Machine learning the regulatory structure of cell states
Building machine learning models that mimic the behaviour of cells in silico to improve the prediction of genes for cell programming.
A complete understanding of gene regulatory networks would allow us to identify the subsets of genes that program specific cell types, saving resources and time. While a complete understanding is still far off, machine learning techniques are enabling us to build black box models that mimic the behaviour of cells. By simulating a cell’s response to different gene combinations, we can disentangle genes that cause programming from those that are a consequence of programming.
In this project we combine autoencoder techniques with inference methods to build low-dimensional models of cell dynamics. This allows us to fuse multiple sequencing measurements into a single low-dimensional cell state space. In this space, we infer a dynamical model which allows us to simulate how cells transition from one state to another. By examining the response of this model to different combinations of genes, we could deduce the combinations that program new types.
The structure of the low-dimensional cell state space can lead to new insights into cell differentiation and disease. By using this to improve the prediction of genes, we can accelerate the production of new programmed cell types, reducing the cost of drug discovery, cell therapies and basic research.
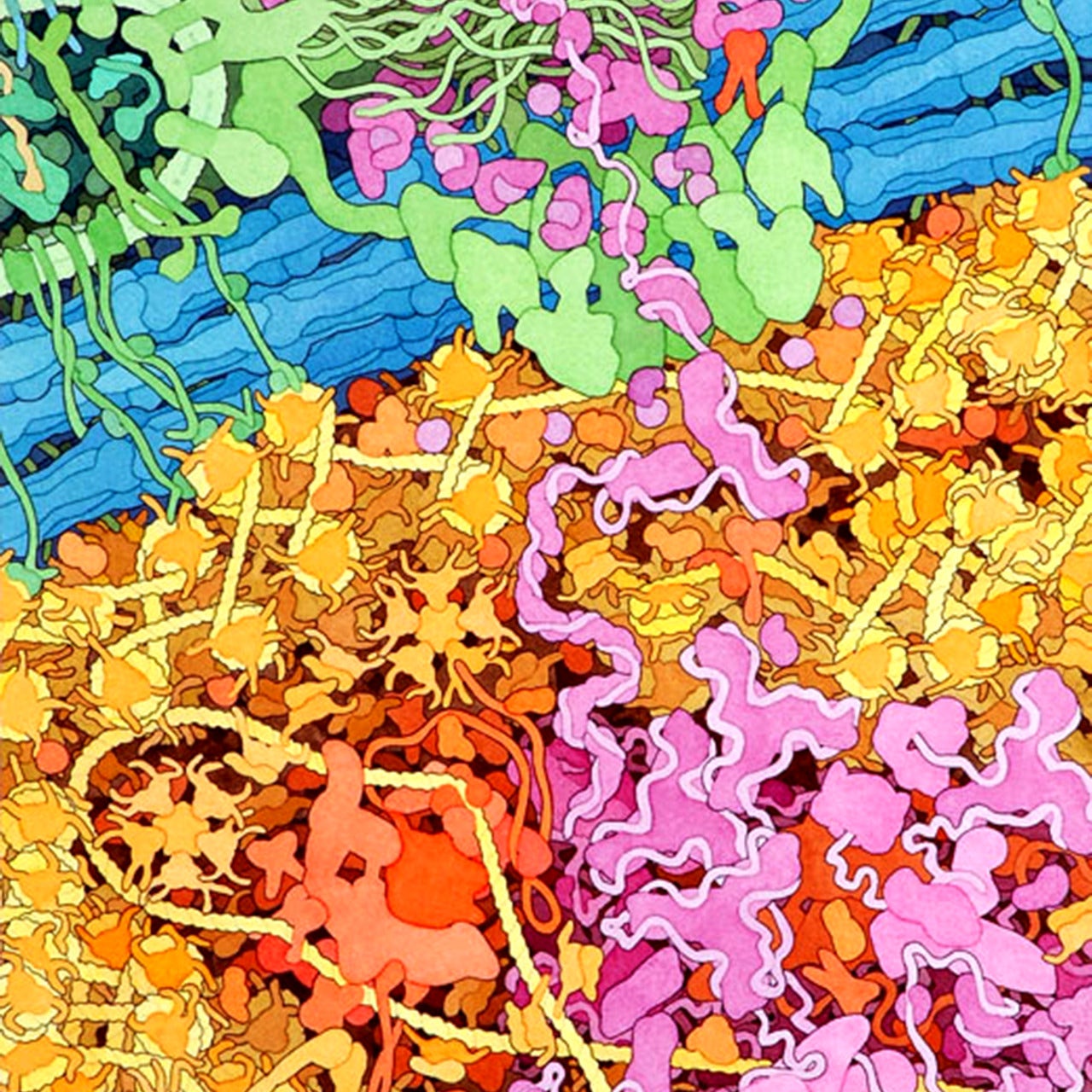
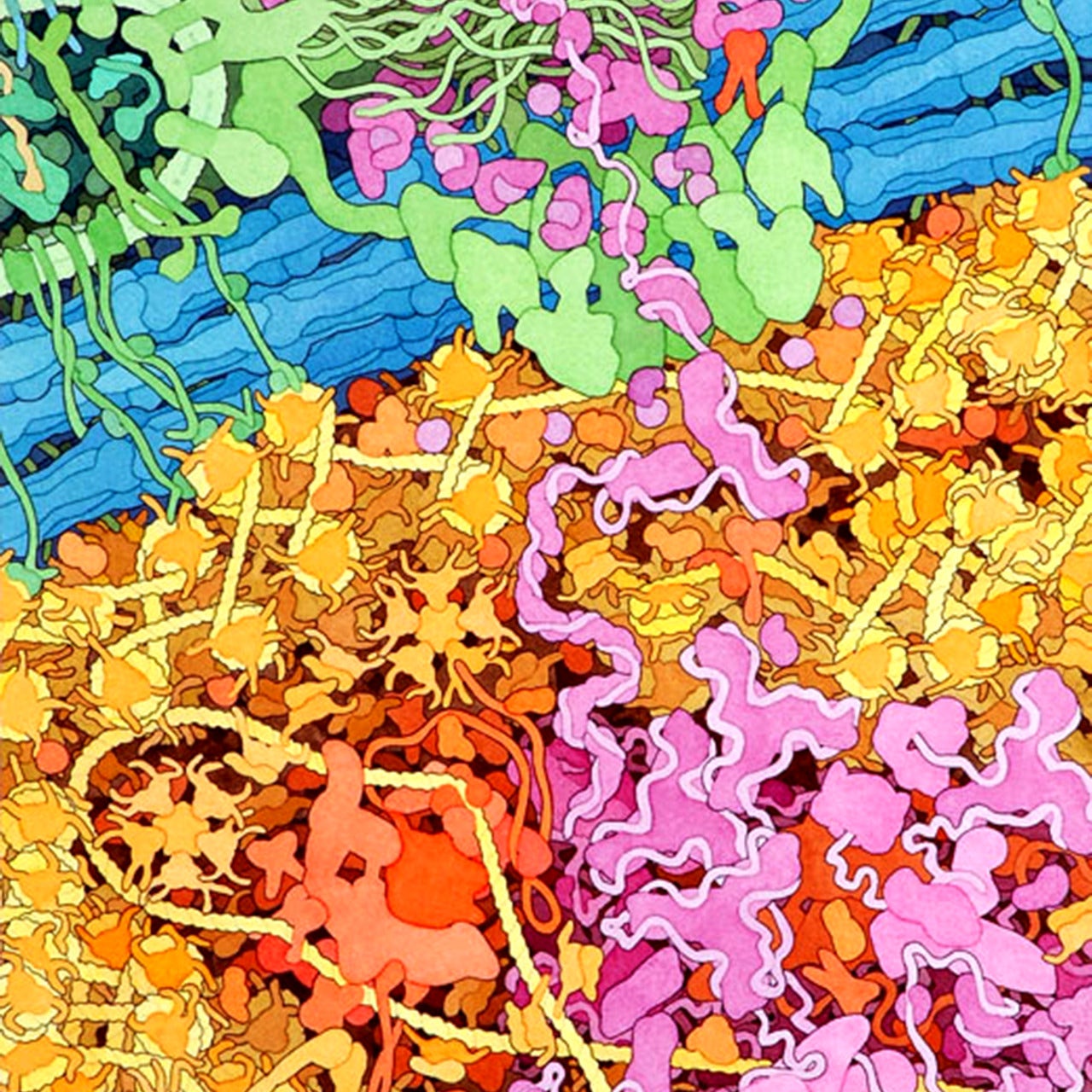
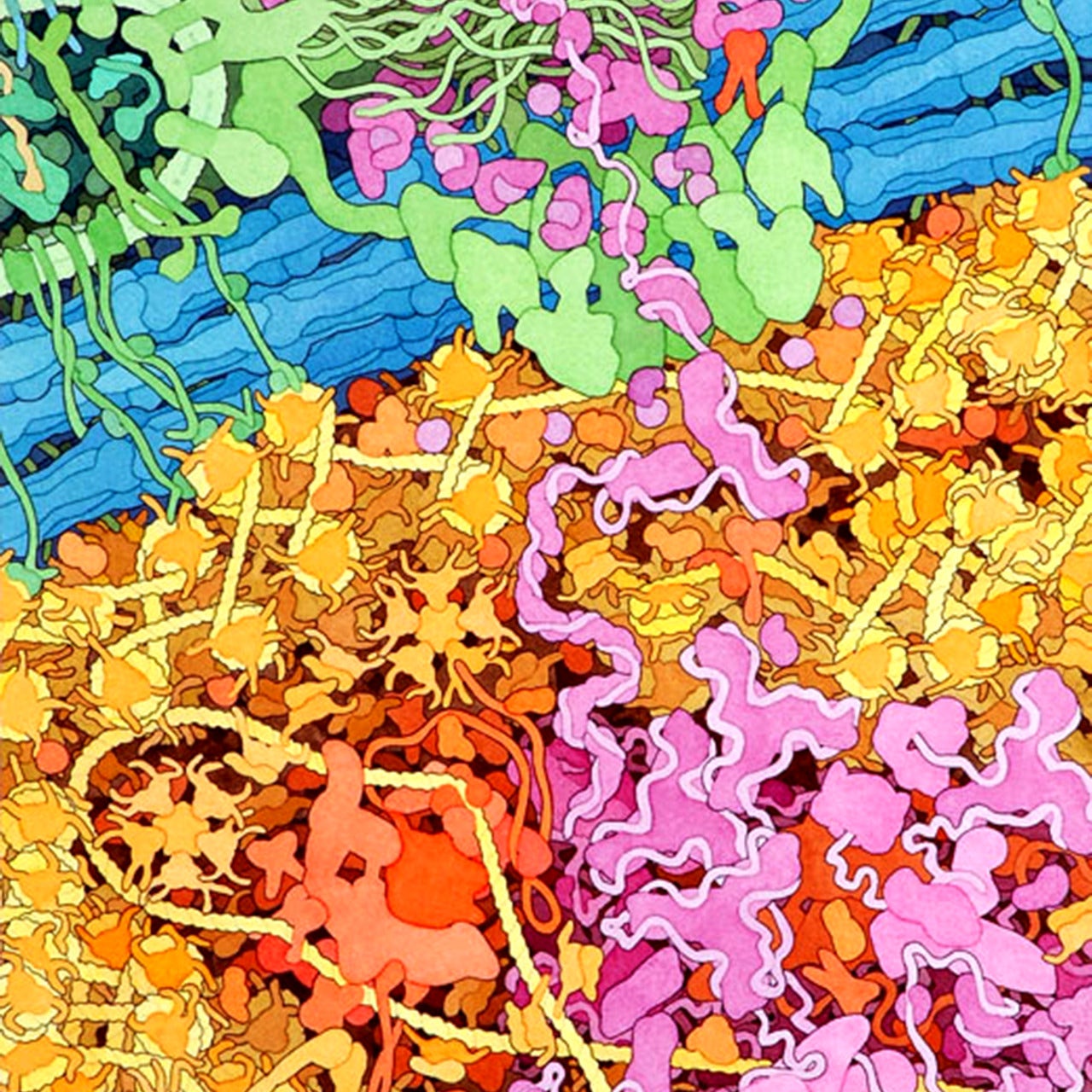
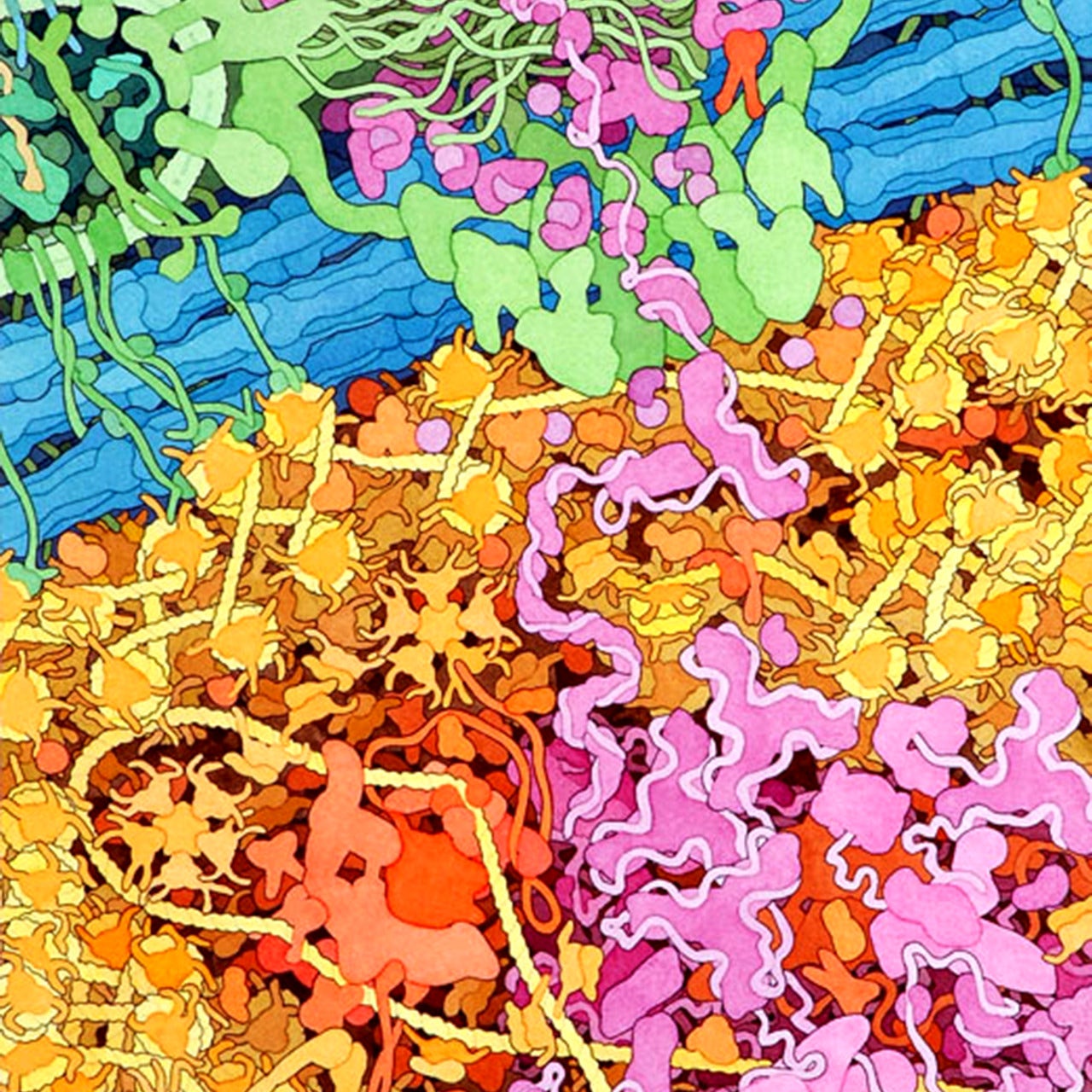
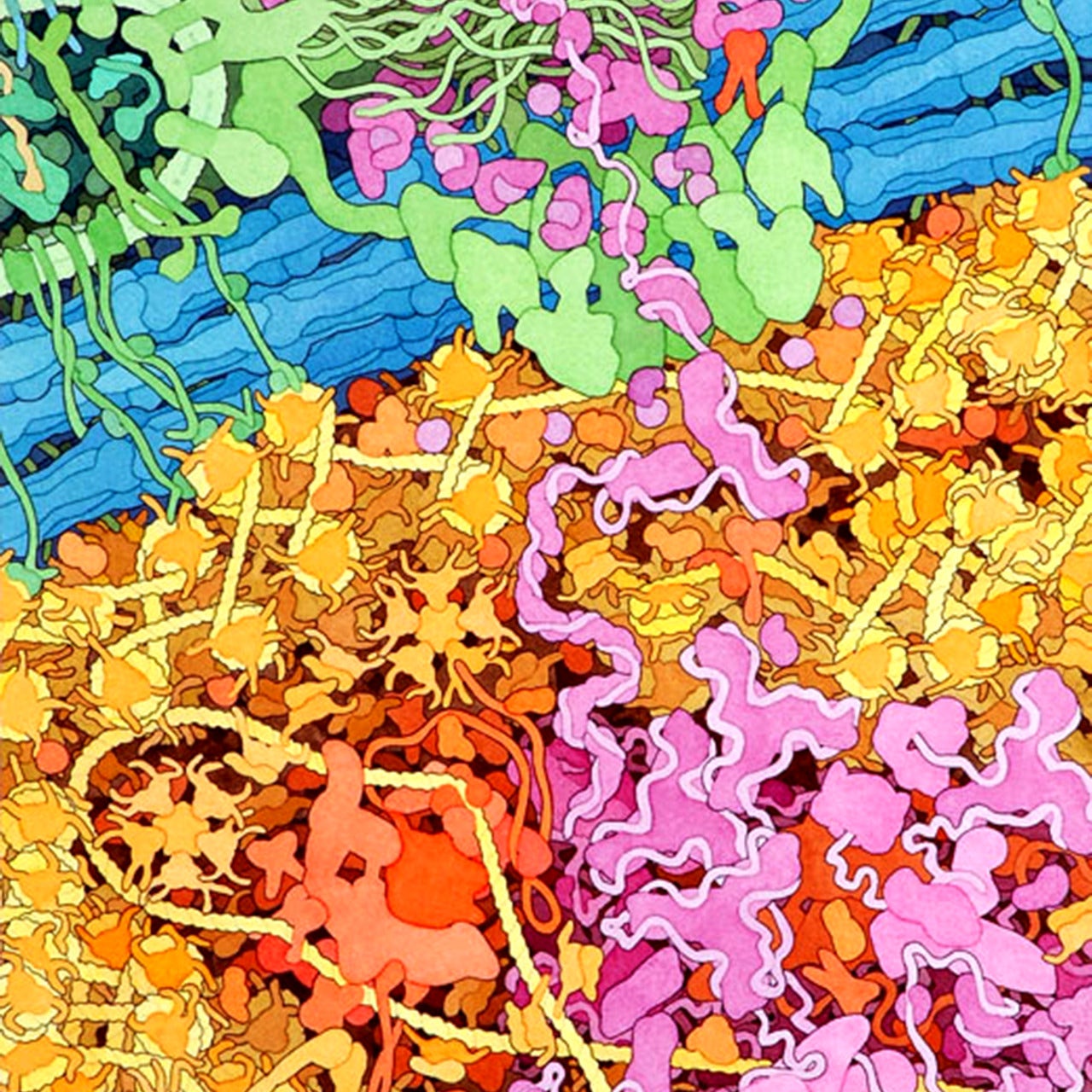
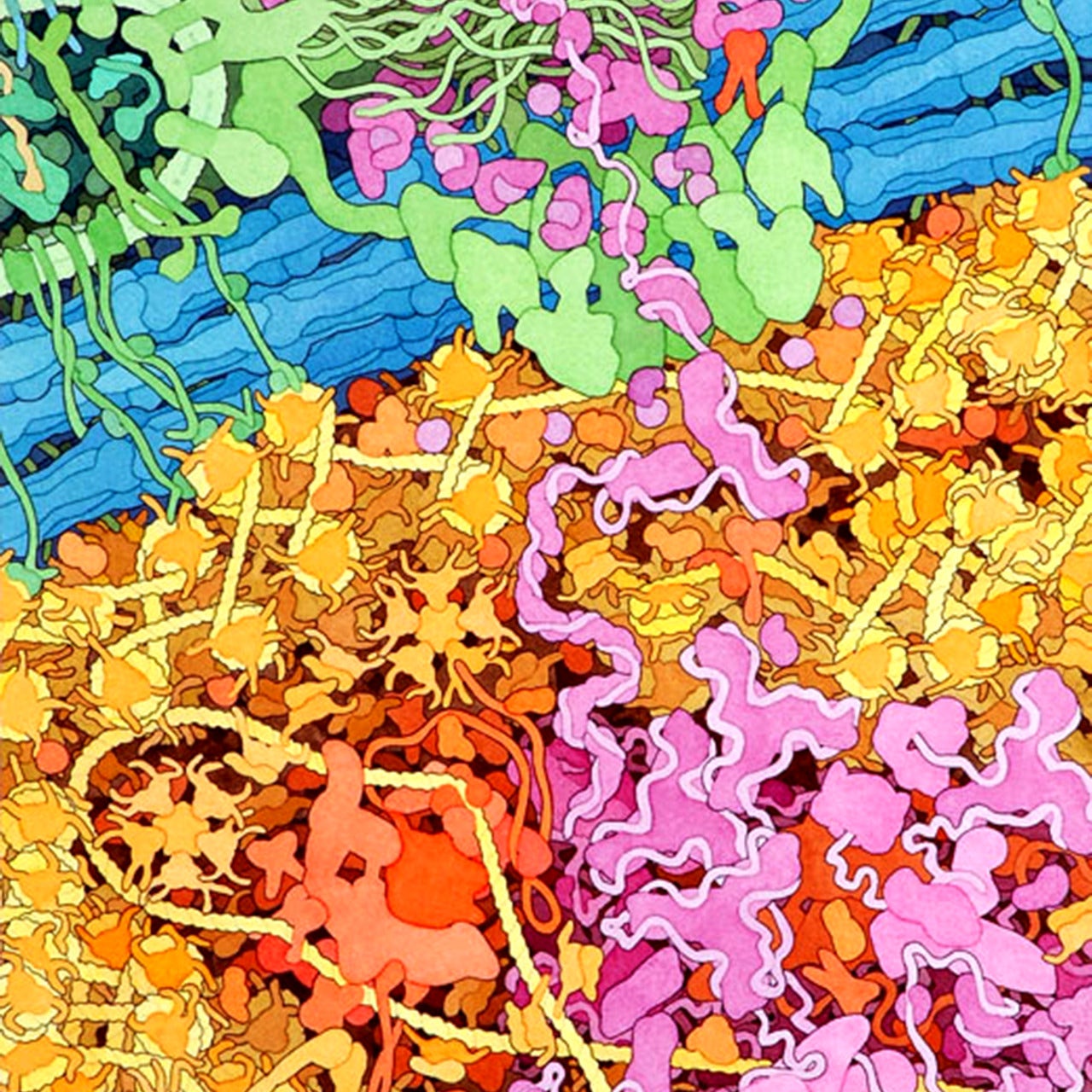
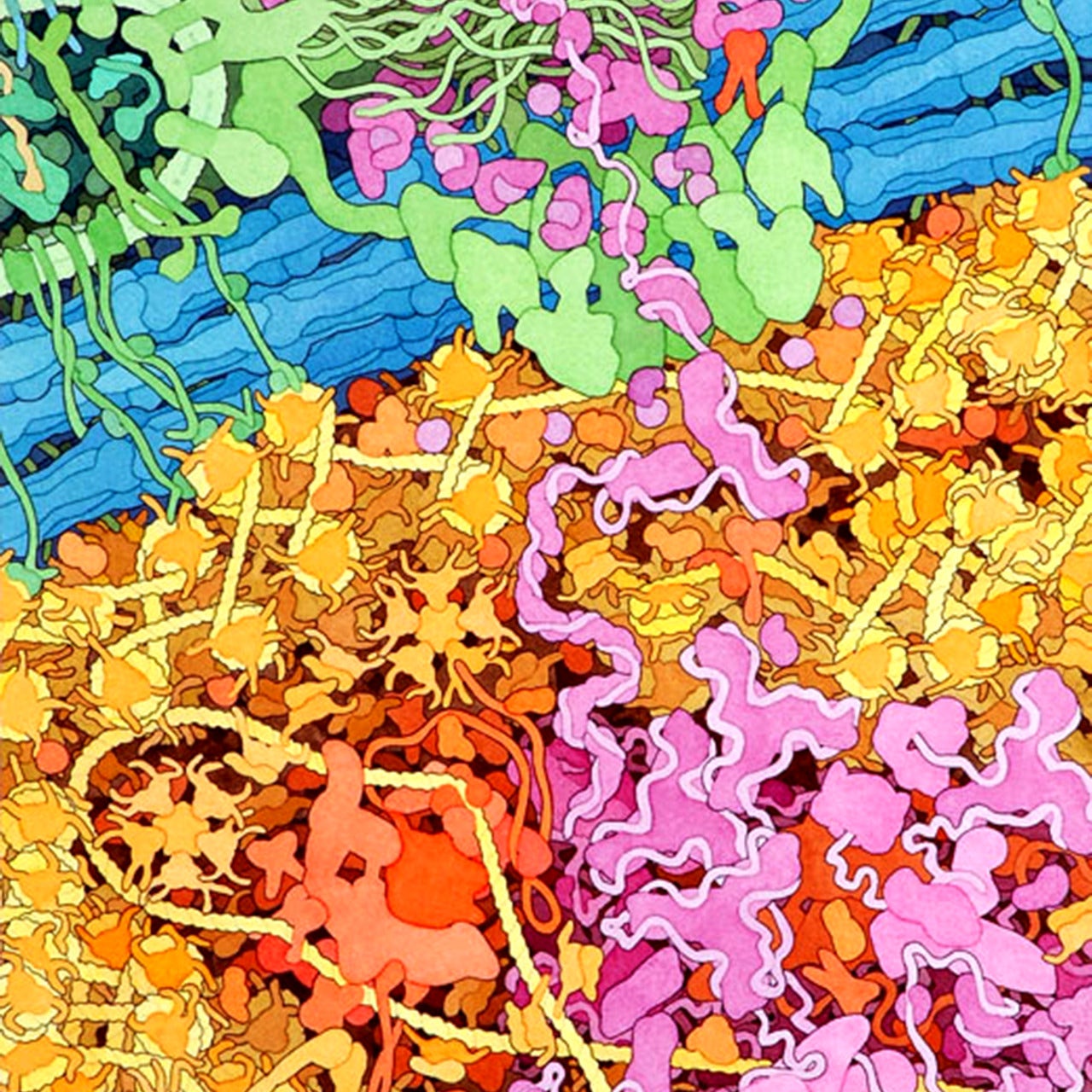
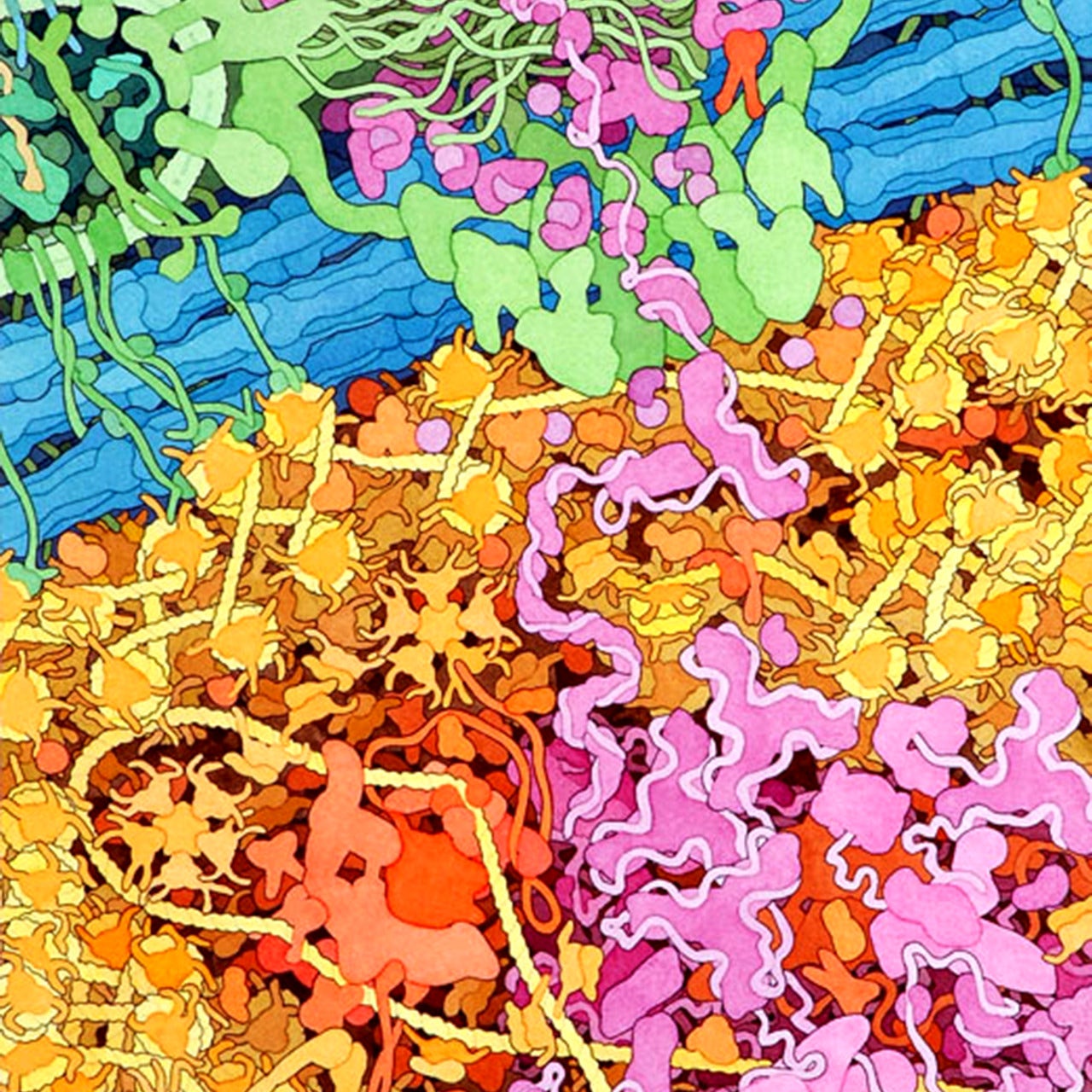
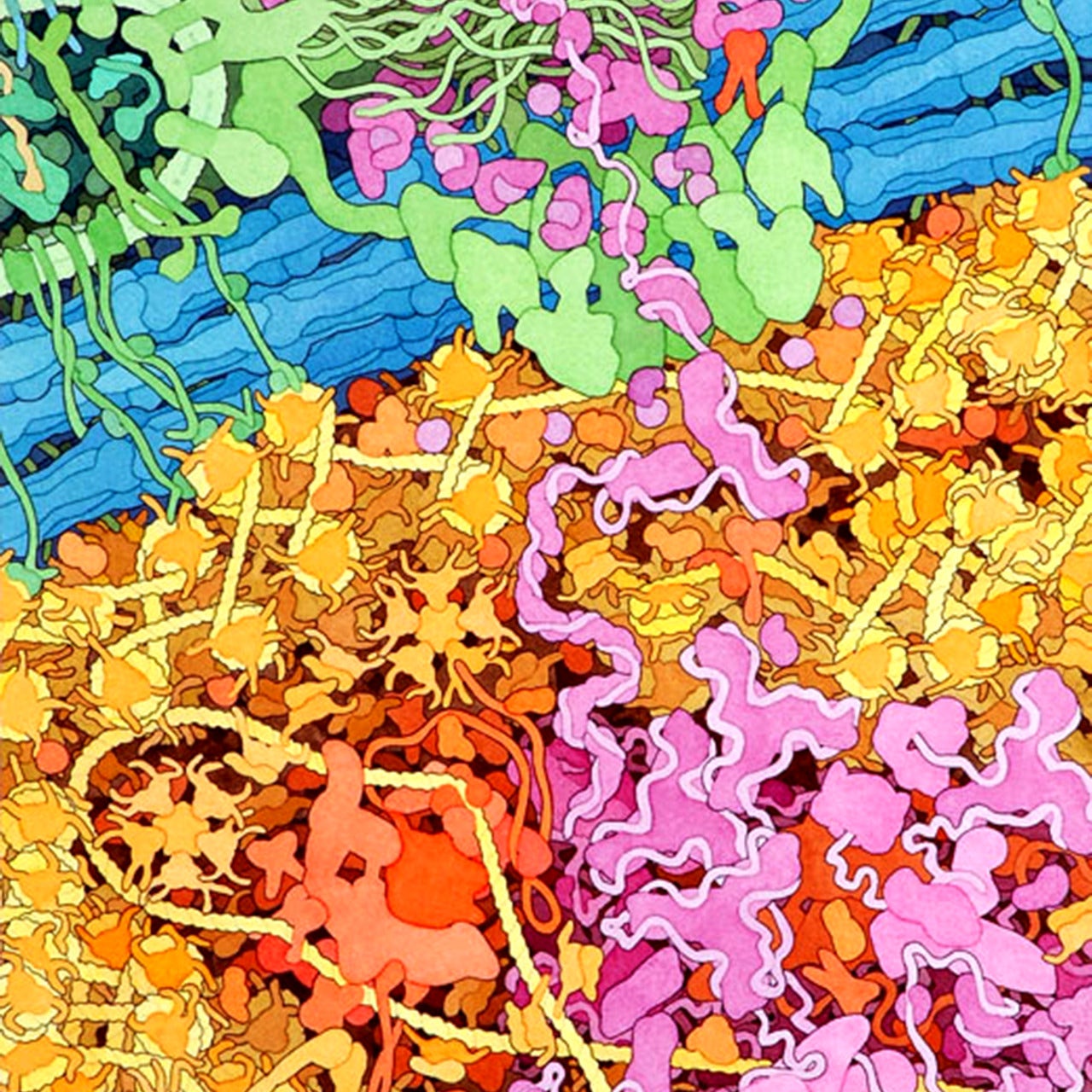
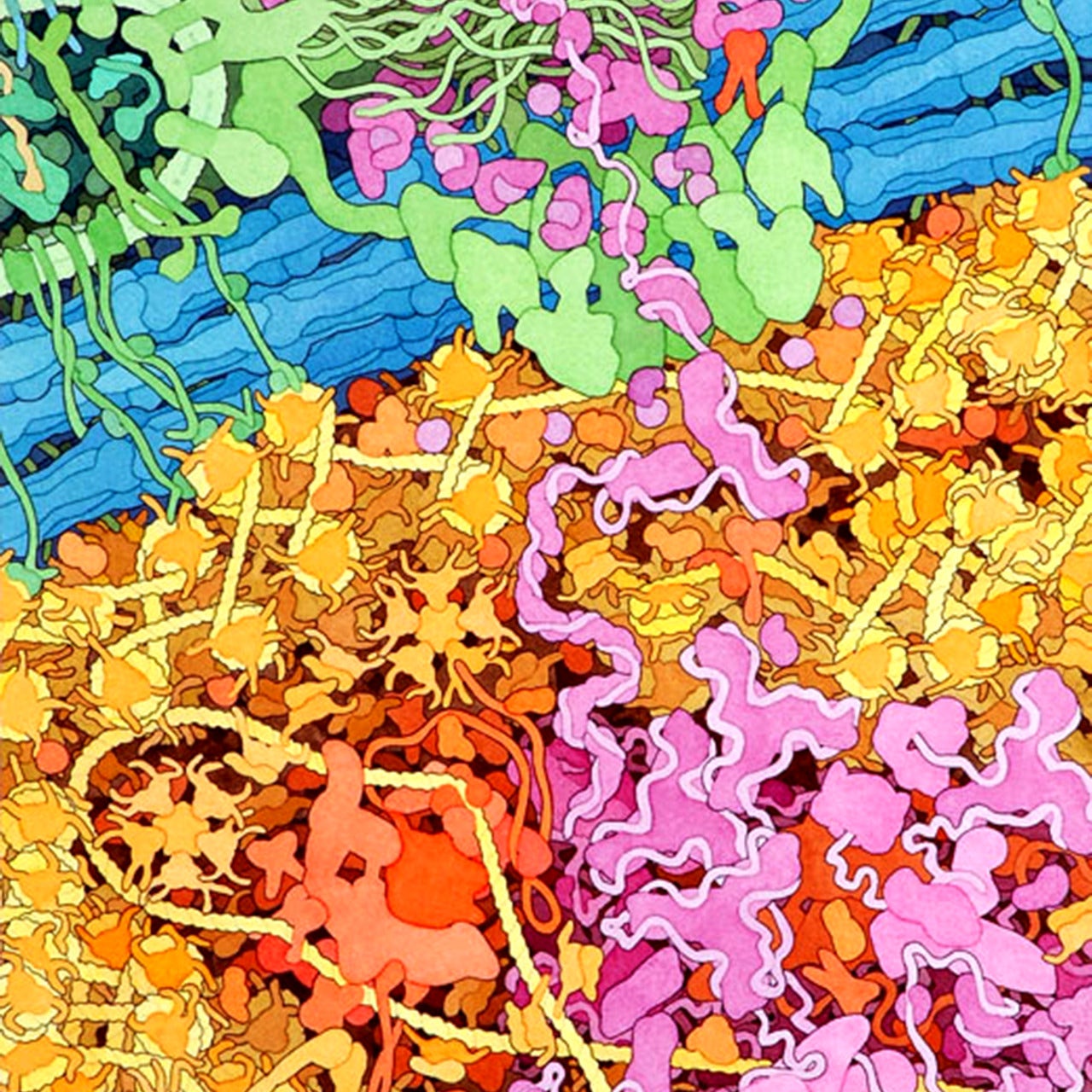
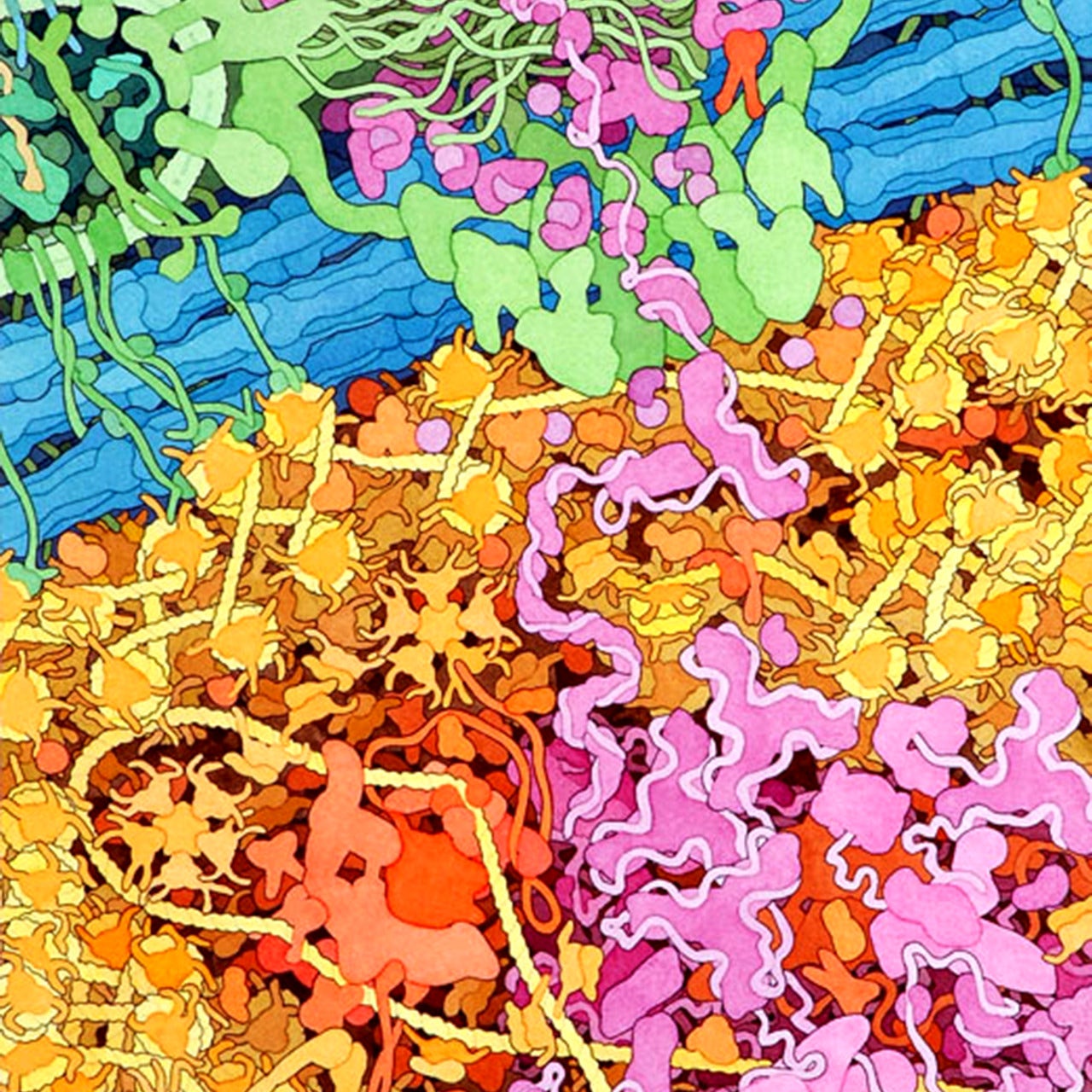
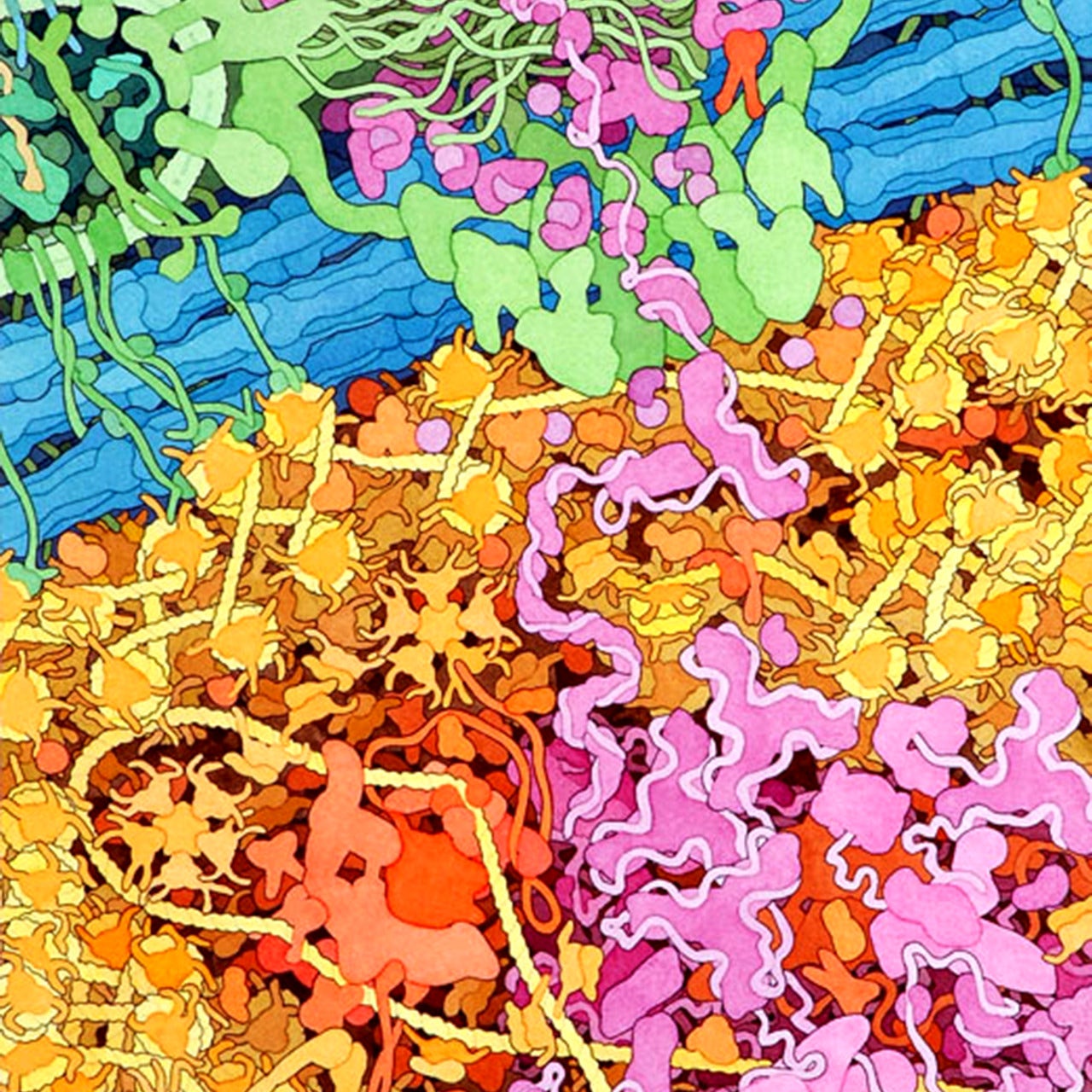
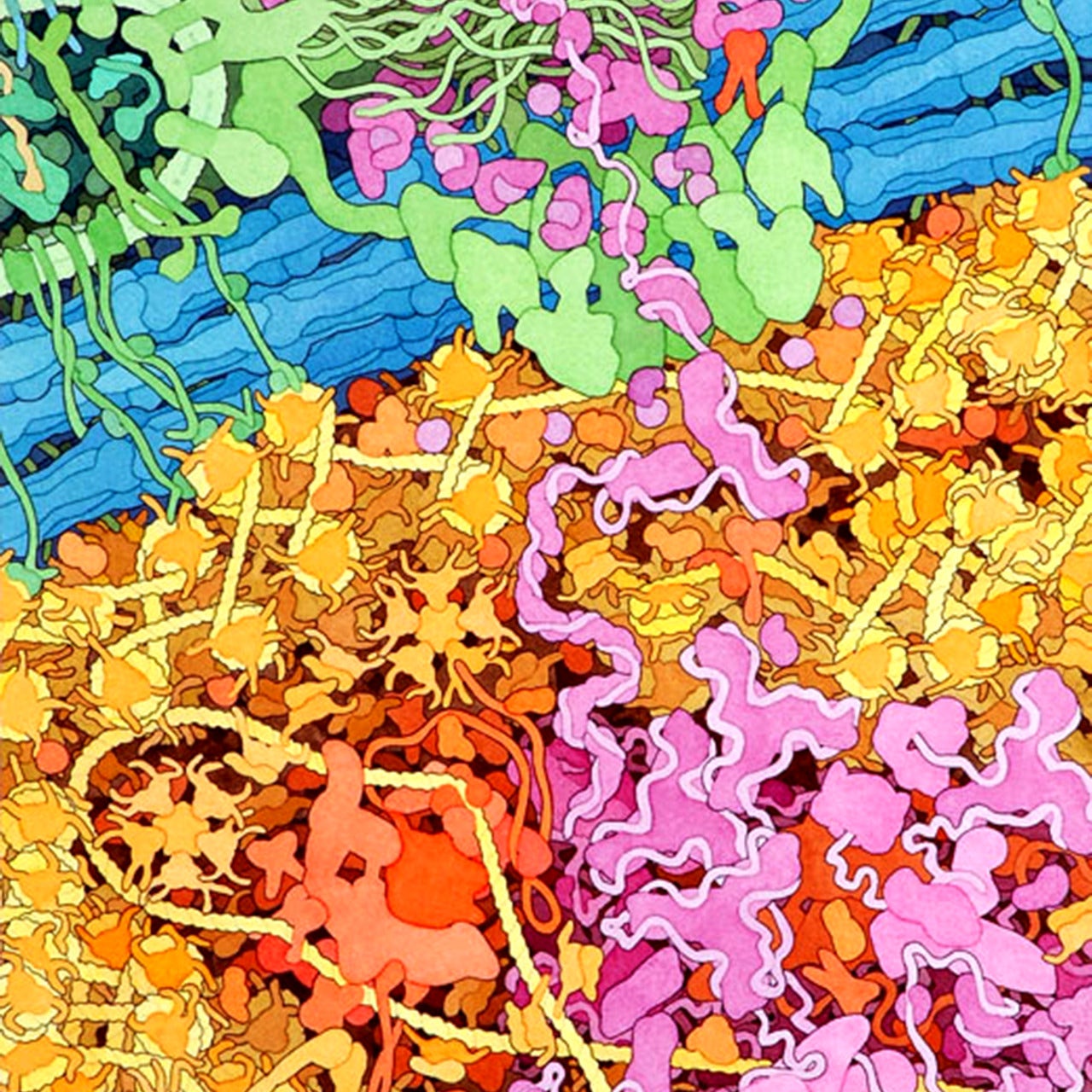
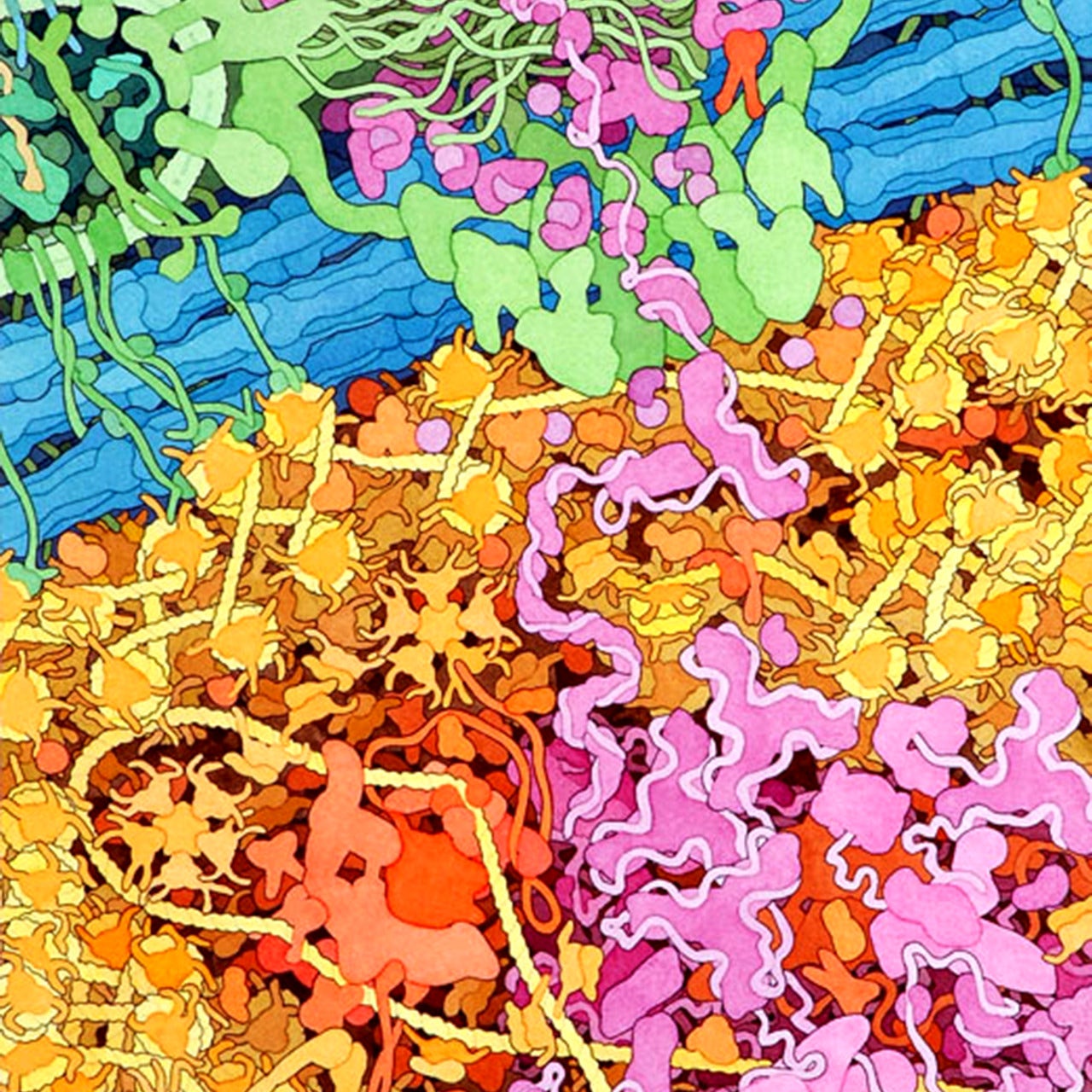
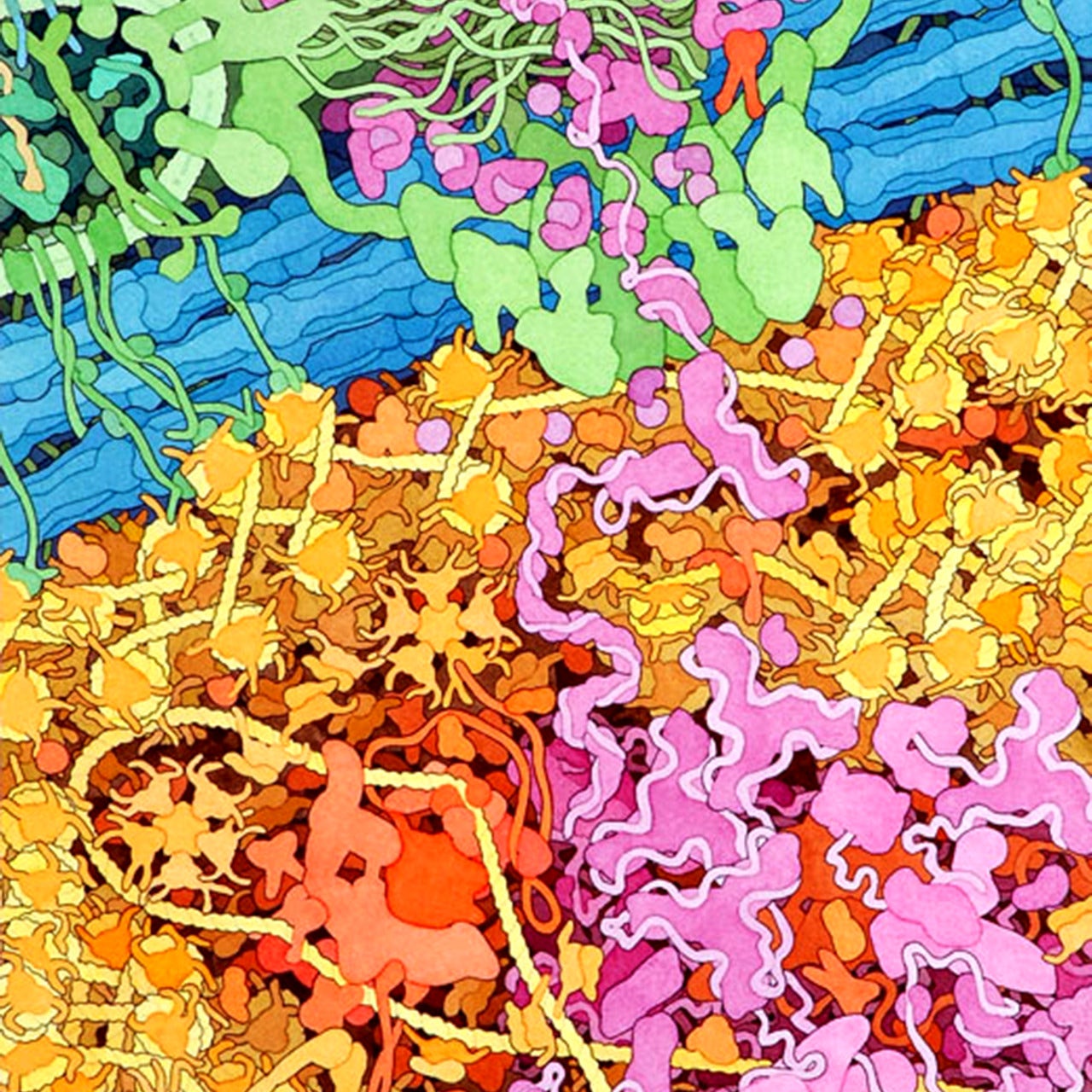
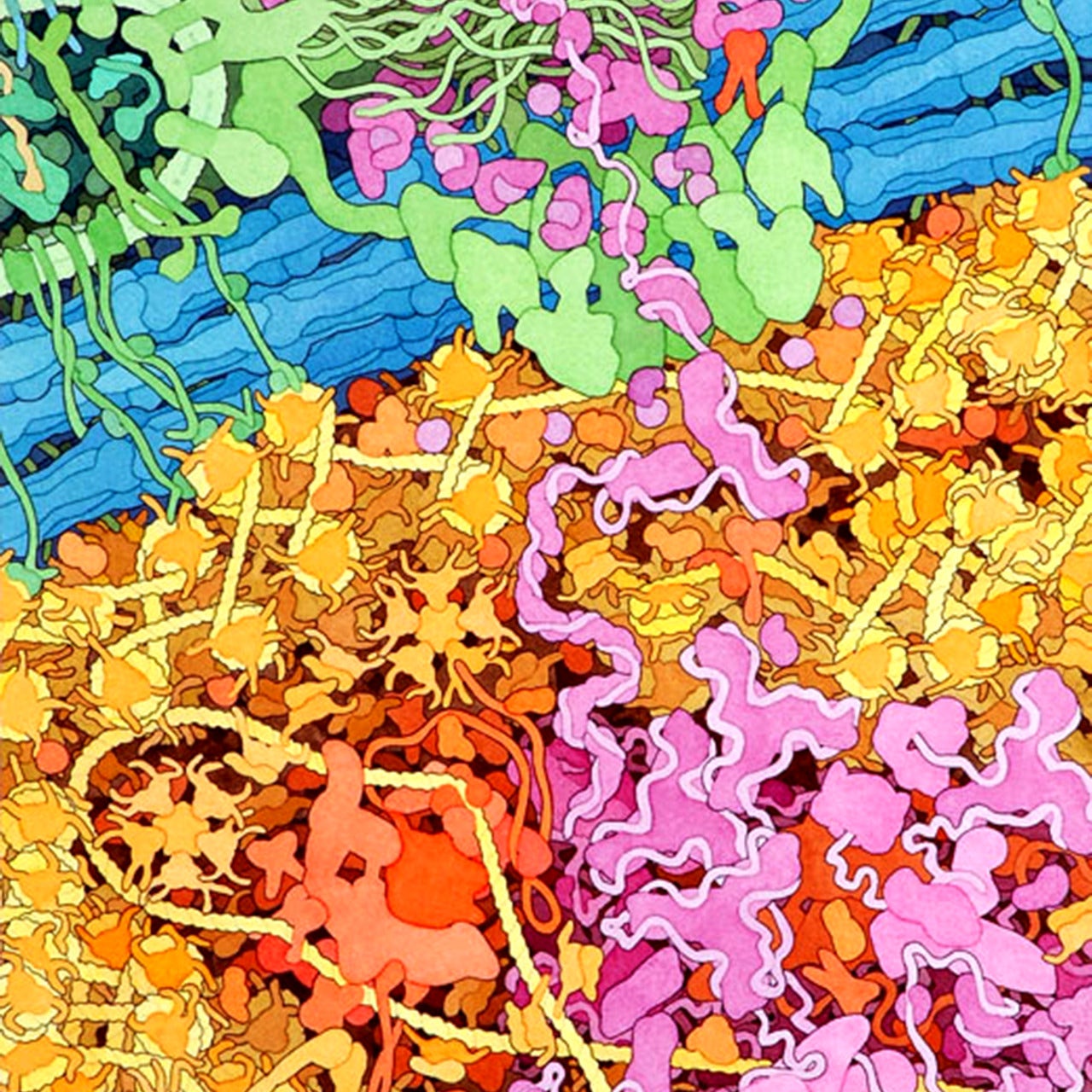