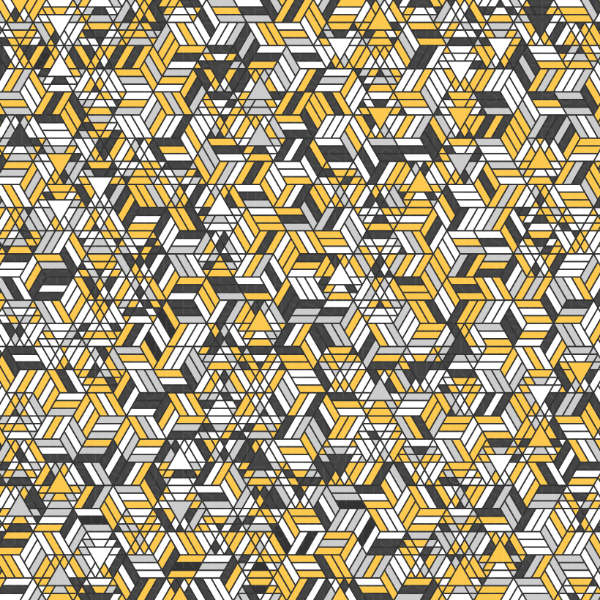
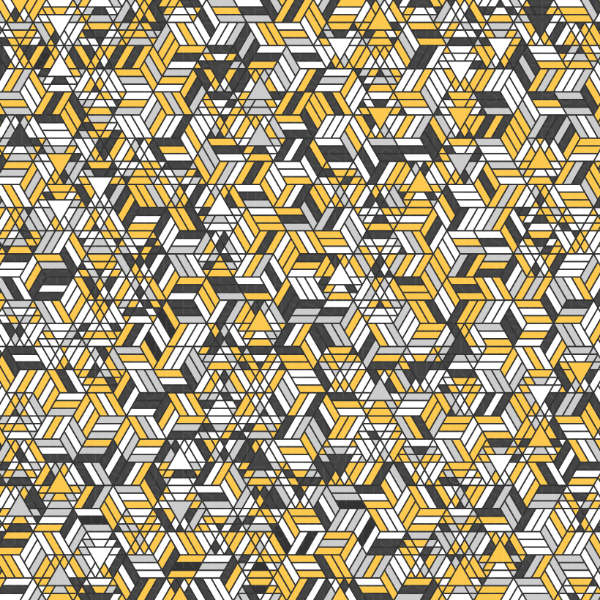
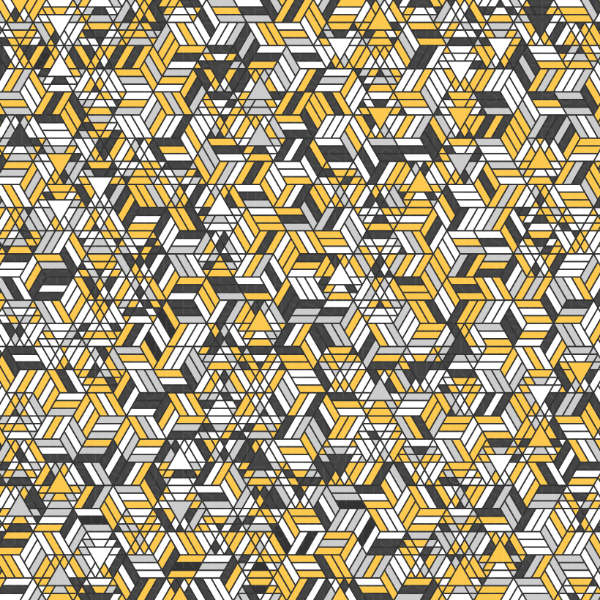
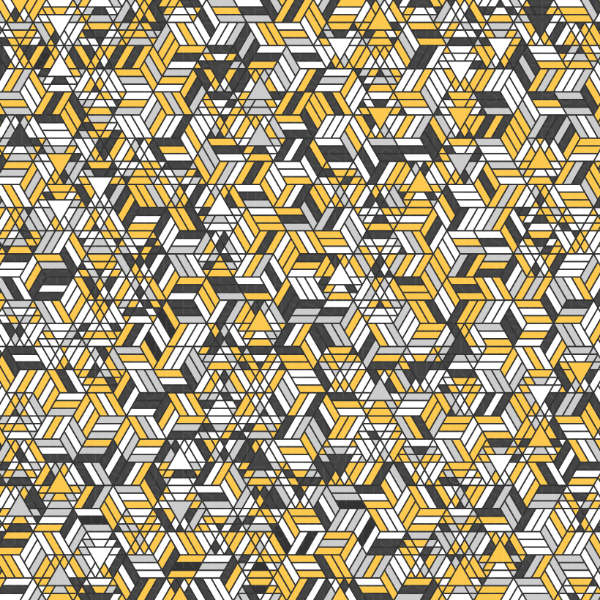
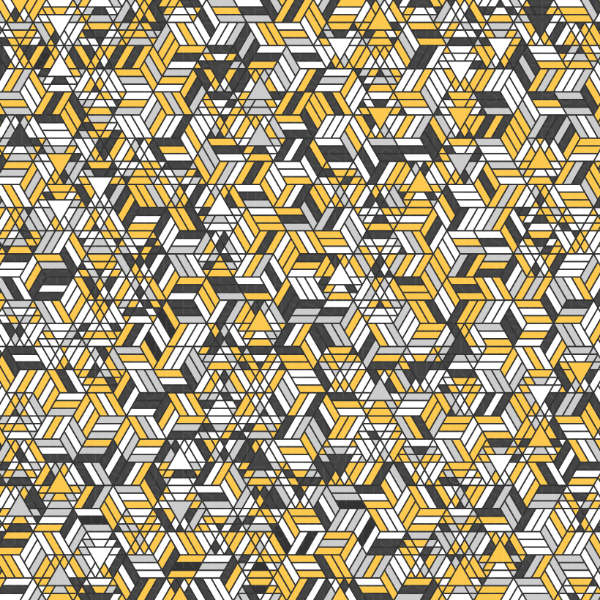
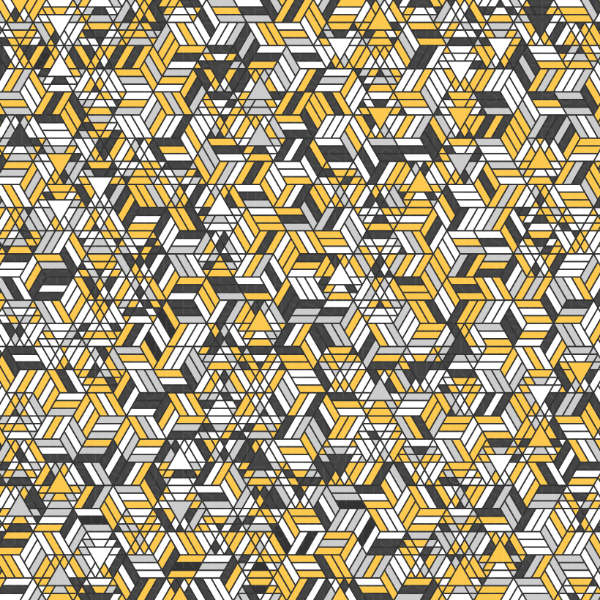
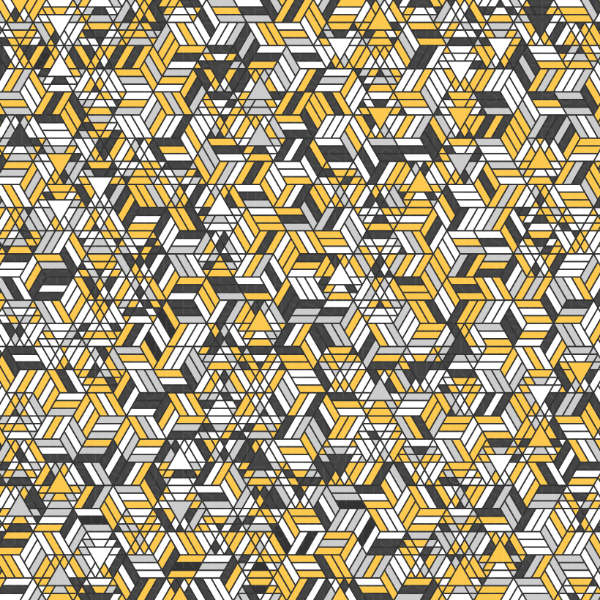
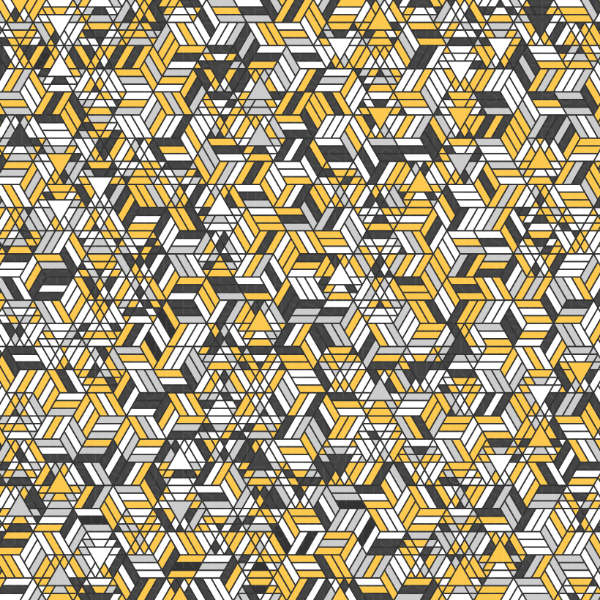
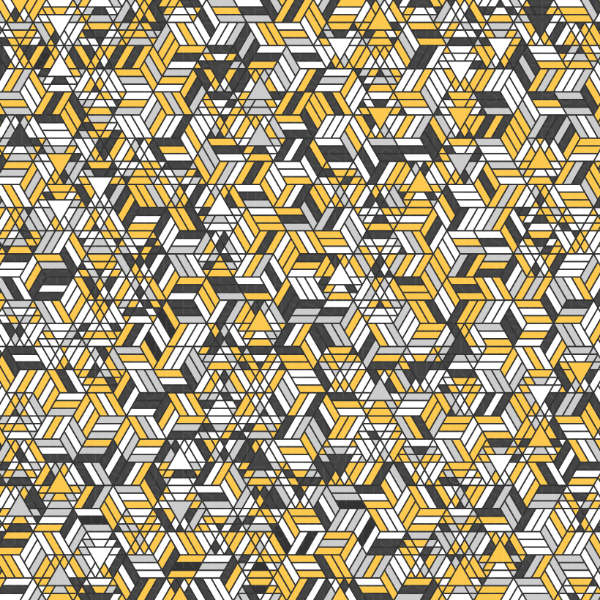
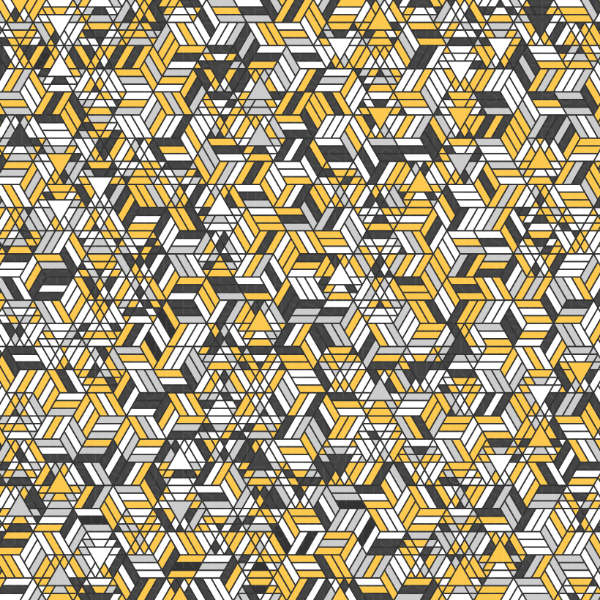
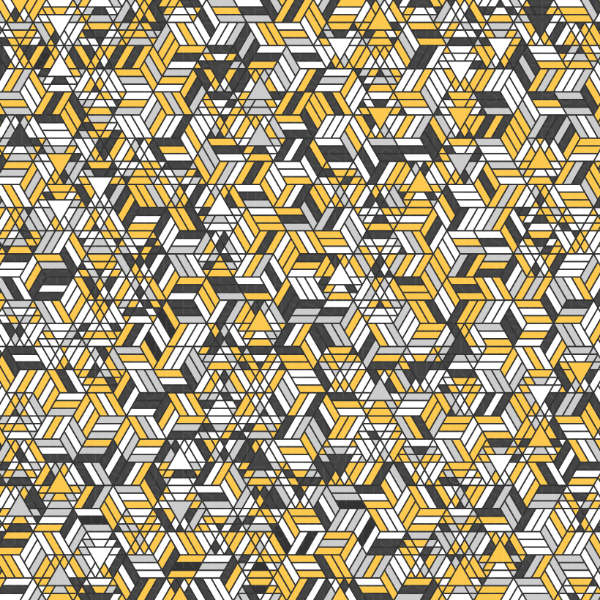
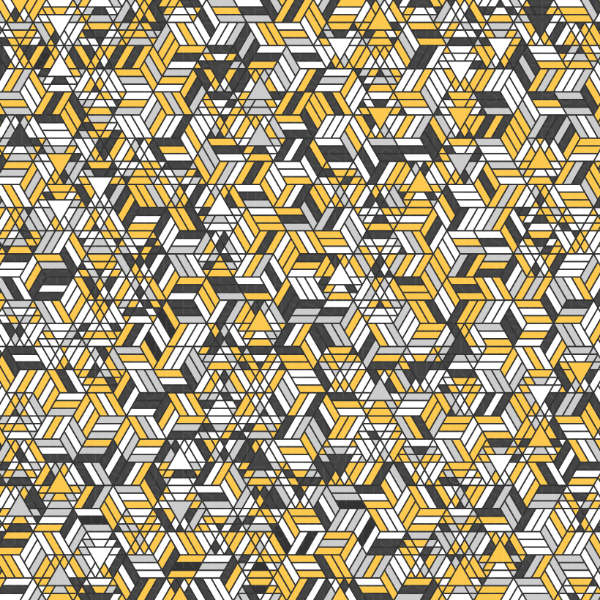
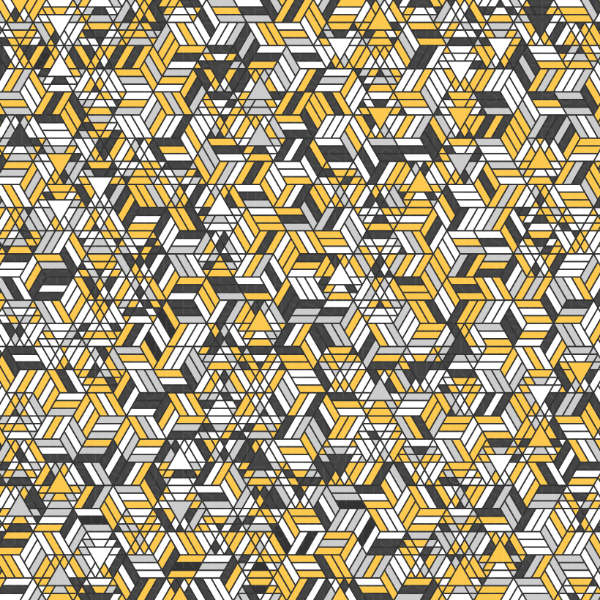
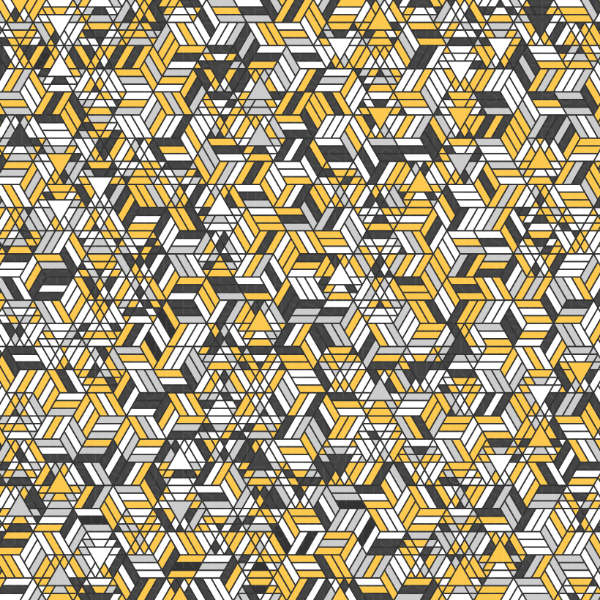
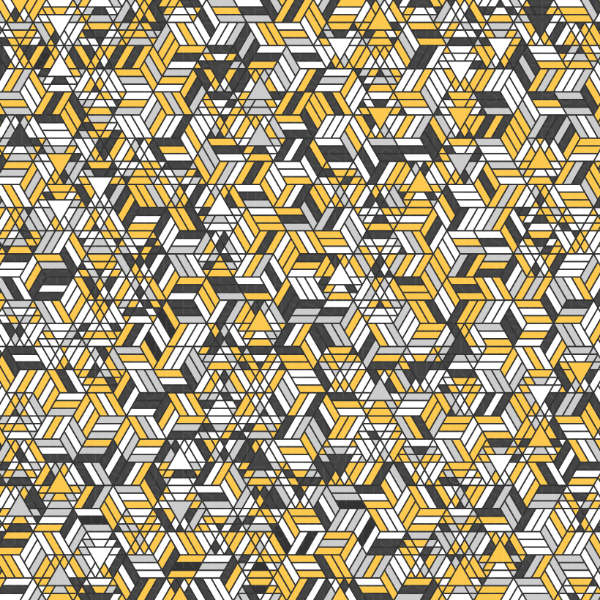
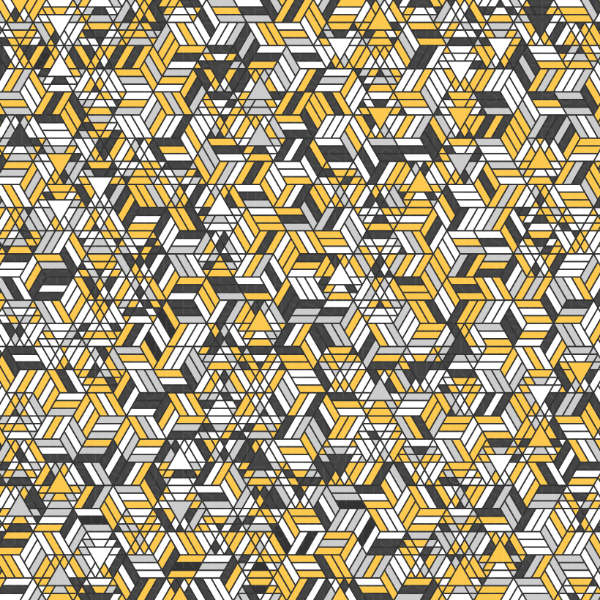
Machine learning Hilbert series
AI-assisted maths
Neural networks find efficient ways to compute the Hilbert series, an important counting function in algebraic geometry and gauge theory.
Hilbert series, machine learning, and applications to physics
We describe how simple machine learning methods successfully predict geometric properties from Hilbert series (HS). Regressors predict embedding weights in projective space to ∼1 mean absolute error, whilst classifiers predict dimension and Gorenstein index to >90% accuracy with 0.5% standard error. Binary random forest classifiers managed to distinguish whether the underlying HS describes a complete intersection with high accuracies exceeding 95%. Neural networks (NNs) exhibited success identifying HS from a Gorenstein ring to the same order of accuracy, whilst generation of “fake” HS proved trivial for NNs to distinguish from those associated to the three-dimensional Fano varieties considered.