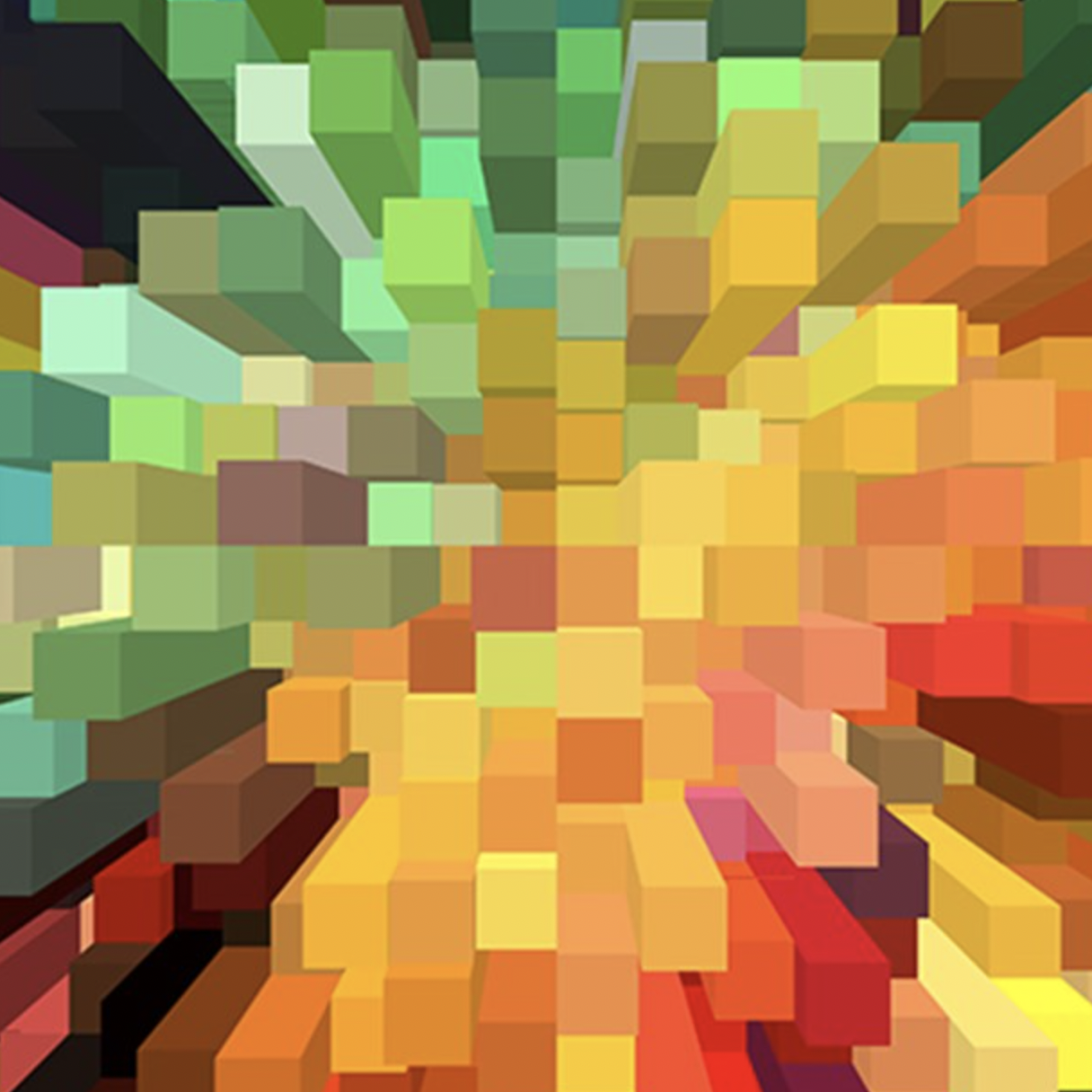
Line bundle connections
AI-assisted maths
Neural networks find numerical solutions to Hermitian Yang-Mills equations, a difficult system of PDEs crucial to mathematics and physics.
Machine learning line bundle connections
We study the use of machine learning for finding numerical hermitian Yang–Mills connections on line bundles over Calabi–Yau manifolds. Defining an appropriate loss function and focusing on the examples of an elliptic curve, a K3 surface and a quintic threefold, we show that neural networks can be trained to give a close approximation to hermitian Yang–Mills connections.