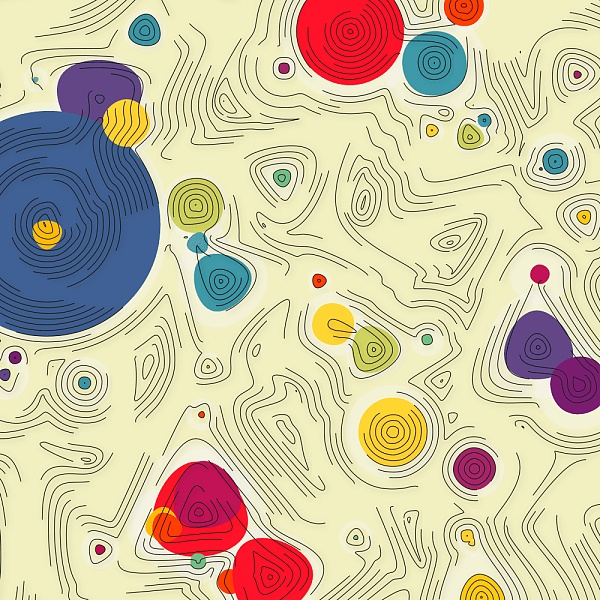
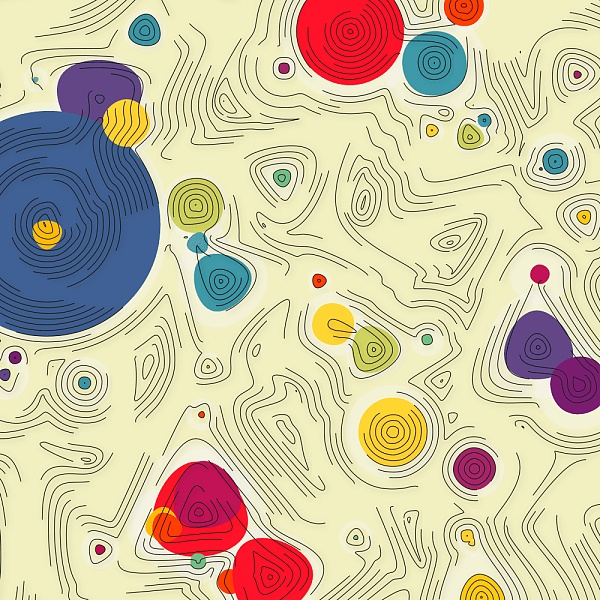
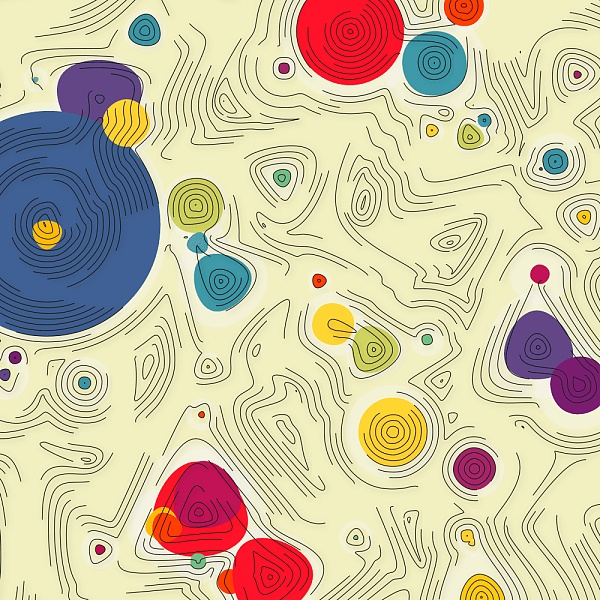
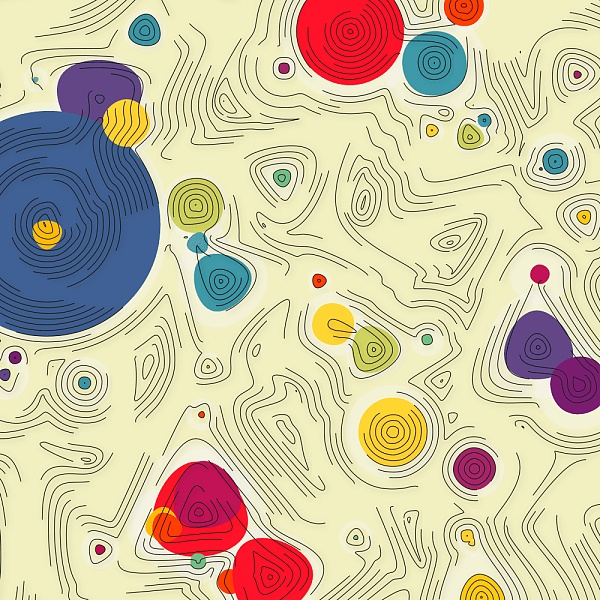
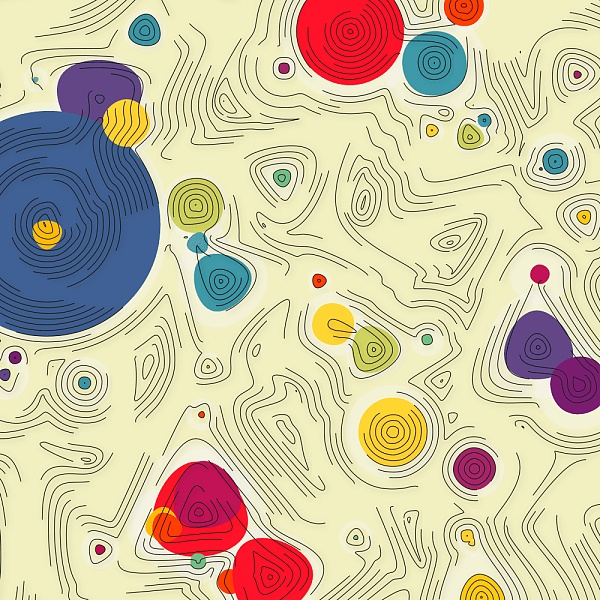
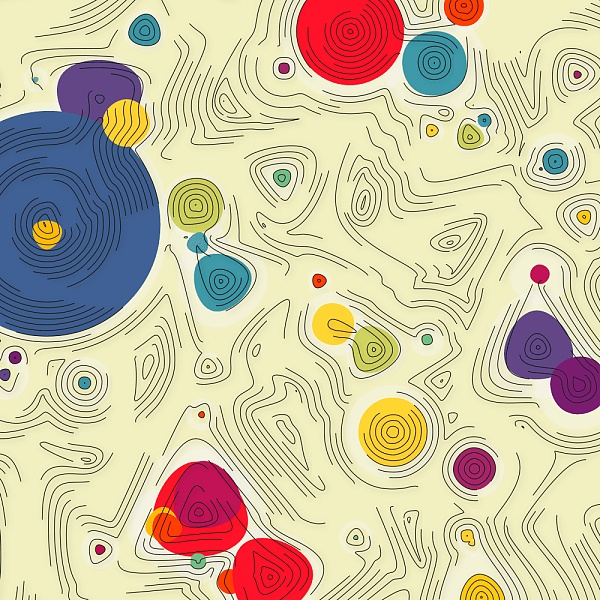
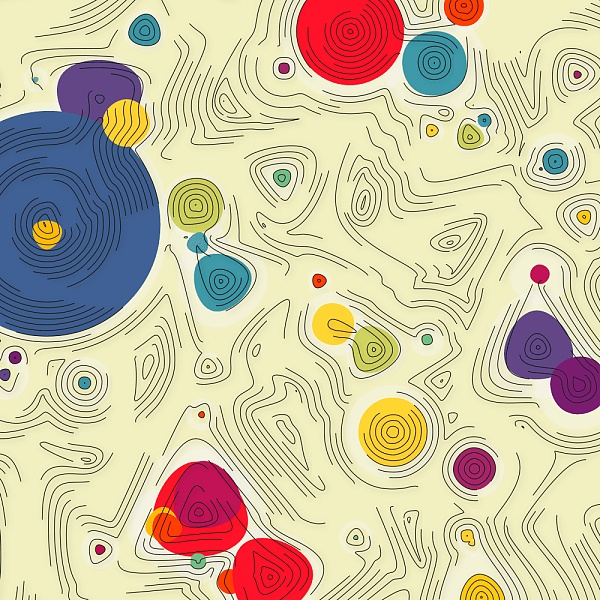
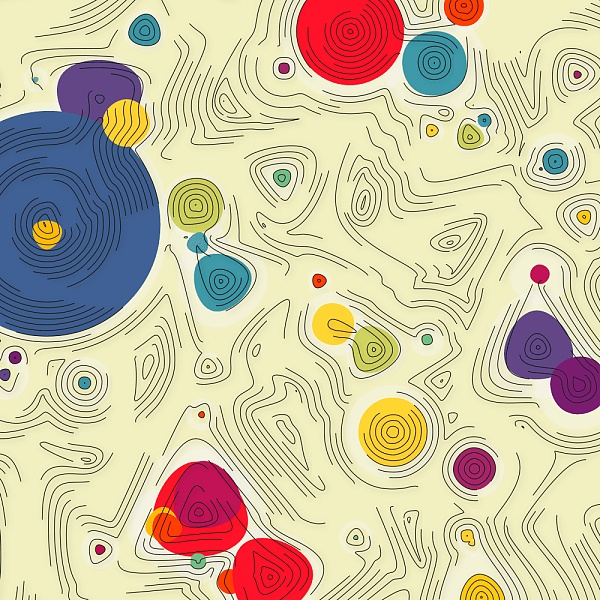
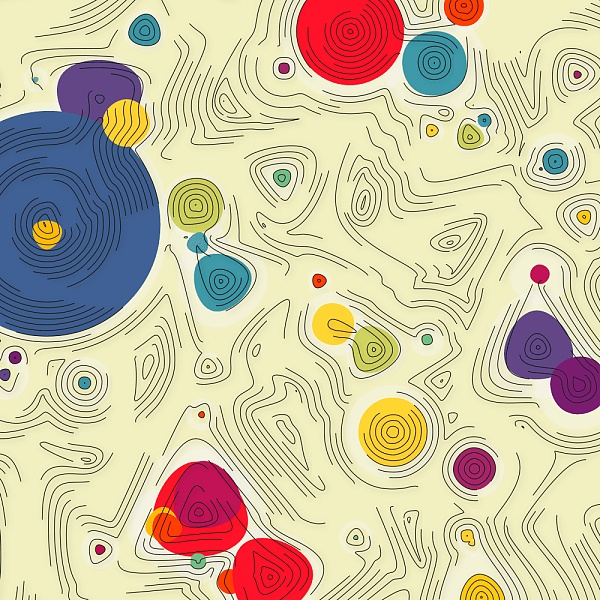
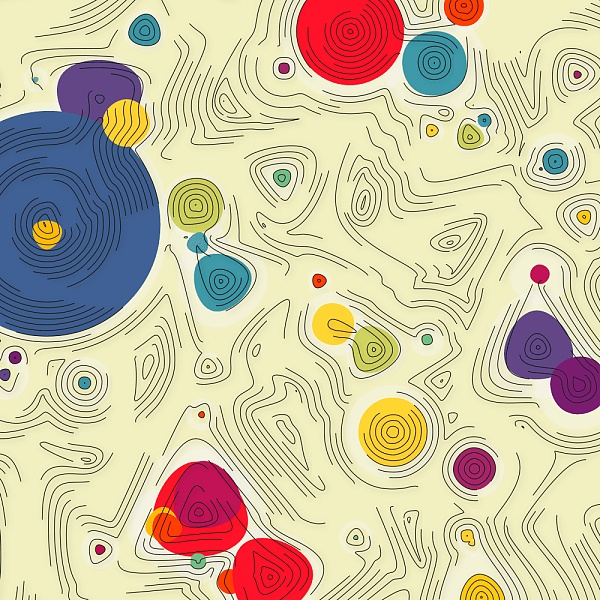
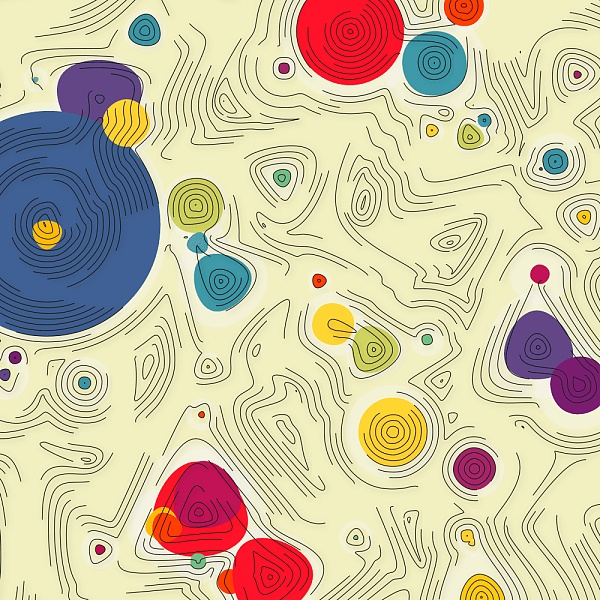
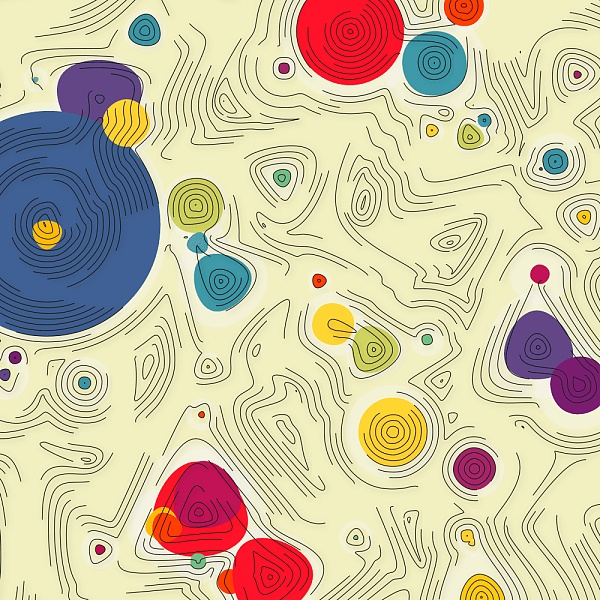
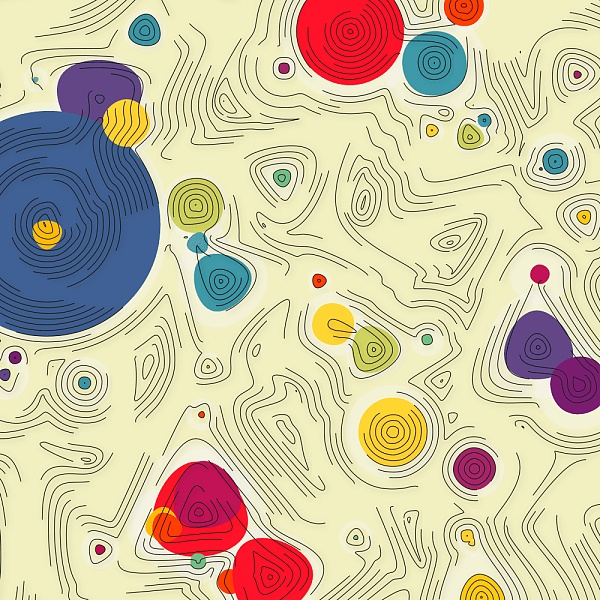
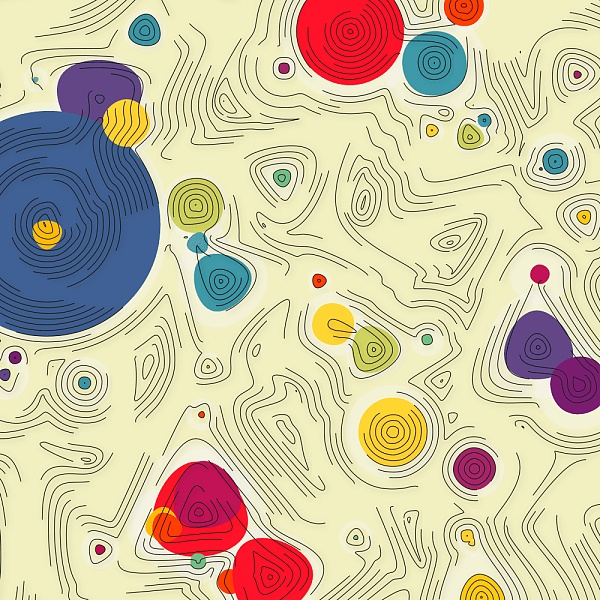
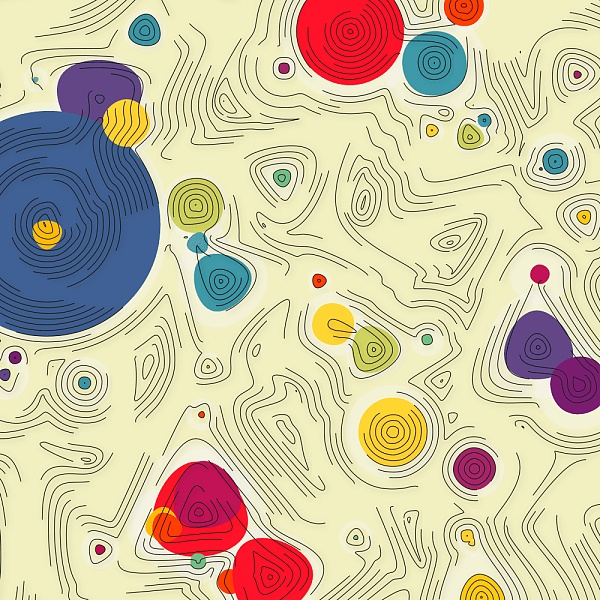
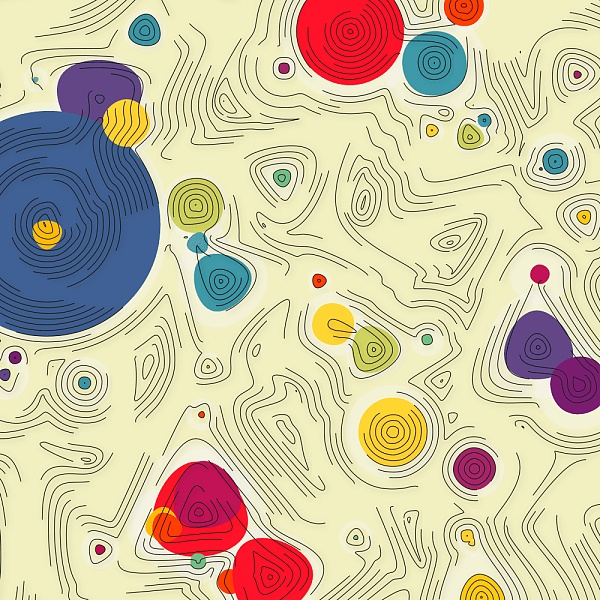
Calabi-Yau anomalies
AI-assisted maths
Unsupervised machine-learning of the Hodge numbers of Calabi-Yau hypersurfaces detects new patterns with an unexpected linear dependence.
Machine learning Calabi-Yau hypersurfaces
We revisit the classic database of weighted -P^4s which admit Calabi-Yau 3-fold hypersurfaces equipped with a diverse set of tools from the machine-learning toolbox. Unsupervised techniques identify an unanticipated almost linear dependence of the topological data on the weights. This then allows us to identify a previously unnoticed clustering in the Calabi-Yau data. Supervised techniques are successful in predicting the topological parameters of the hypersurface from its weights with an accuracy of R^2>95%. Supervised learning also allows us to identify weighted-P^4s which admit Calabi-Yau hypersurfaces to 100% accuracy by making use of partitioning supported by the clustering behavior.