Disentangling group and link persistence in Dynamic Stochastic Block models
Inference from single snapshots of temporal networks can misleadingly group communities if the links between snapshots are correlated.
Journal of Statistical Mechanics 2018, 12 (2017)
P. Barucca, F. Lillo, P. Mazzarisi, D. Tantari
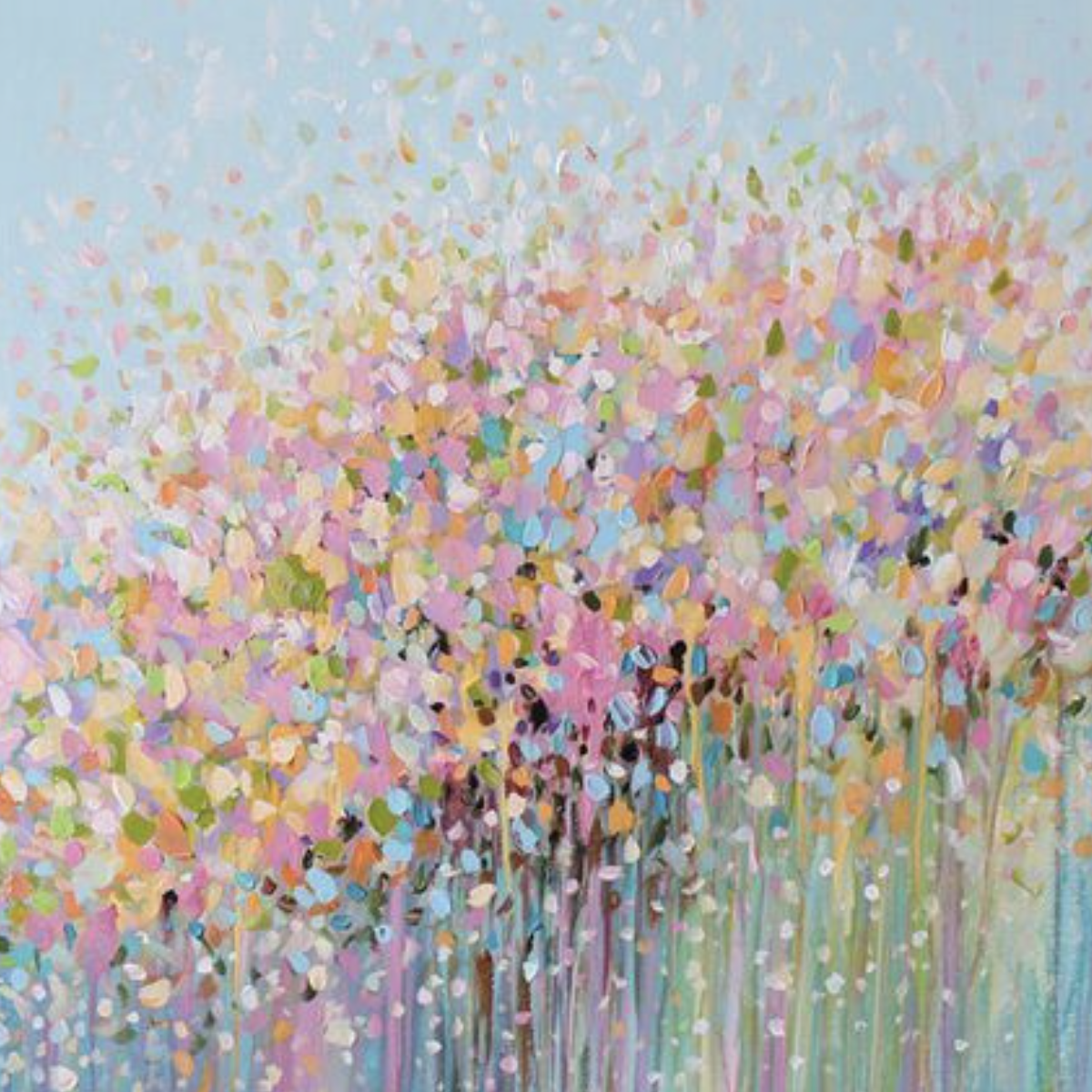
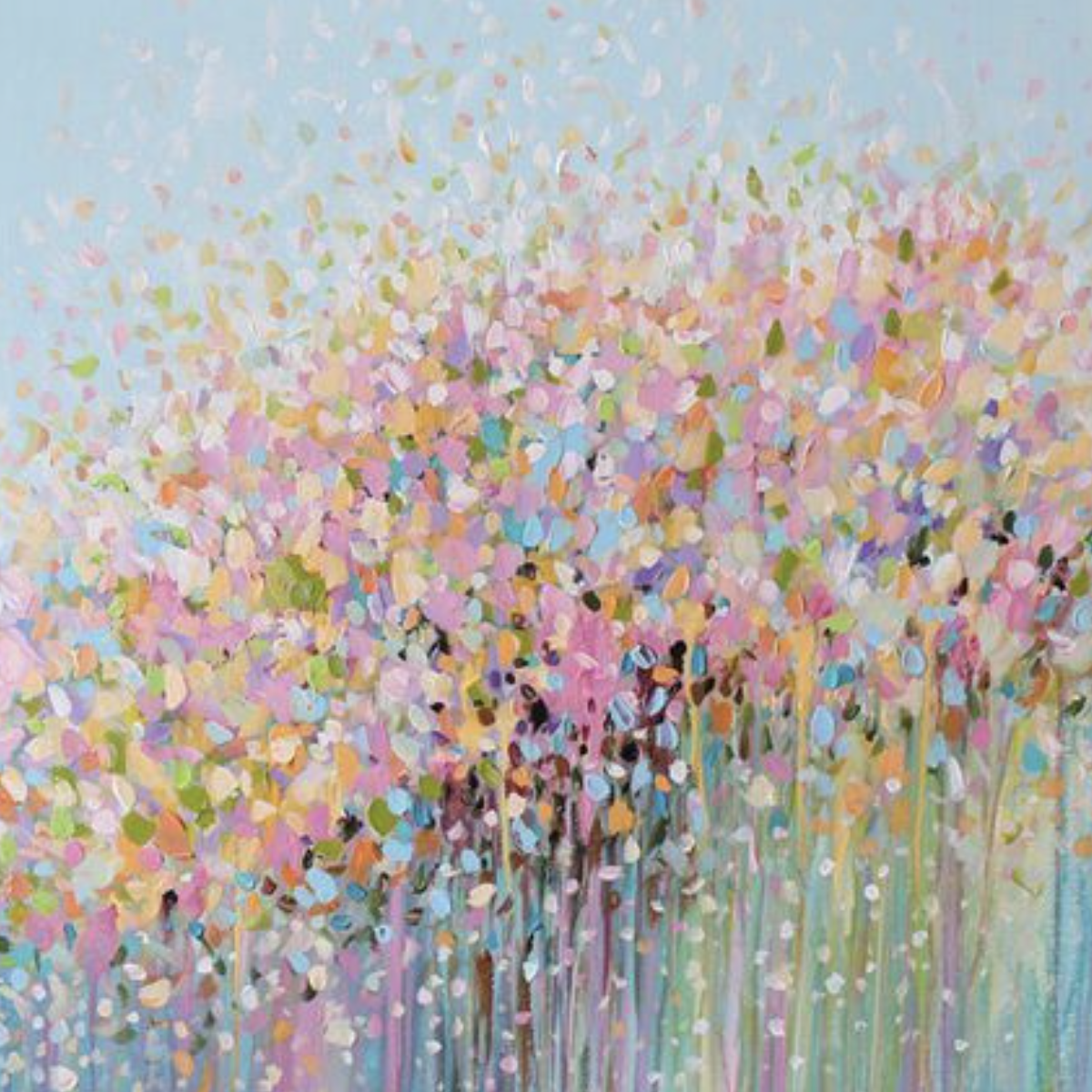
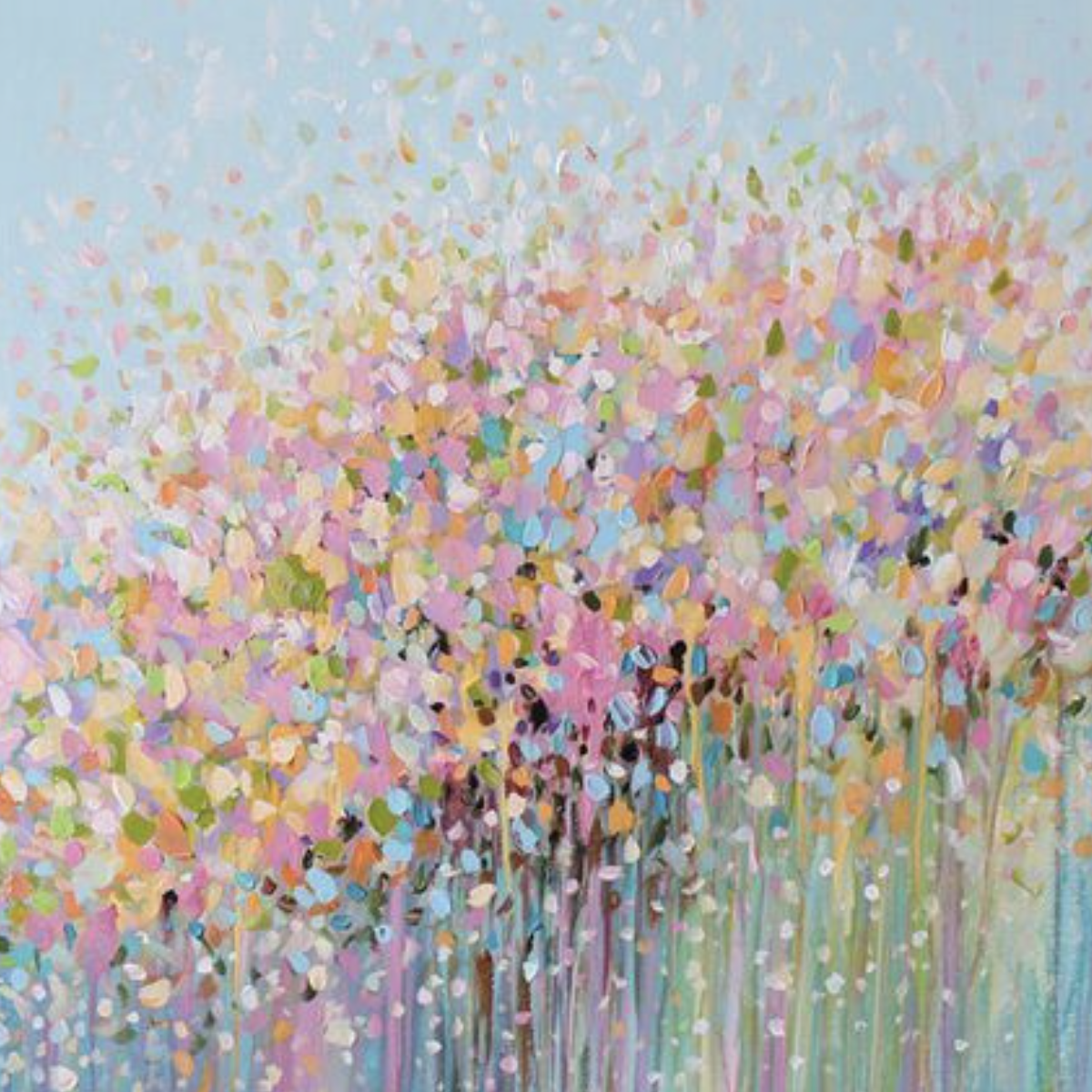
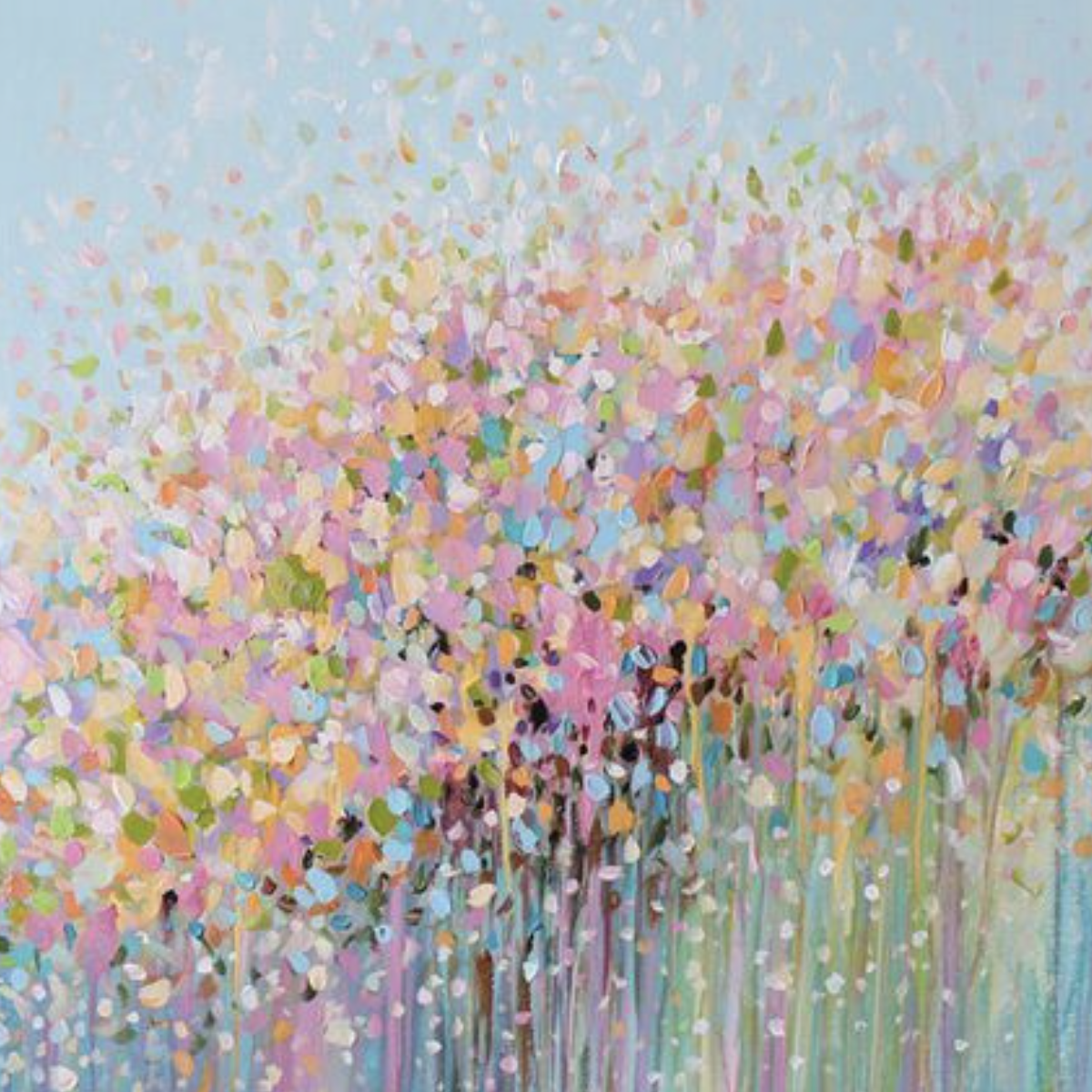
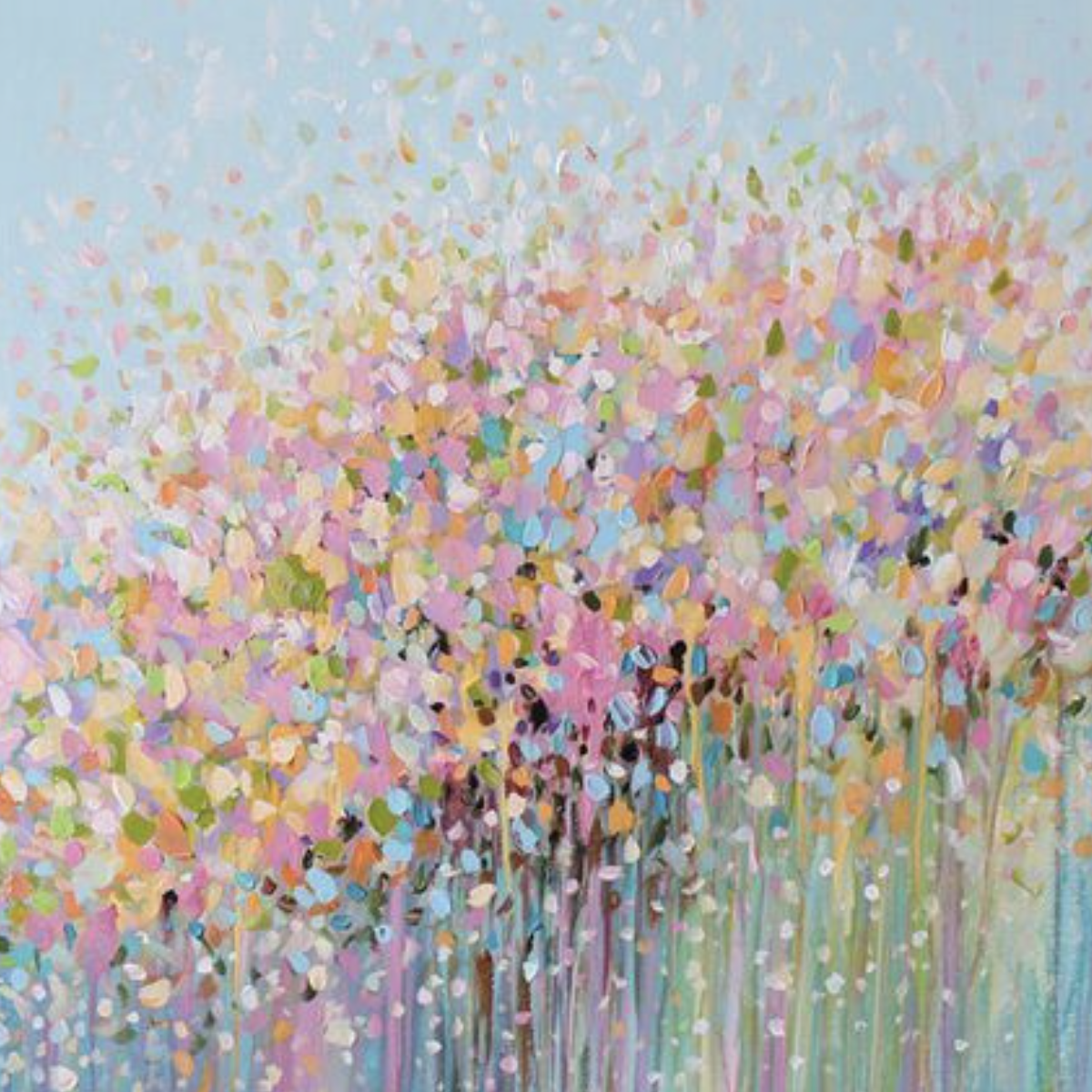
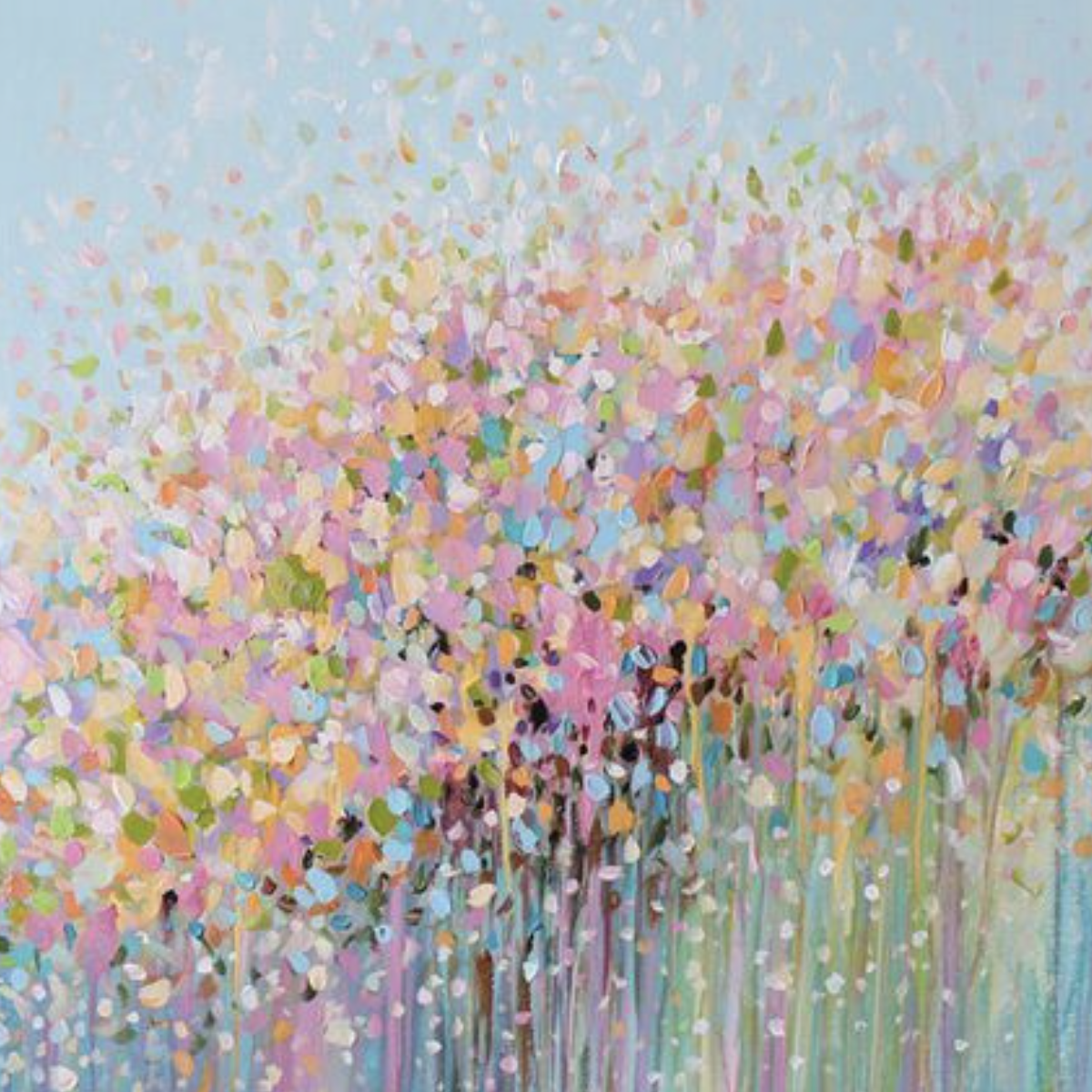
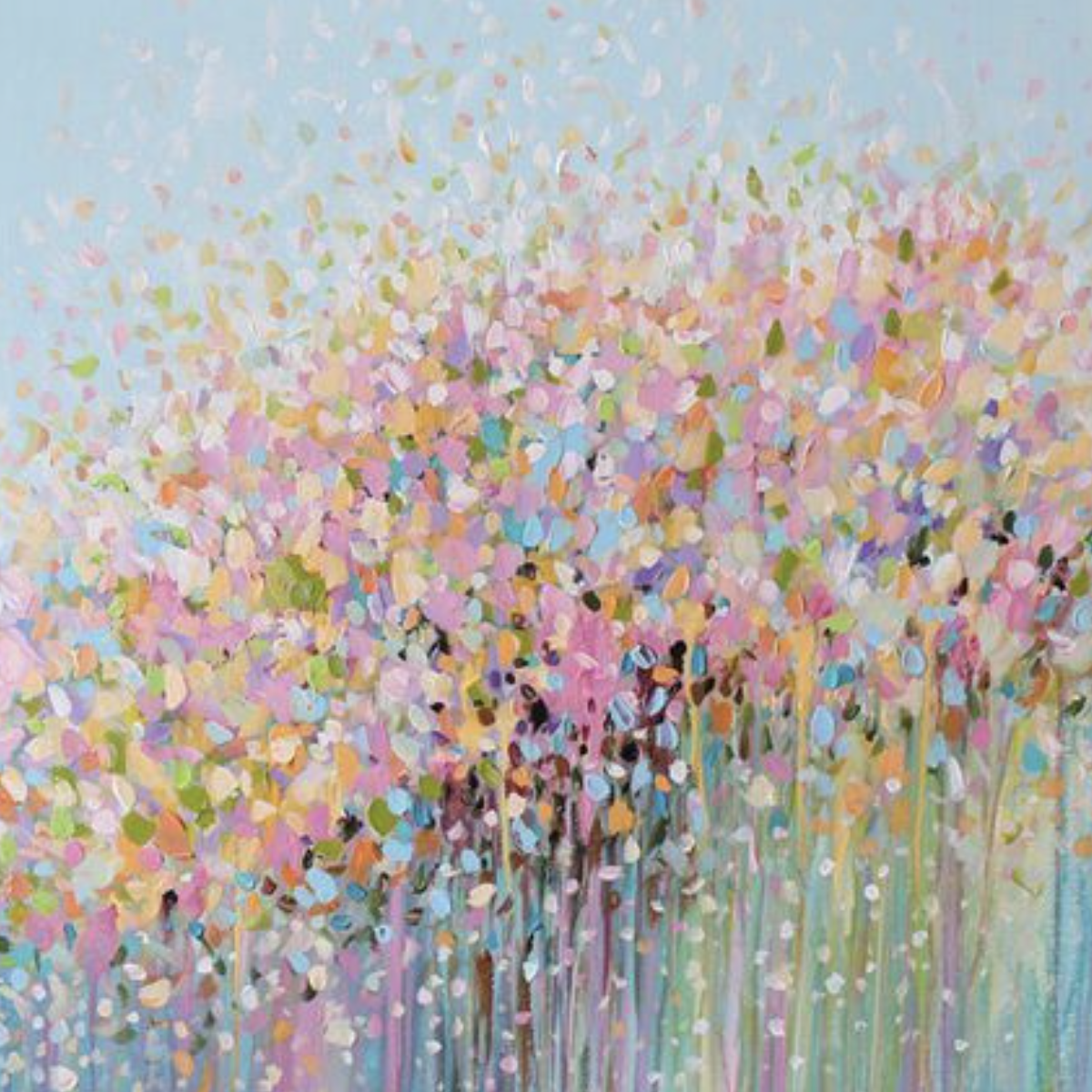
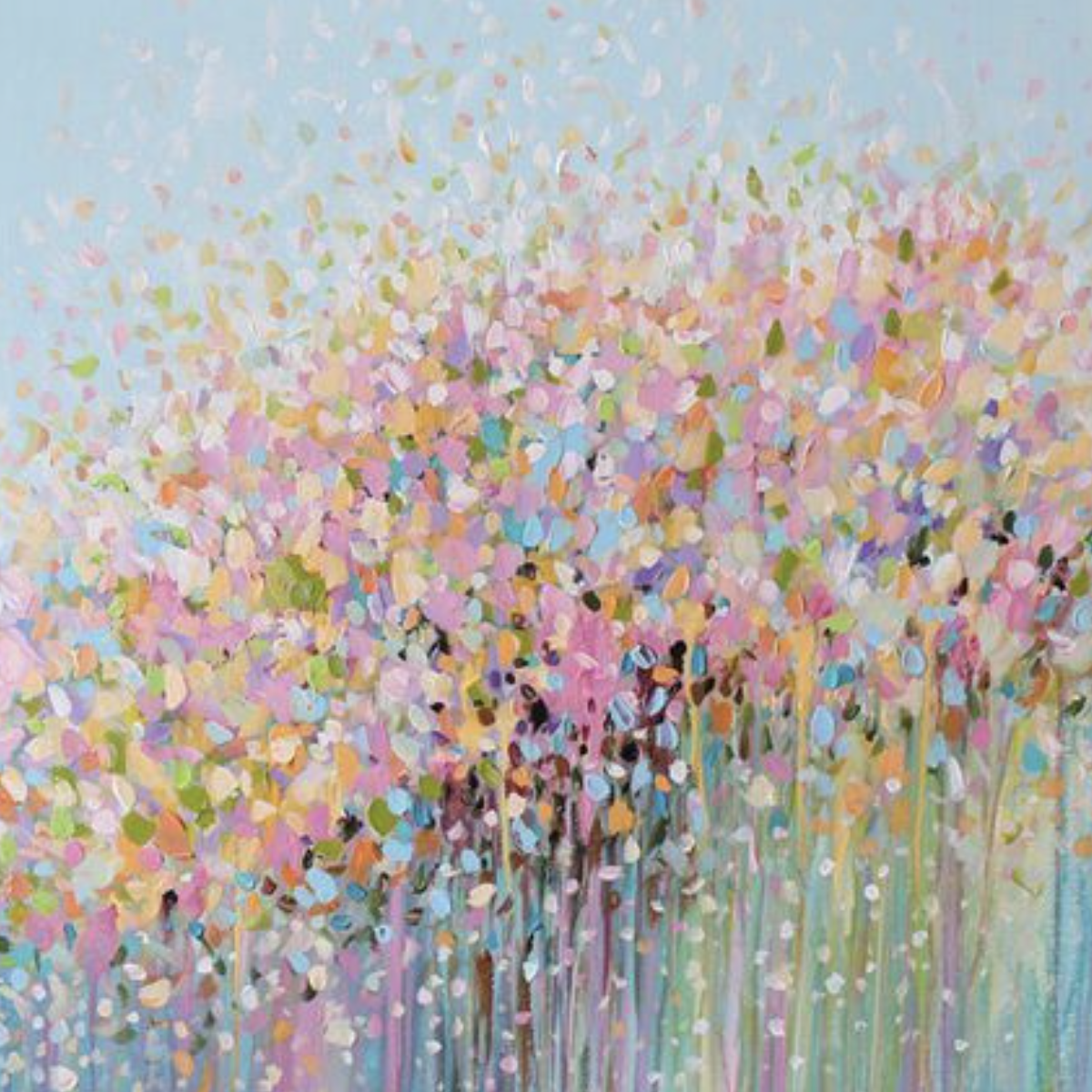
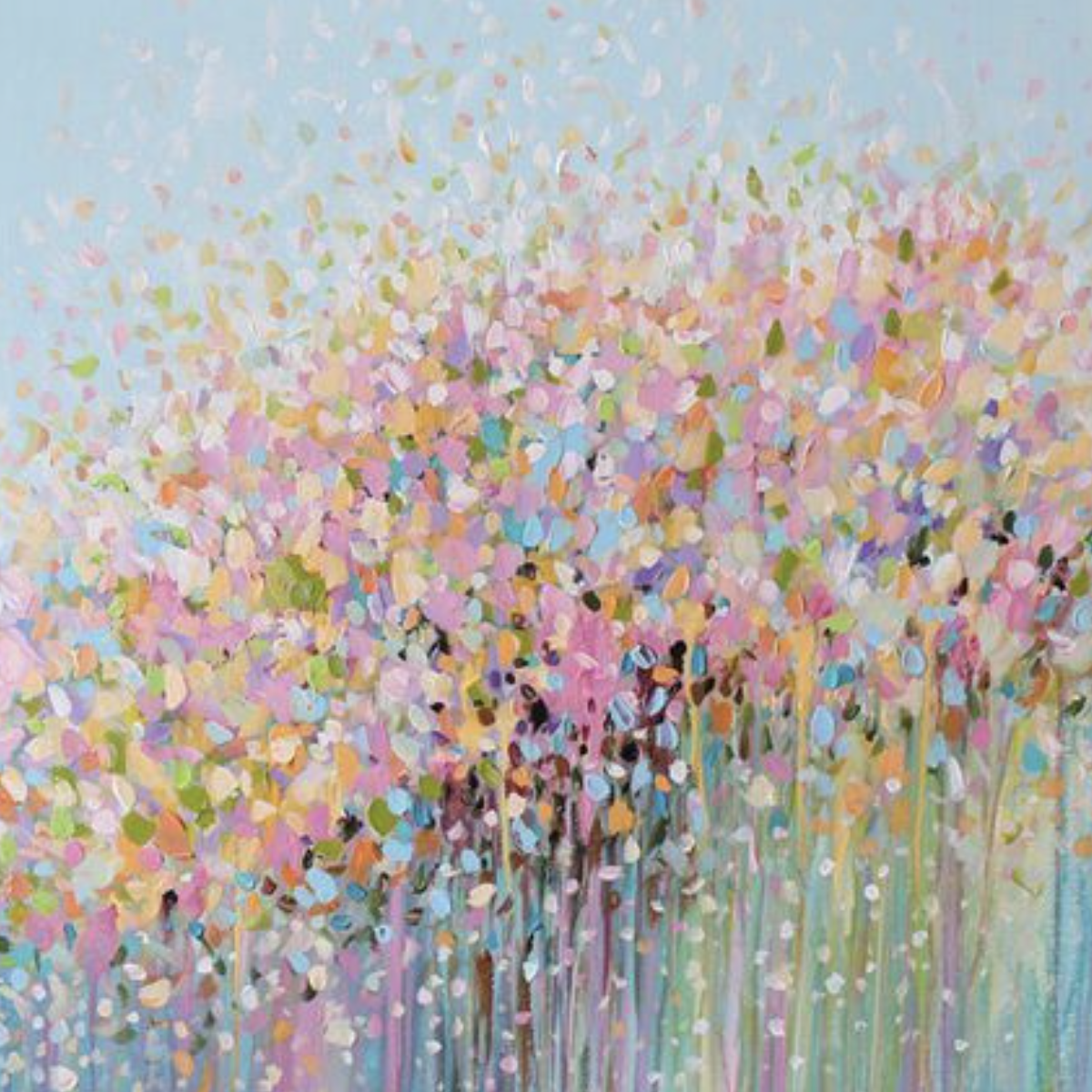
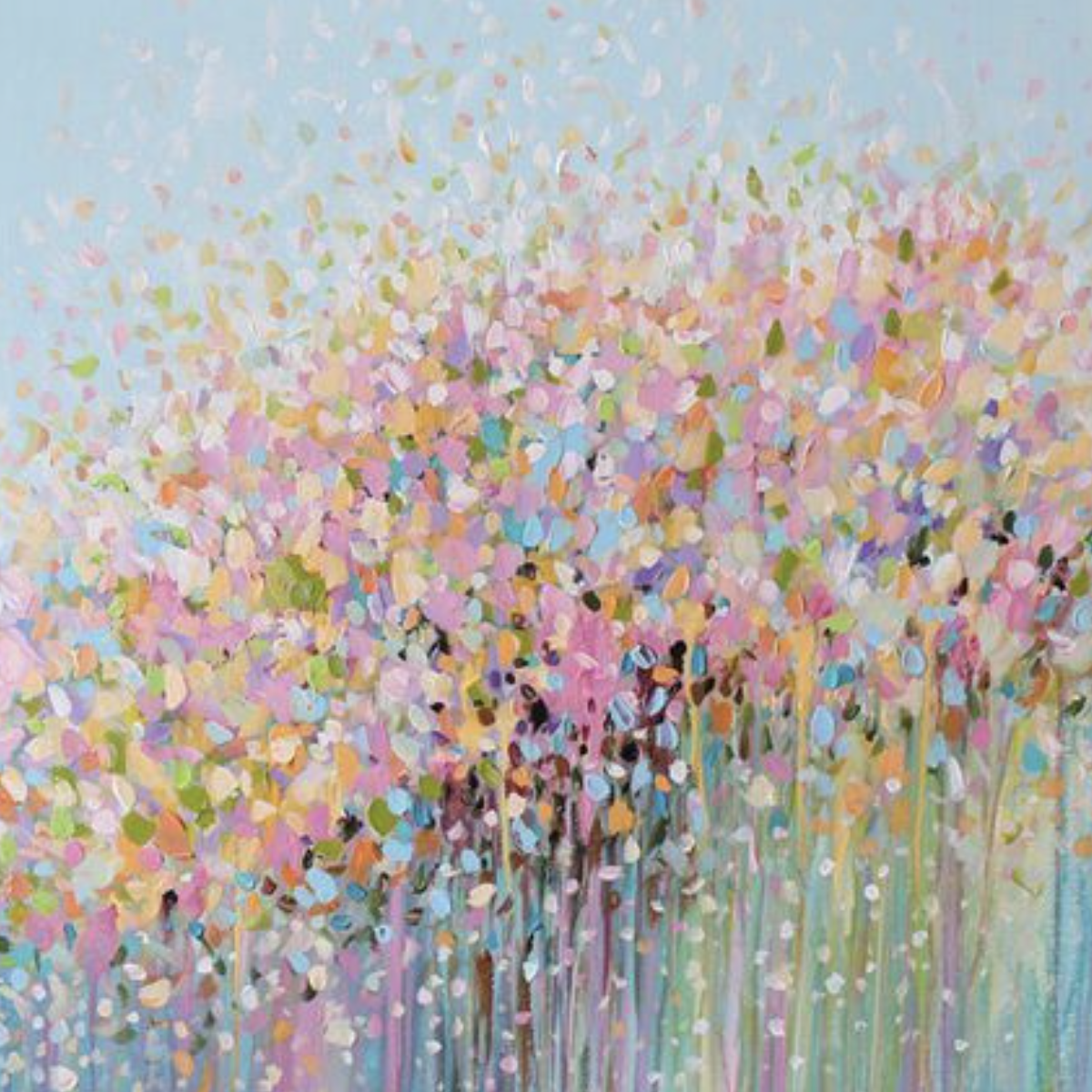
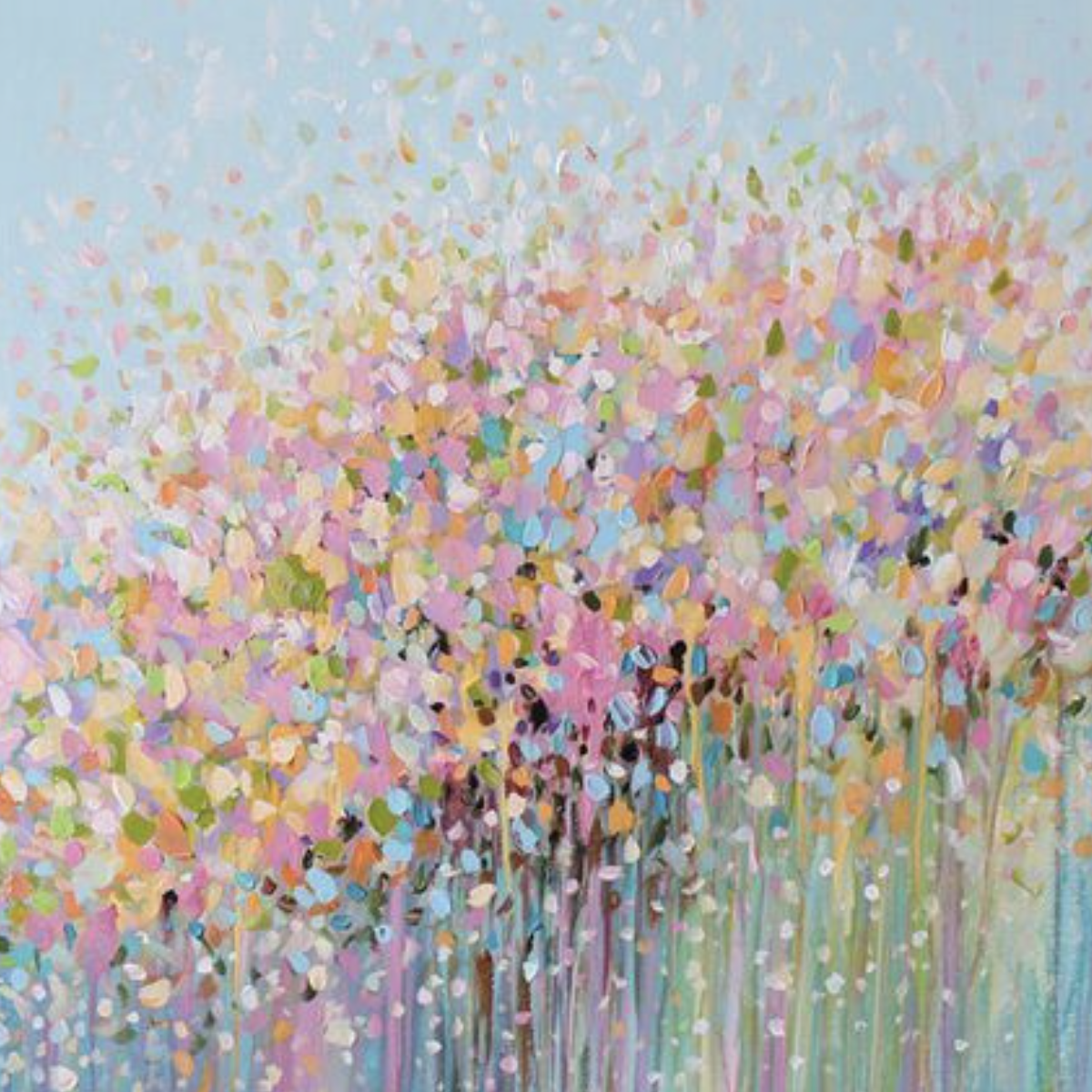
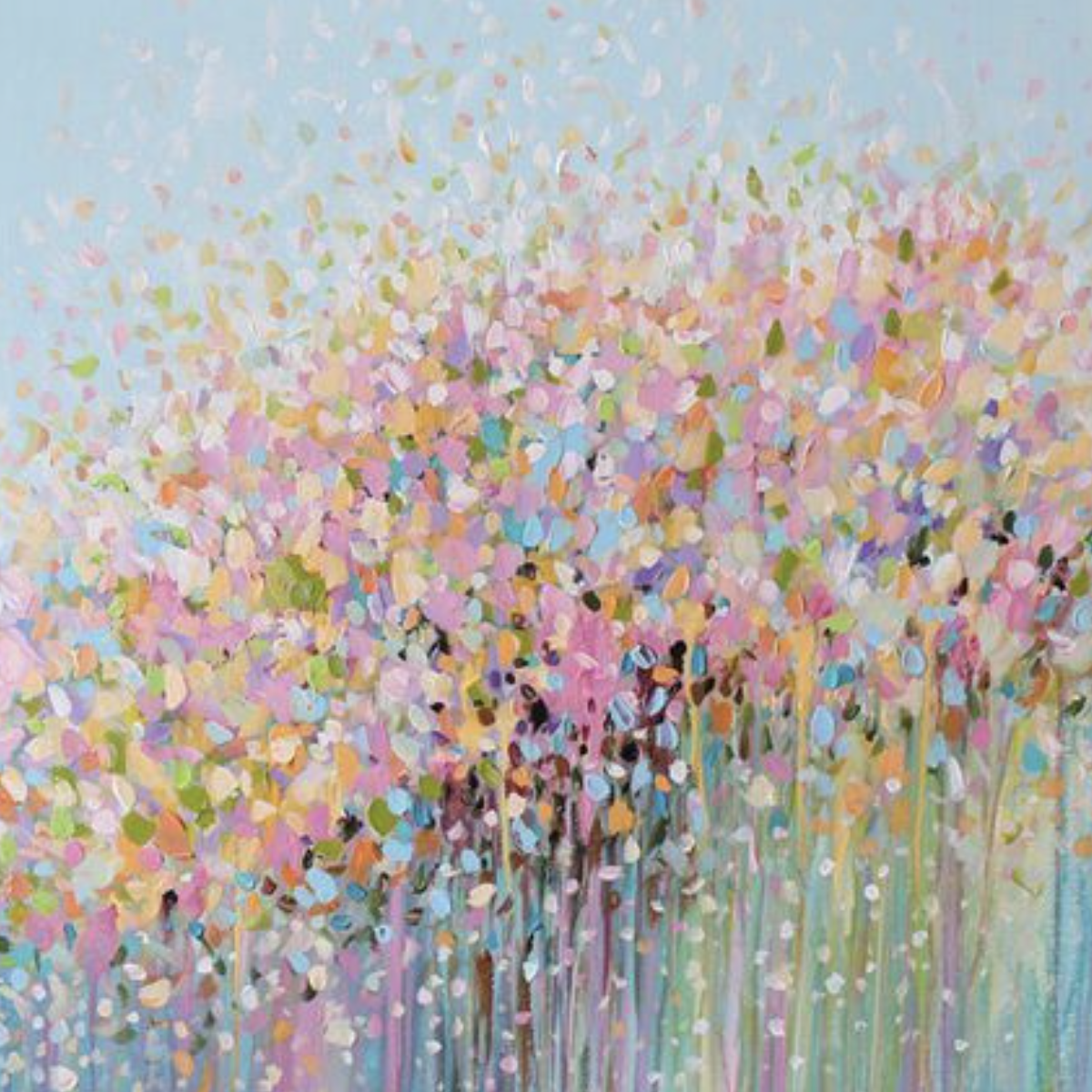
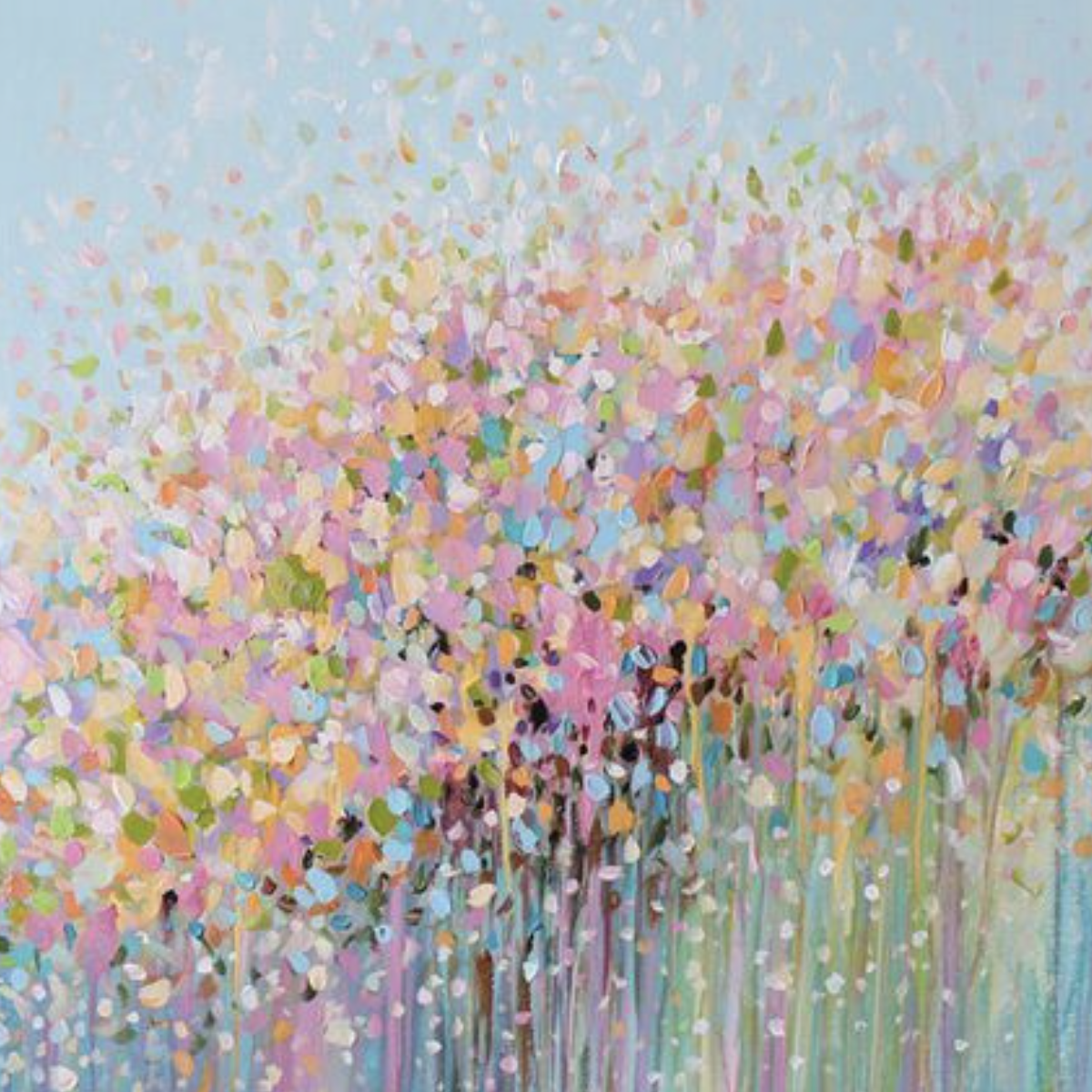
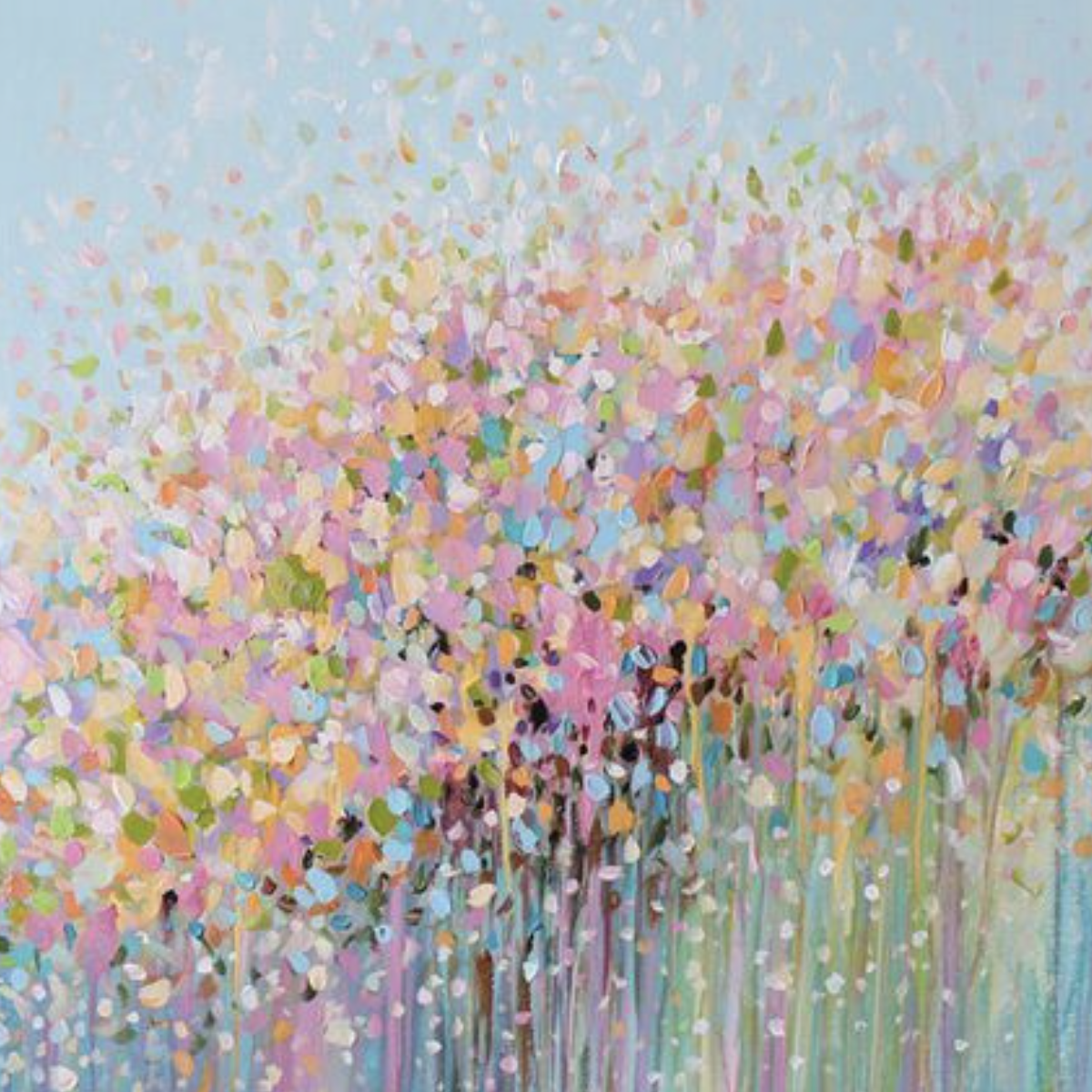
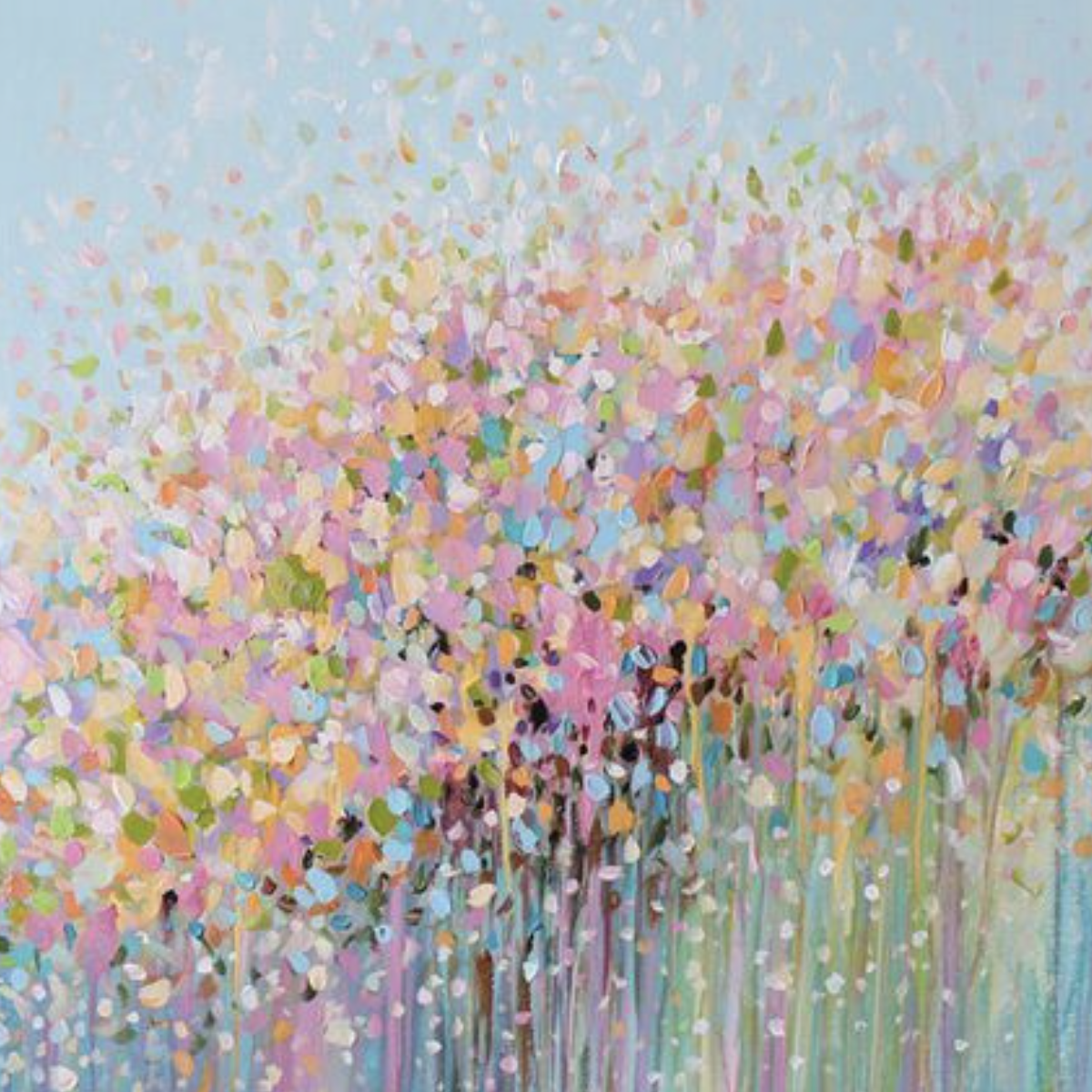
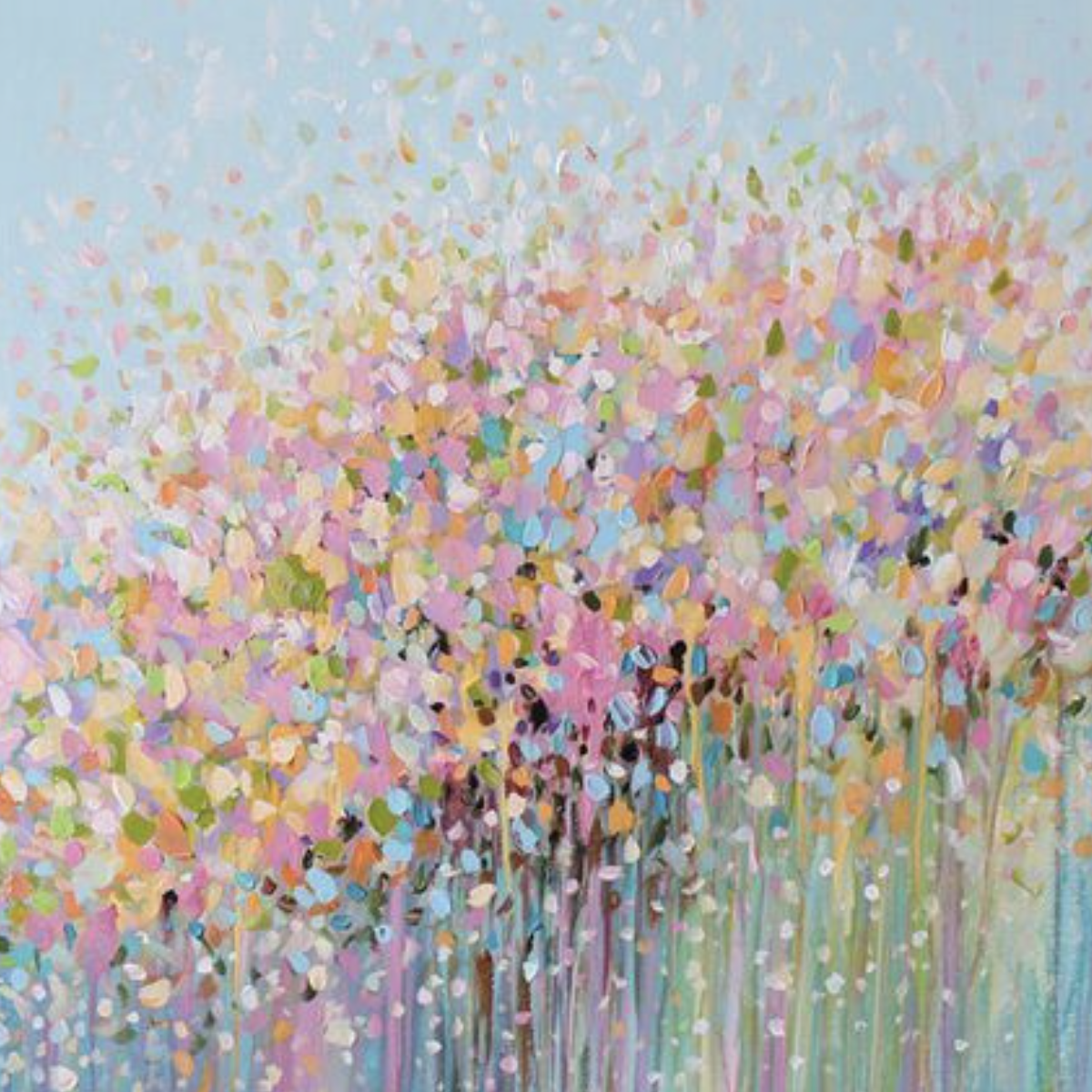
We study the inference of a model of temporal networks in which both communities and links keep memory of previous network state. By considering maximum likelihood inference from single snapshot observation of the network, we show that link persistence decreases the detectability threshold, preventing the inference of communities even when they are in principle strong enough, while community persistence tends to restore this possibility. Then we show that the inferred communities share a maximum overlap with those of a specific previous instant of time, corresponding to the maximum of a time-lagged assortativity parameter, and therefore they can be closer to those of the past than of the present. We analytically characterize these transitions as a function of the memory parameters of the model.