Fundamental advances in machine learning
Developing radical new approaches to inference and automated decision making using advances in quantum information and statistical physics.
The quantity of data in our increasingly digitised world is now far too great to be processed by hand. This has led to the rebirth of artificial neural networks as a framework for automated data processing. Whilst these networks can deal with an unprecedented amount of information, their capabilities and limitations are not yet fully understood.
This project investigates the inner workings of both classical and quantum neural networks, in an attempt to unpick their “black box” natures. Analytic methods shed light on the functions computed deep within two different types of classical neural networks. We show that the simpler set-up computes the same range of functions as the more complex one, meaning that resources can be saved. Furthermore, we pose a way of generalising classical neural networks so that they can deal with quantum inputs, and also maintain the reversible nature of a quantum signal’s progression through a network.
Developing our understanding of classical and quantum neural networks simultaneously allows for the two fields to grow together. They are having profound effects on the way society runs through their use in energy harvesting, autonomous vehicles and social media, but as such, need to be treated with care.
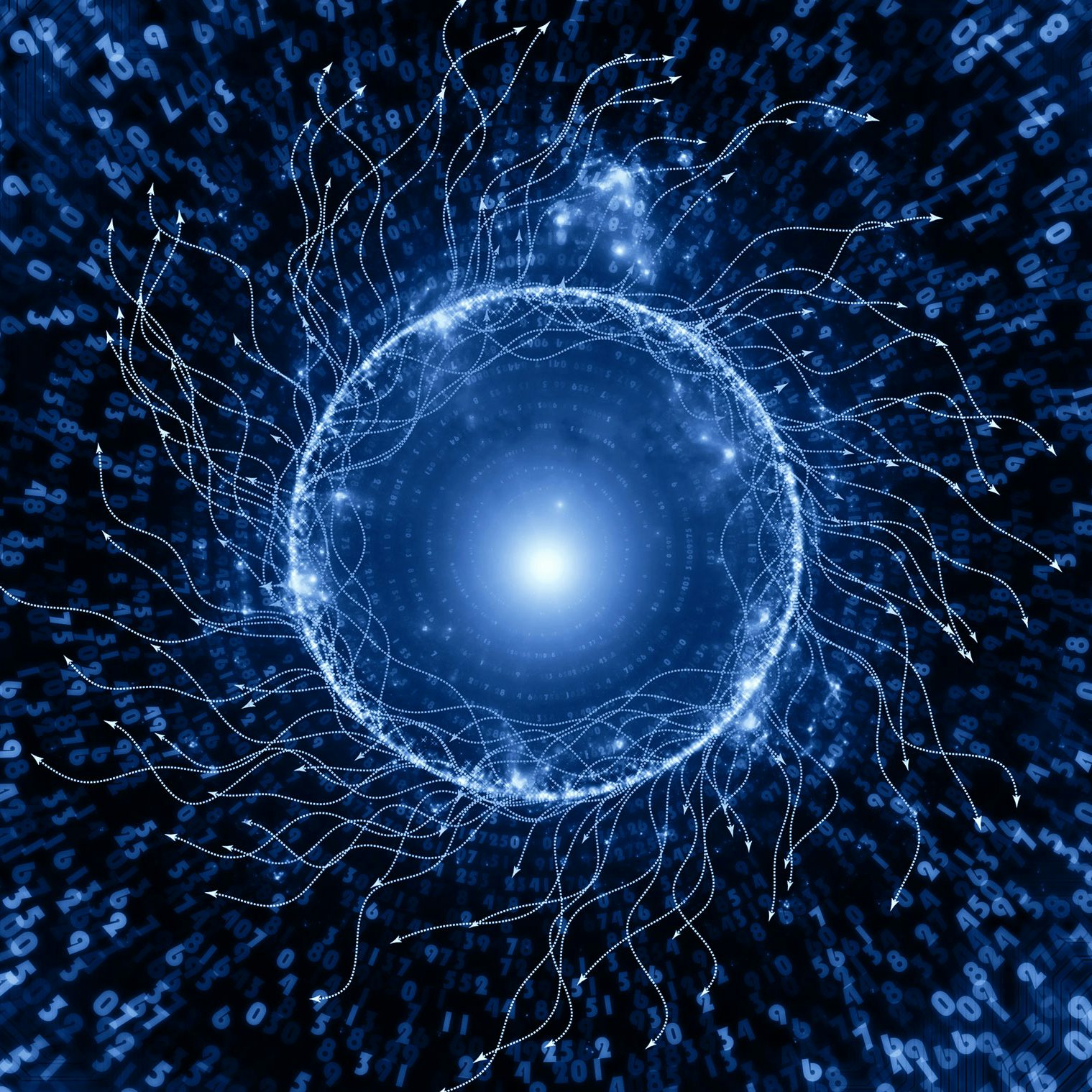
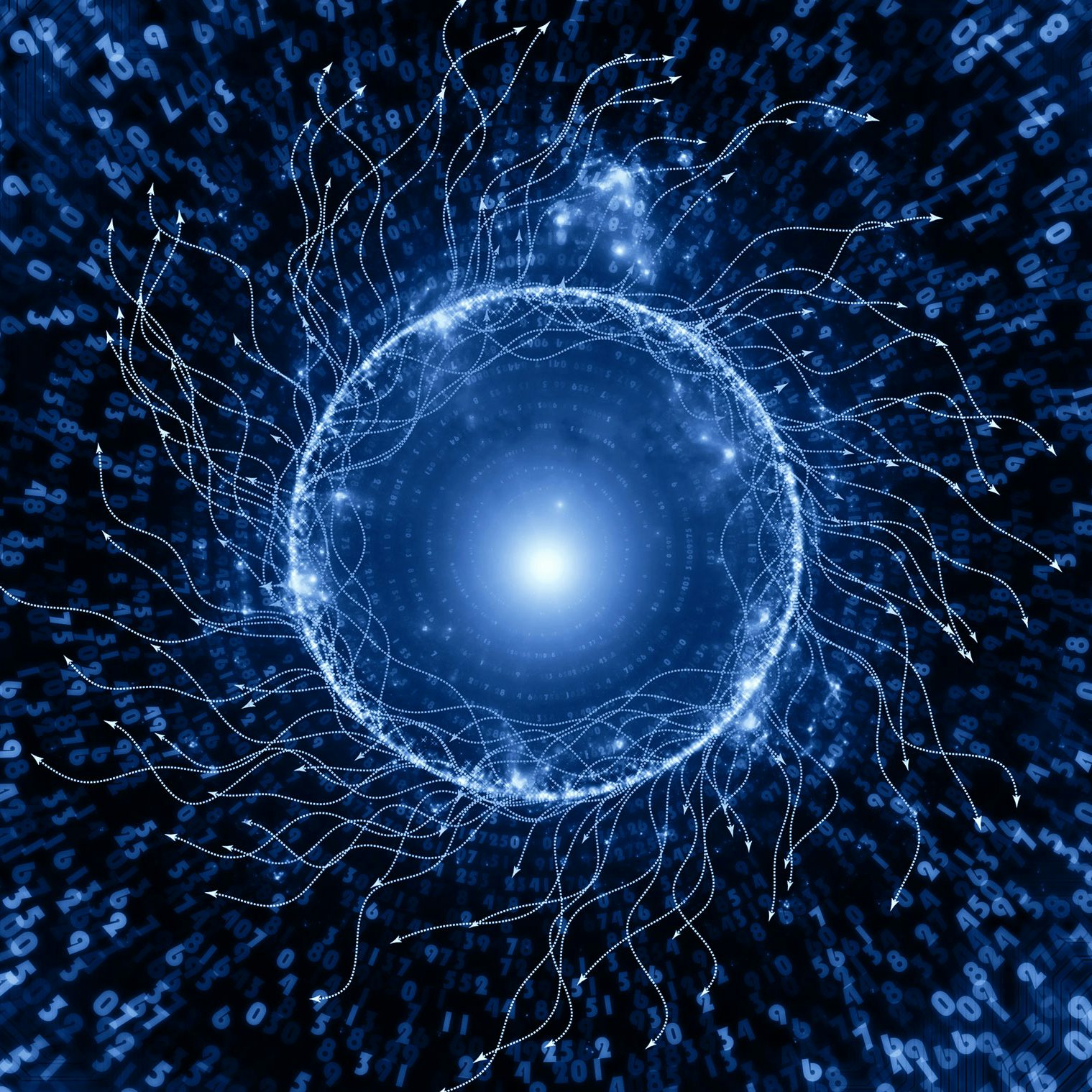
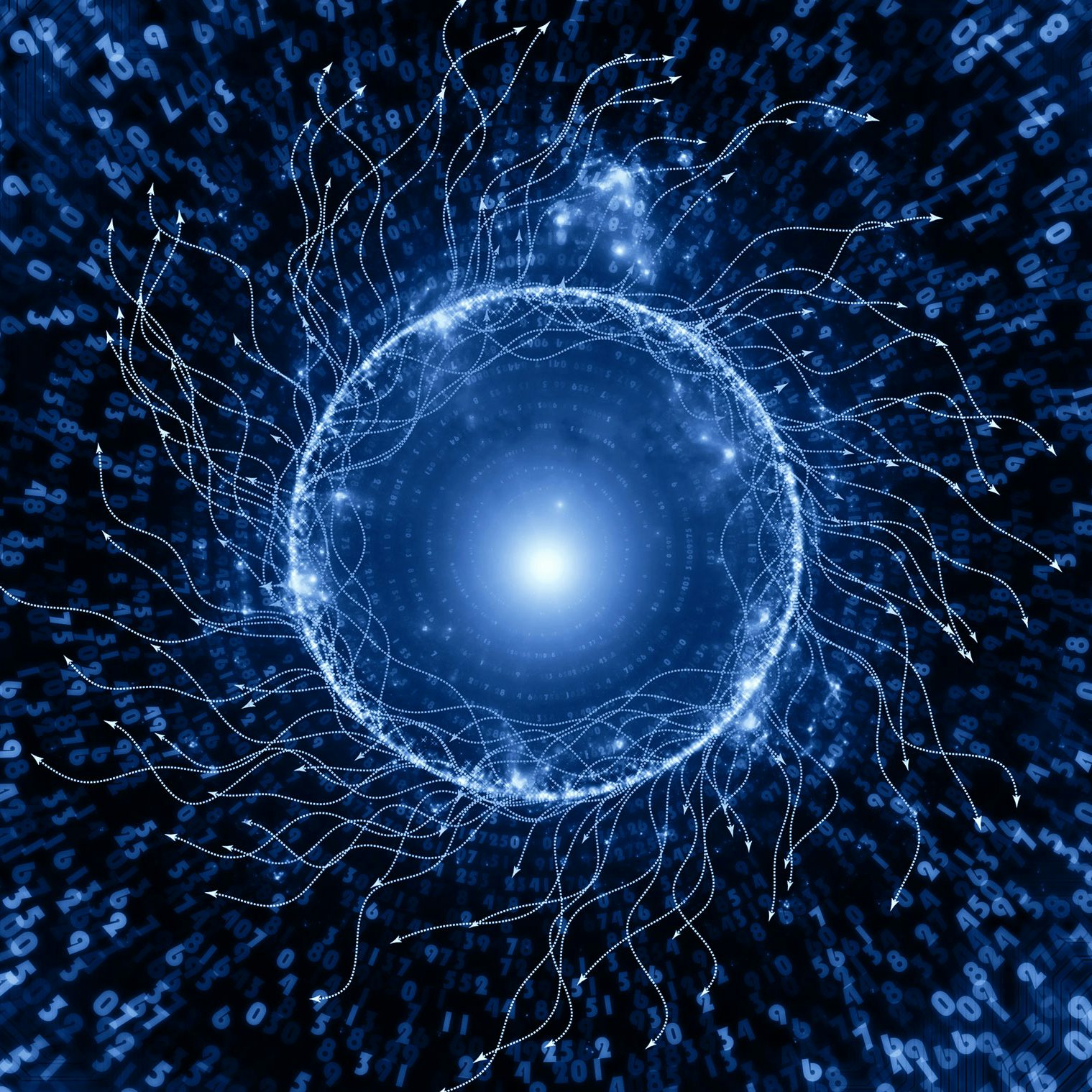
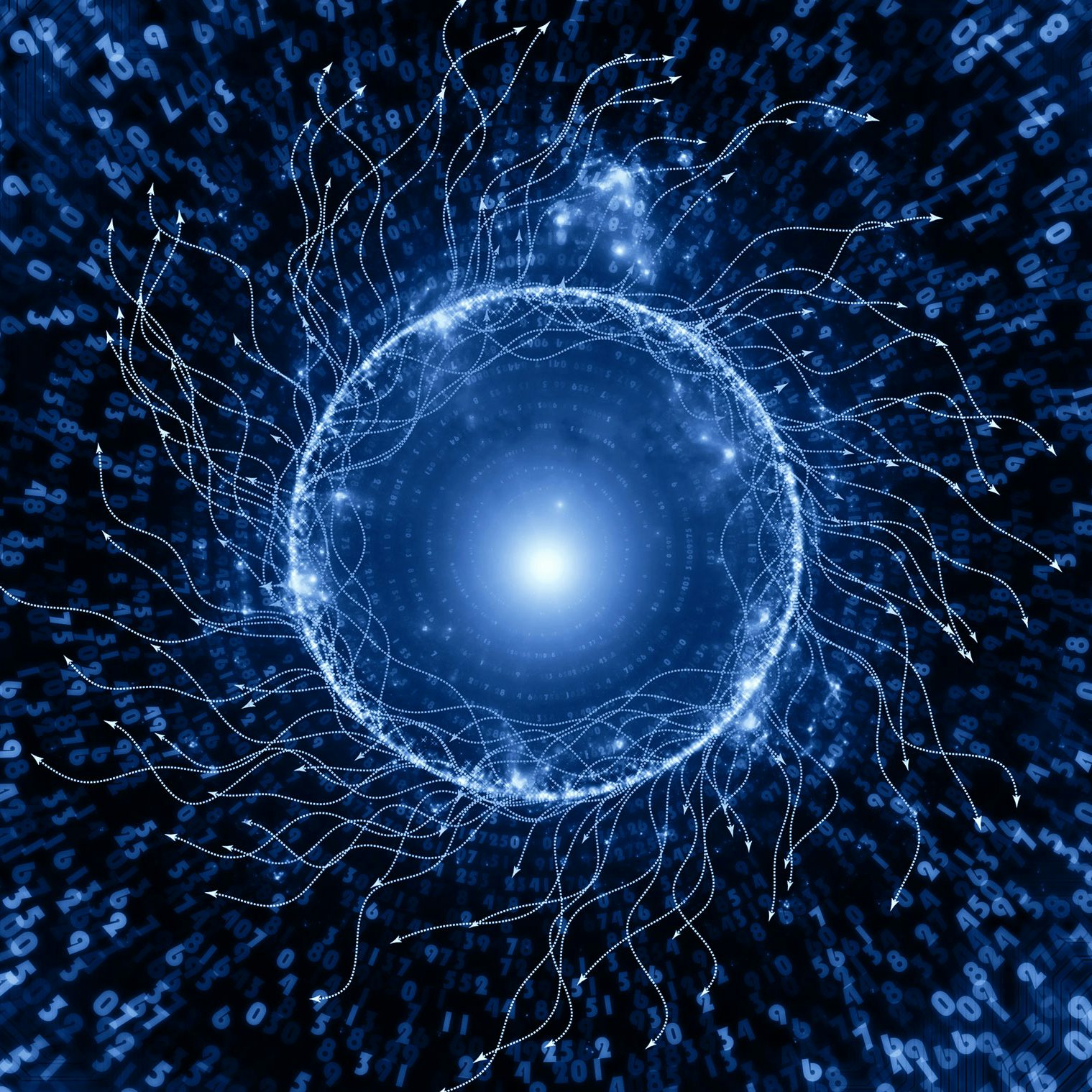
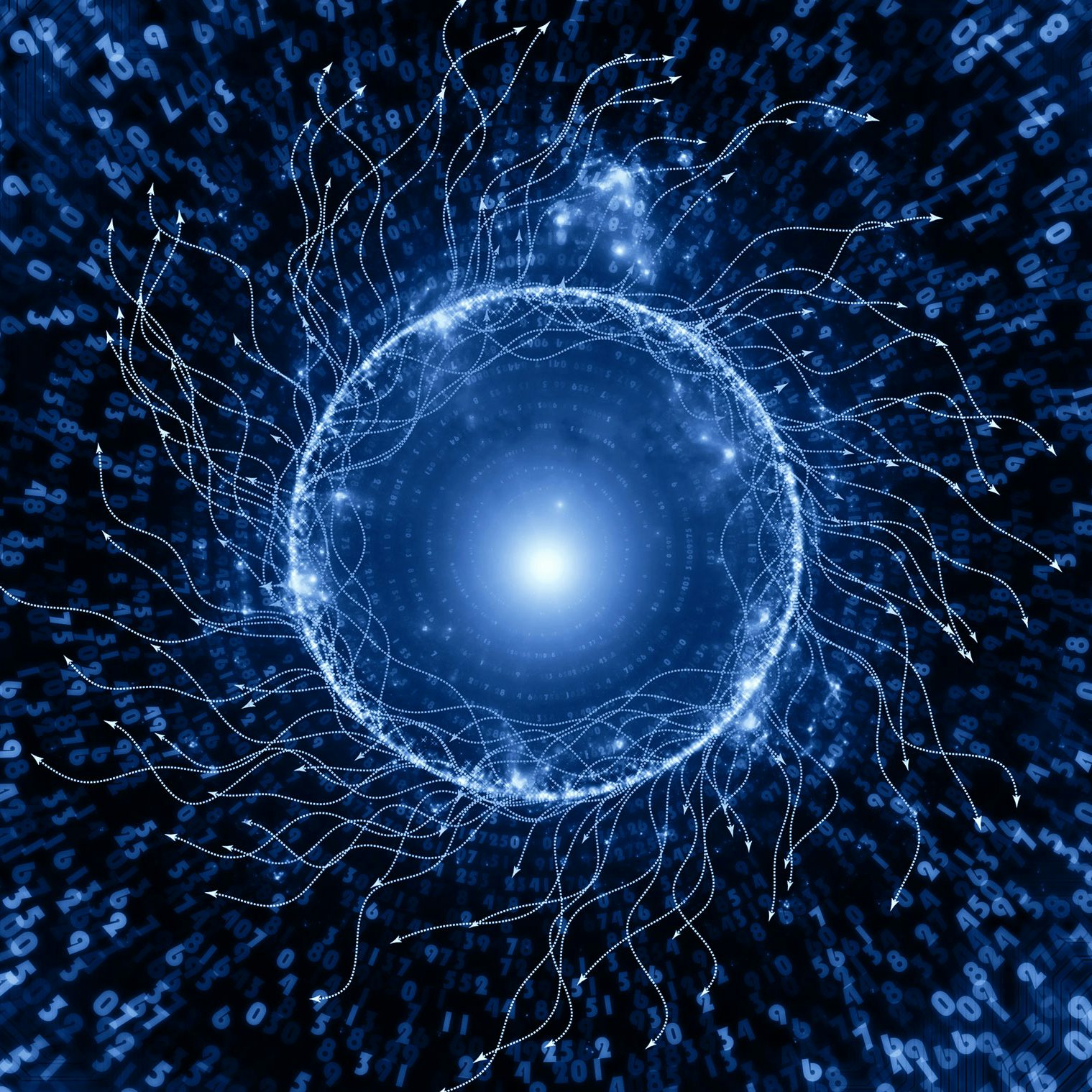
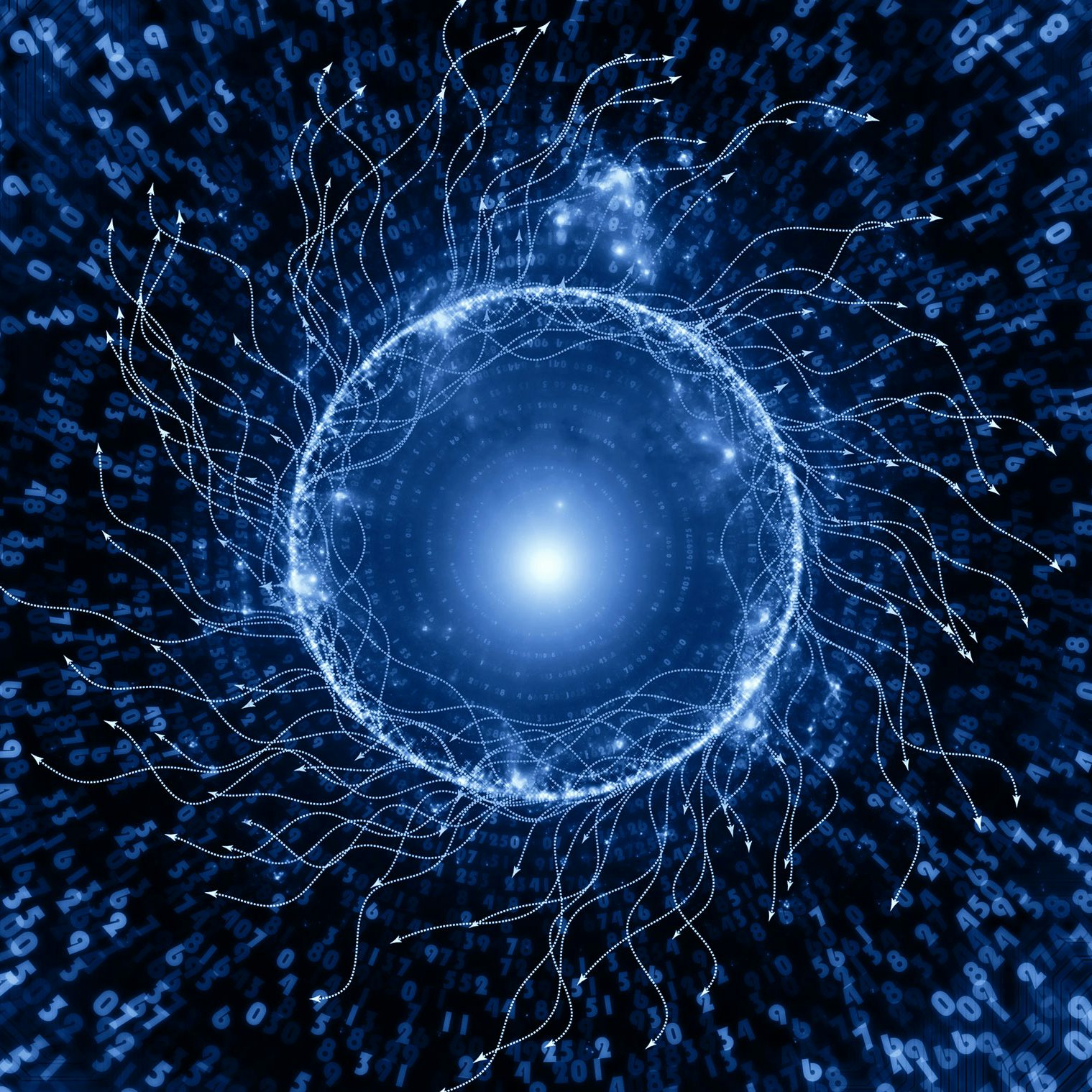
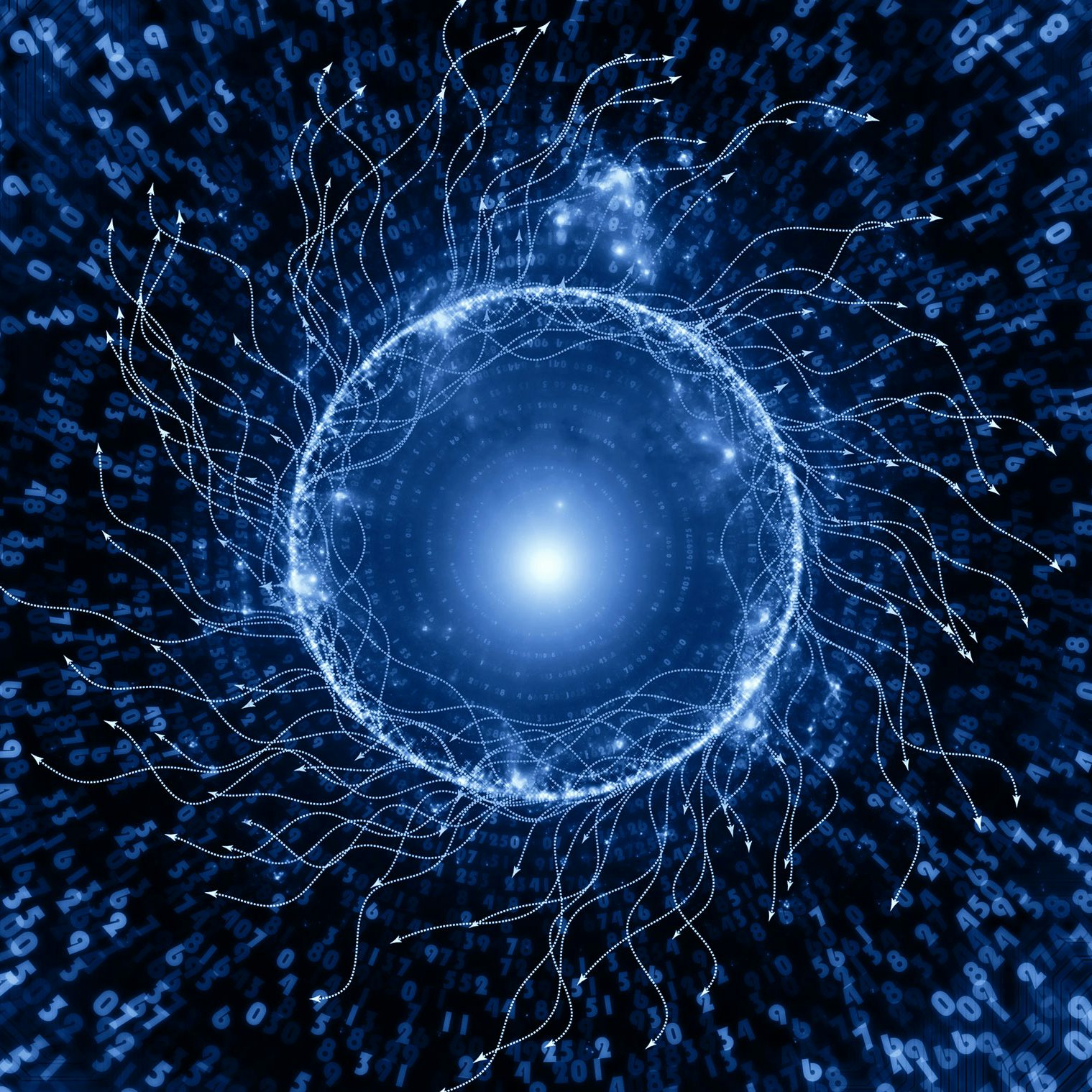
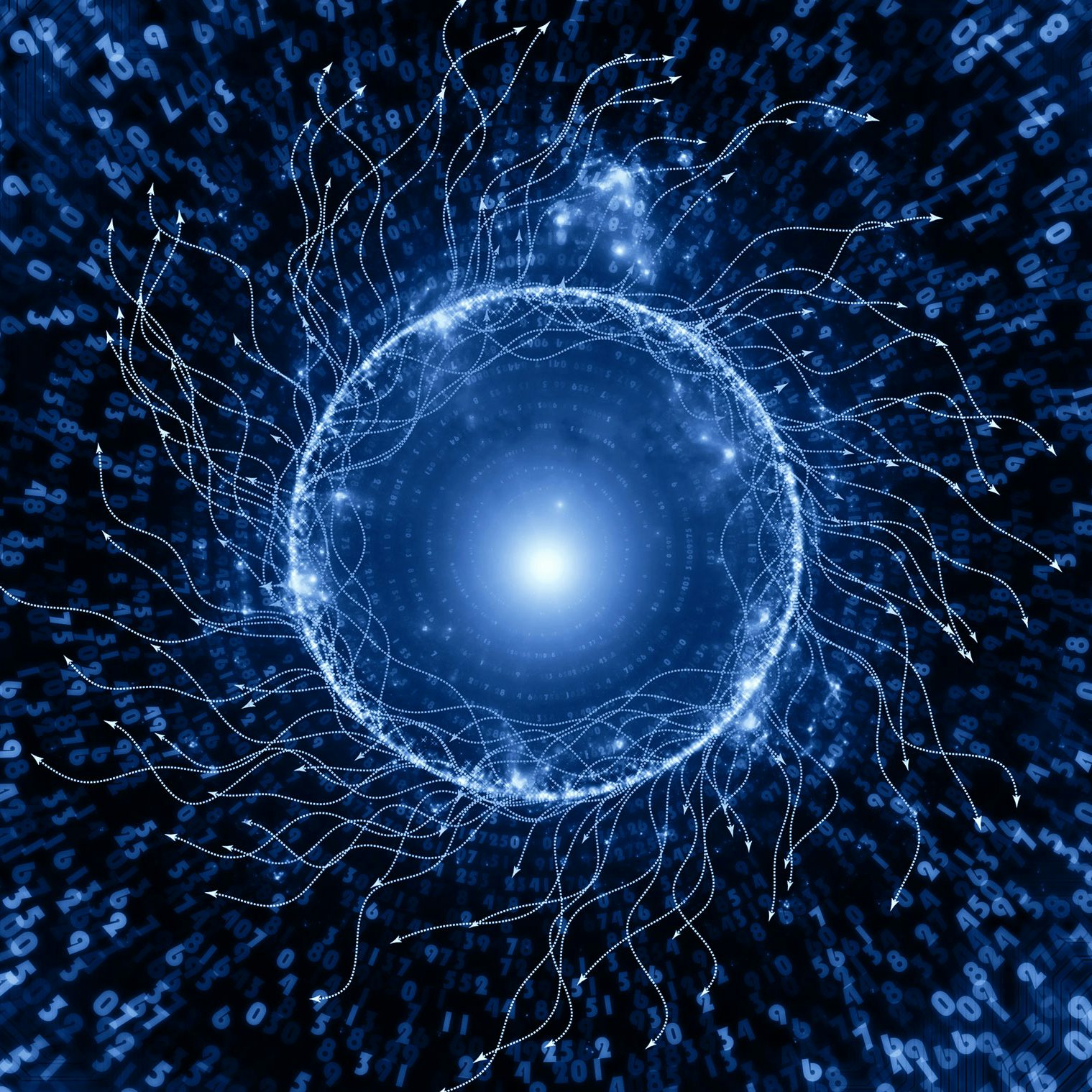
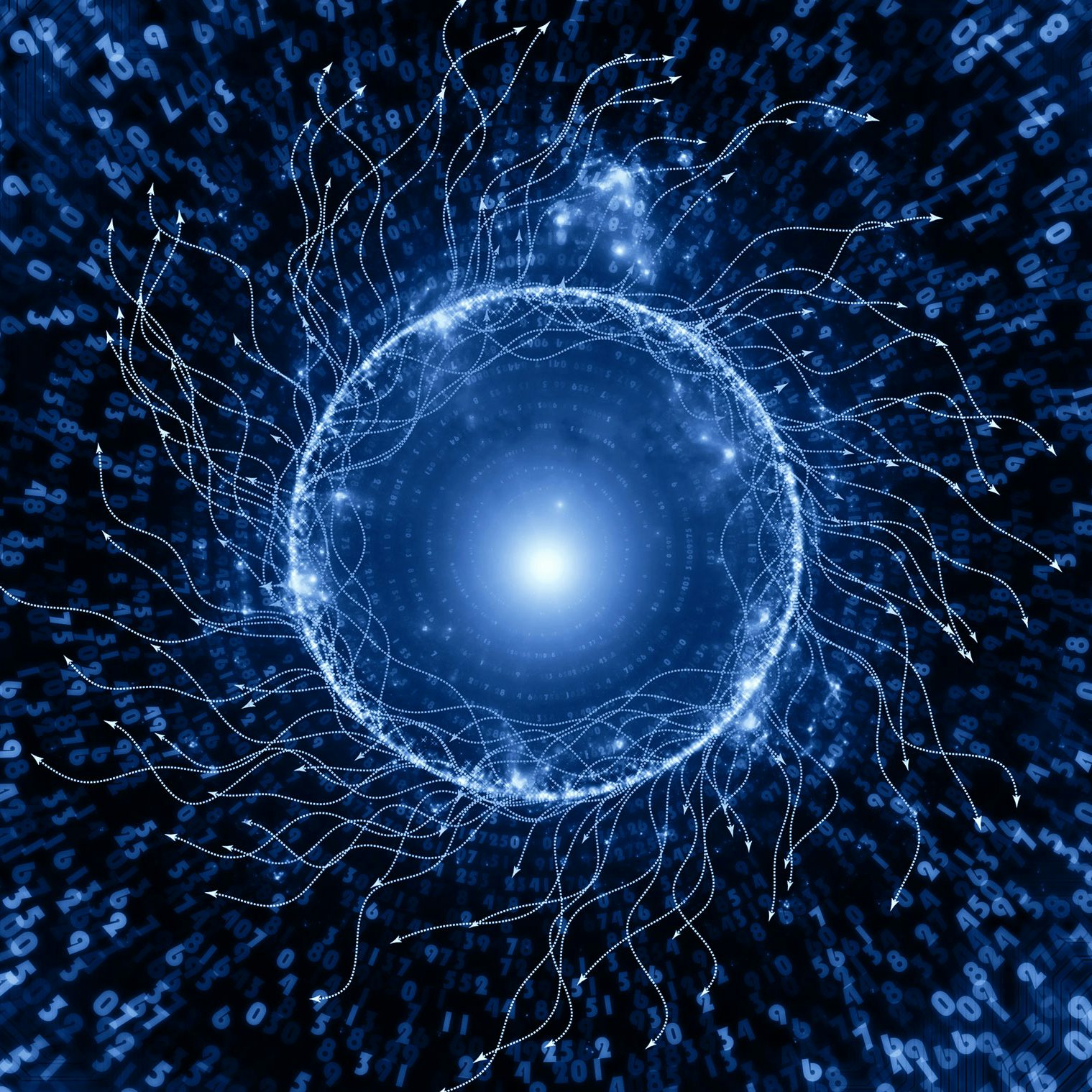
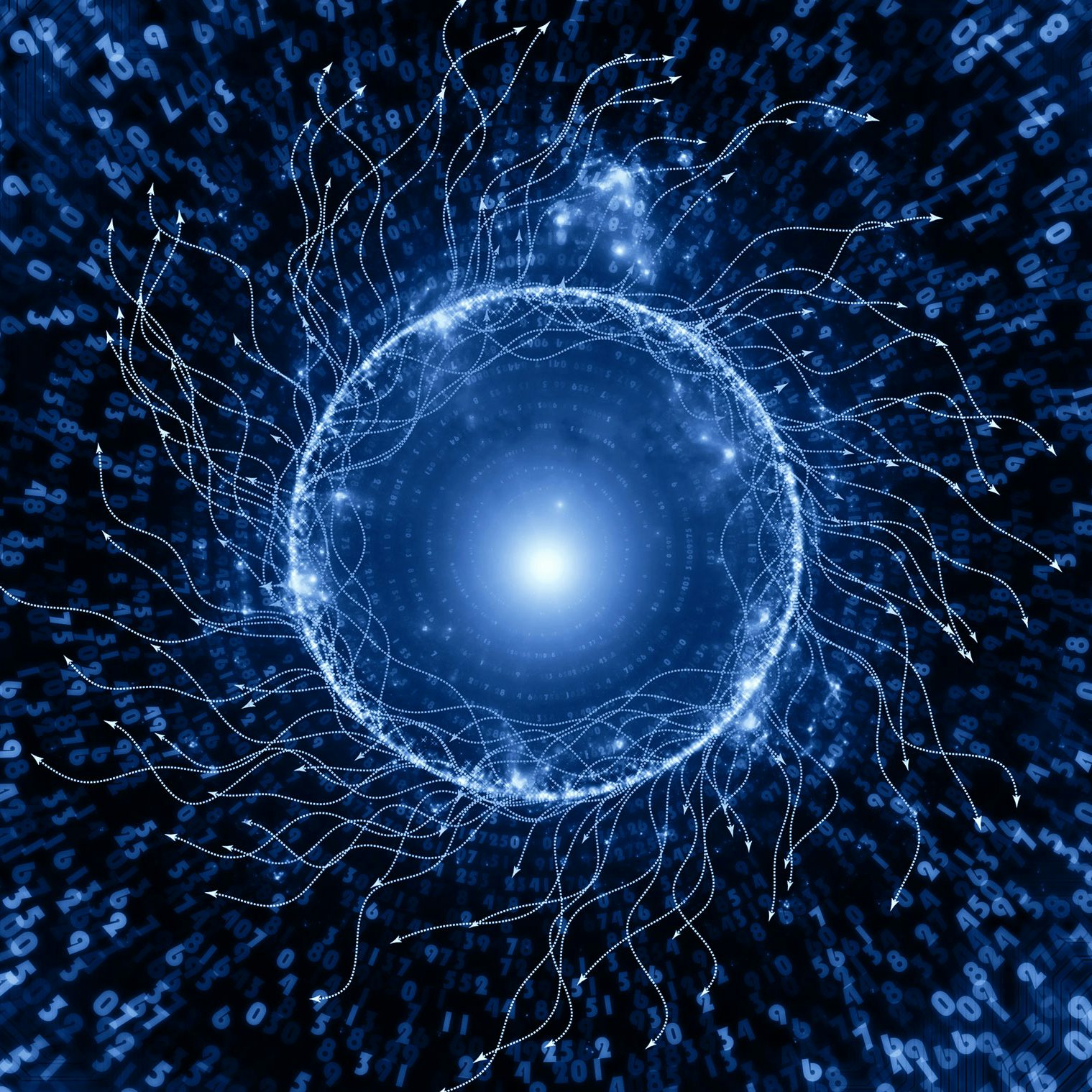
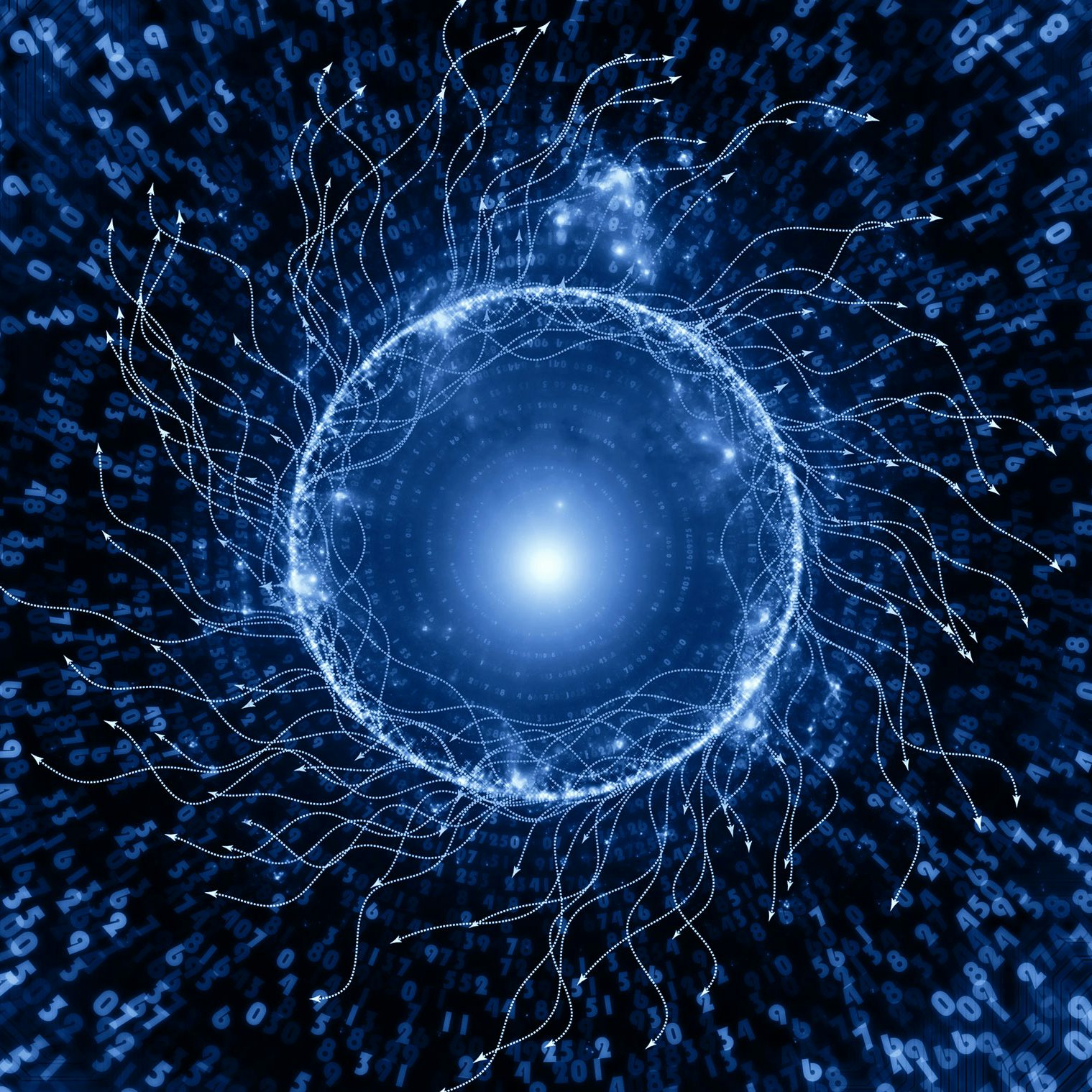
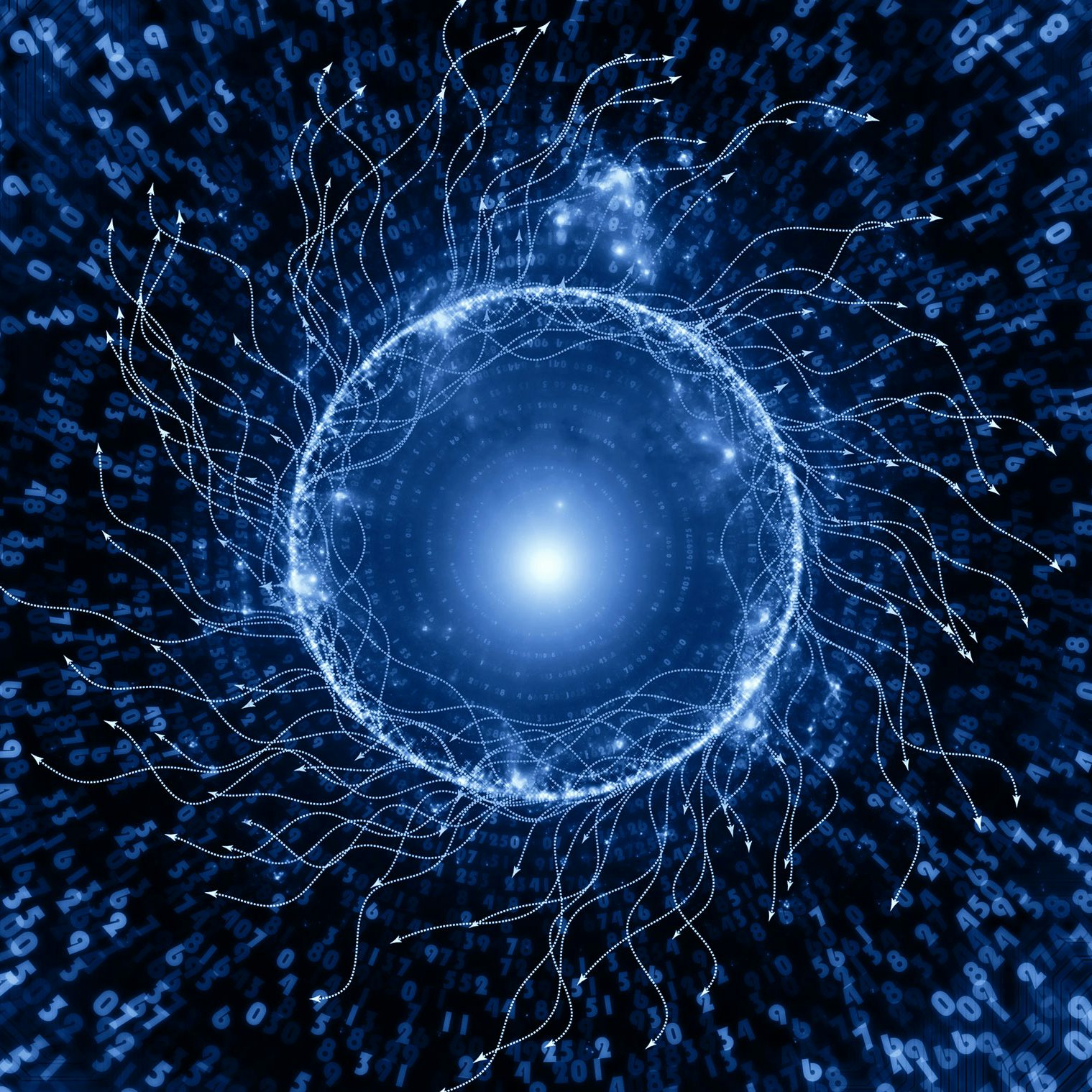
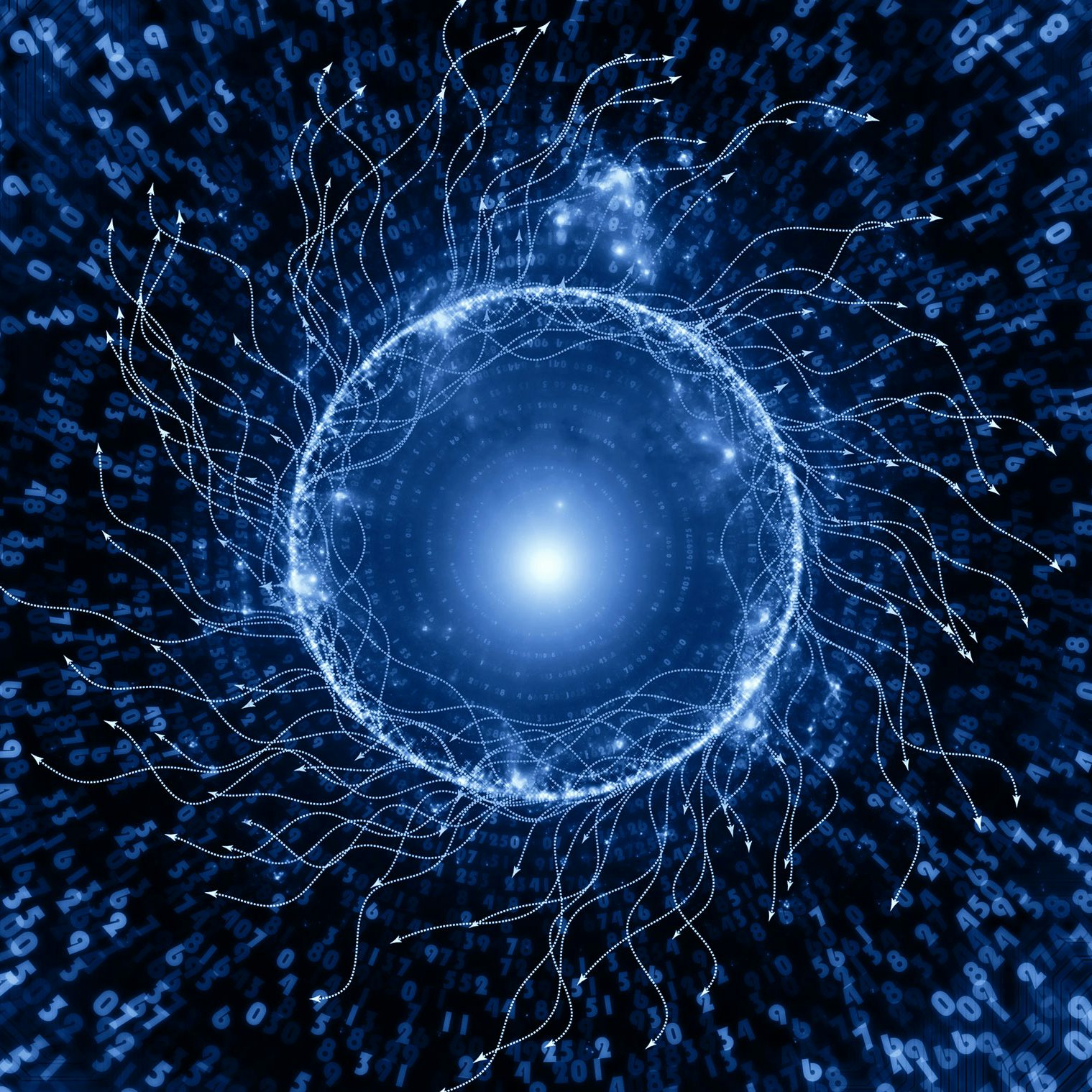
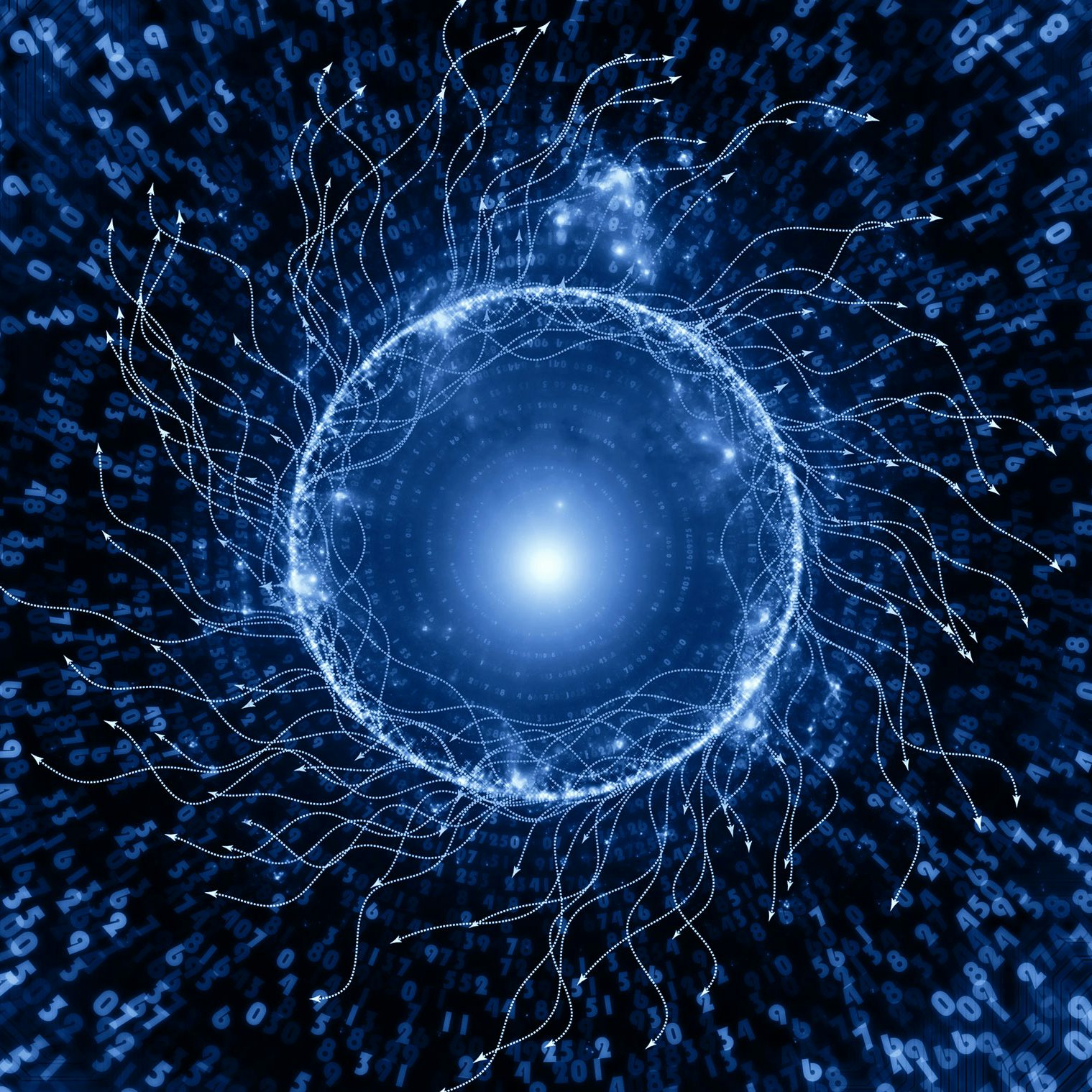
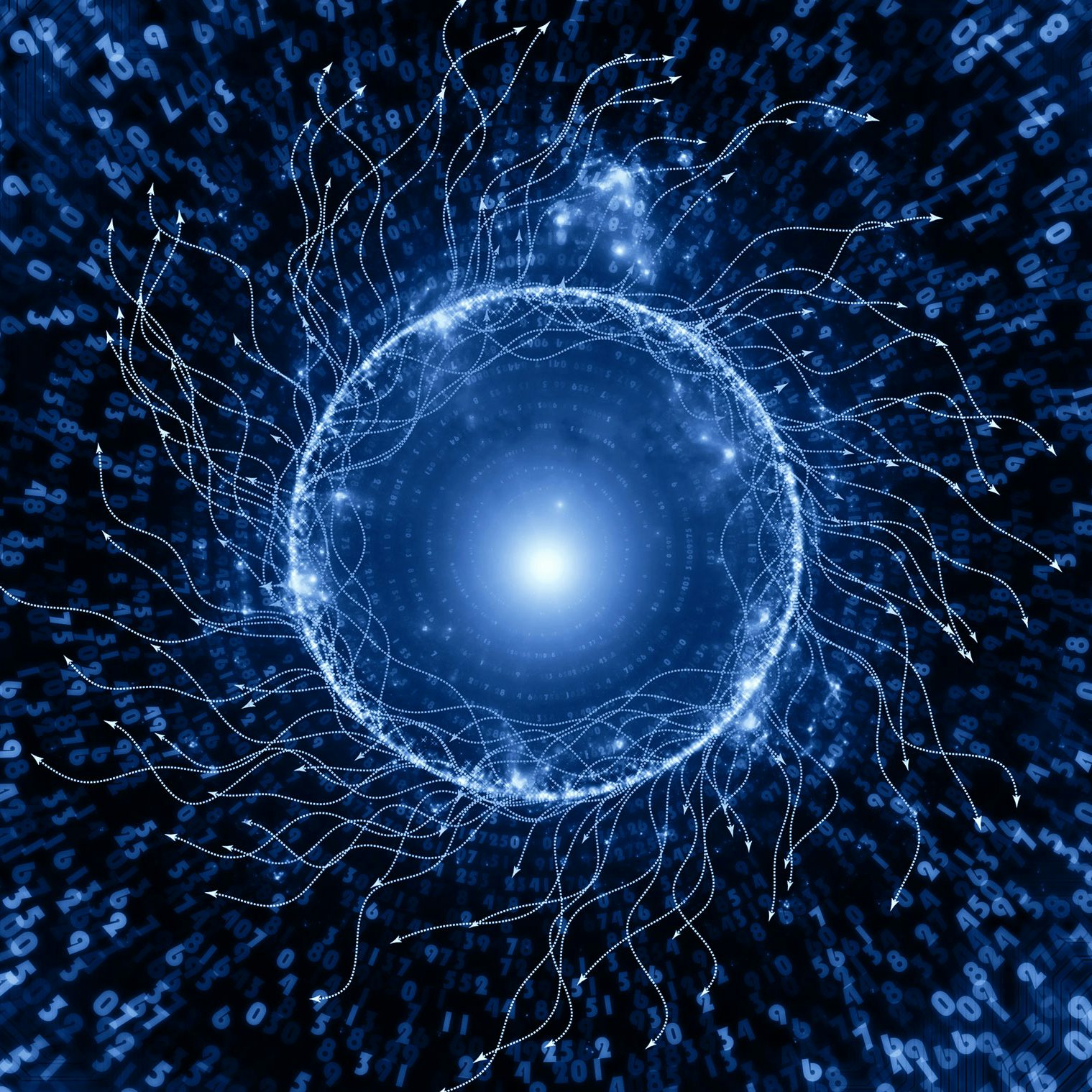
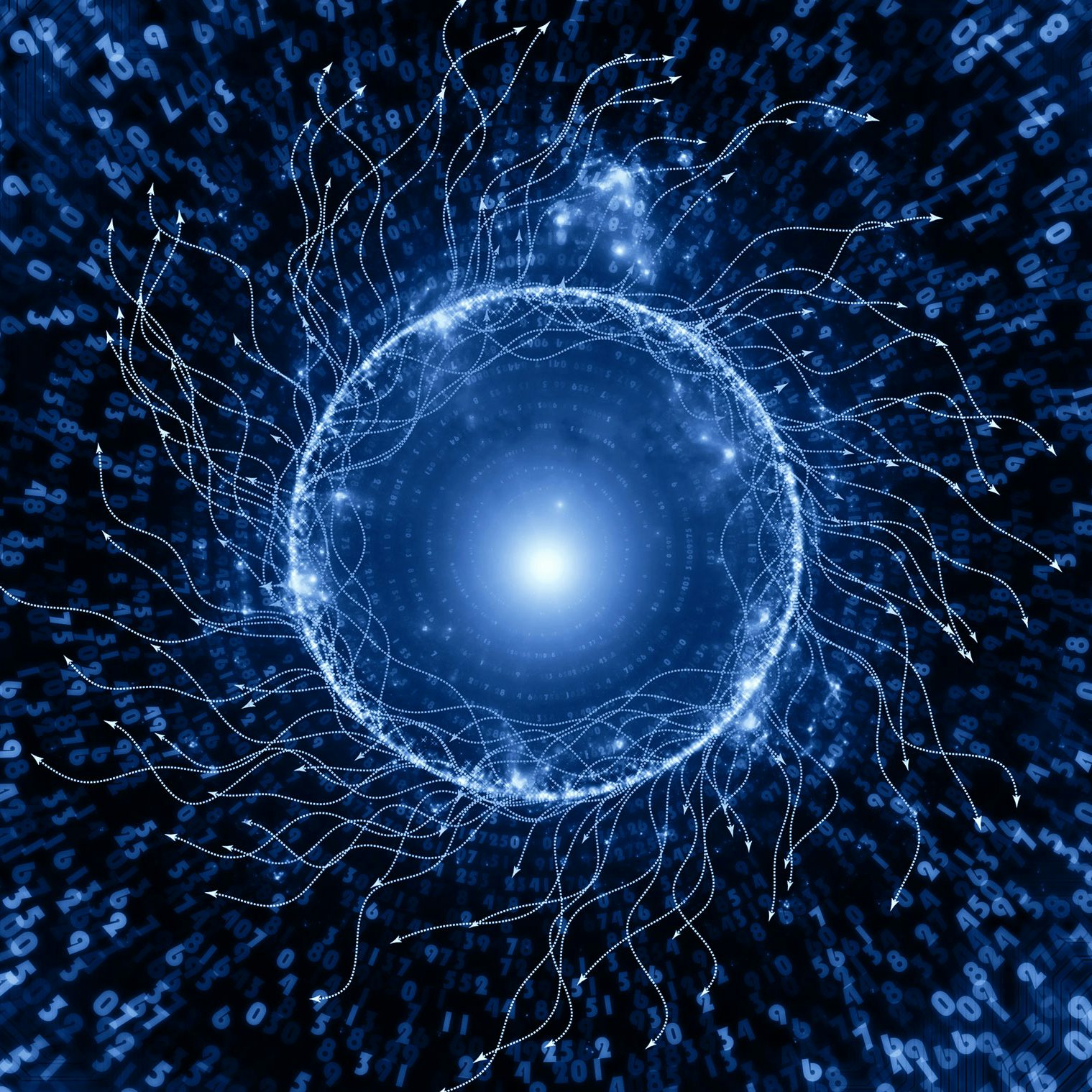