Irix uses machines to speed up discovery
Research Fortnight, 30 OCT 2024
The London Institute, which uses humans to speed up fundamental discovery, launches Irix, a company that harnesses machines to do the same.
Before the Nobel Banquet in Stockholm’s Blue Hall on 10 December, someone faces the unenviable task of coming up with a seating plan for the 1,300 guests. Yet it so happens that a couple of the attendees will be, in a sense, the greatest living experts at creating optimal seating plans.
I’m talking about Demis Hassabis and John Jumper of Google DeepMind, two of the three winners of this year’s Nobel Prize in Chemistry. The citation praises their creation of AlphaFold, “an AI model to solve a 50-year-old problem: predicting proteins’ complex structures”.
A protein consists of a two-dimensional chain of amino acids. To create its 3D structure, that chain bunches up so that friendly molecules are next to each other and far from their enemies. To put it another way, it’s the best arrangement of tables in a banqueting hall to accommodate a given sequence of guests.
Make no mistake: this is a milestone in the history of science. That’s not because of the implications for biology and medicine. It is because it’s the first time the Swedish academy has singled out a discovery driven by machine intelligence.
AI-driven discovery
By definition, a technology is a combination of more primitive technologies that harnesses a scientific principle. The space of new technologies depends on the scientific principles available to choose from.
Imagine if AI could uncover not just new technologies but the very principles upon which future technologies will be built. Now let me make a prediction: it is poised to do so foremost in my own field of the mathematical sciences.
There are two reasons. First, real-world experiments and simulations are plagued by false negatives and false positives—by their own account, Hassabis’ and Jumper’s tool for protein prediction suffers from “hallucinations” of “spurious structural order”. But there are no coincidences in the mathematical sciences. A single counterexample leaves a conjecture dead in the water.
Second, mathematical data are cheap. Primes, knots and groups are abundant or easily computed. The On-Line Encyclopedia of Integer Sequences contains 370,000 entries, from the ubiquitous Catalan numbers (1, 1, 2, 5, 14, 42…) to the crafty Look and Say sequence (1, 11, 21, 1211, 111221…). In contrast, the protein structures used to train AlphaFold had to be painstakingly deduced via crystallography.
So if maths and physics are such fertile territory for AI-driven science discovery, why, beyond a few success stories, aren’t we seeing a scientific revolution?
The hold-up is not the computational cost or even the difficulty of the problems. Rather, it is the way we organise discovery itself.
Two cultures
AI-assisted discovery of scientific principles requires a combination of humans and machines. Machines, coded by AI experts, are good at spotting patterns; humans, usually scientists, are good at telling which patterns are important.
AI experts work at for-profit companies and are mission-driven. Scientists work at universities and other non-profit research institutions and are self-driven.
The two groups rarely sit at the same table. For AI-assisted discovery to flourish, they will need to.
To that end, the London Institute has launched a company called Irix, which aims to use machines to speed up fundamental discovery. Owned partly by investors and partly by the institute, Irix should be a symbiosis between human ingenuity and intelligent automation, operating at the frontier of our understanding of the universe.
What price golden eggs?
At a recent session of the Science and Technology Committee, the UK science minister Patrick Vallance said his top priority for British science was to “protect and grow basic curiosity-driven science”. It’s “the goose that lays the golden egg”, he said, creating “all the economic and societal benefits”.
Even so, the difficulty of putting a value on fundamental discovery makes governments wary of funding it.
Estimating the cost of discovery is a bit easier. A typical research paper might cost $250,000, factoring in author salaries, workloads and the cost of publishing. By that measure, the 210,000 papers posted on the ArXiv repository for physics, mathematics and computer science in 2023 would have cost $52 billion.
That same year, the private sector spent $570bn on advanced technology R&D. The irony, though, is that the ultimate bottleneck to technological innovation is the repertoire of scientific principles available to harness.
Technological cycles are speeding up, and the lag between principle and product is shrinking. Companies across space, mobility, cybersecurity, bioengineering, alternative computing and generative and applied AI have a vested interest in the acceleration of science.
As a result, the market may end up doing what governments have struggled to do: figure out how to value fundamental discovery. As scientists and AI experts sit down together for the long haul, we can expect radically new technologies—and a banquet of Nobel prizes.
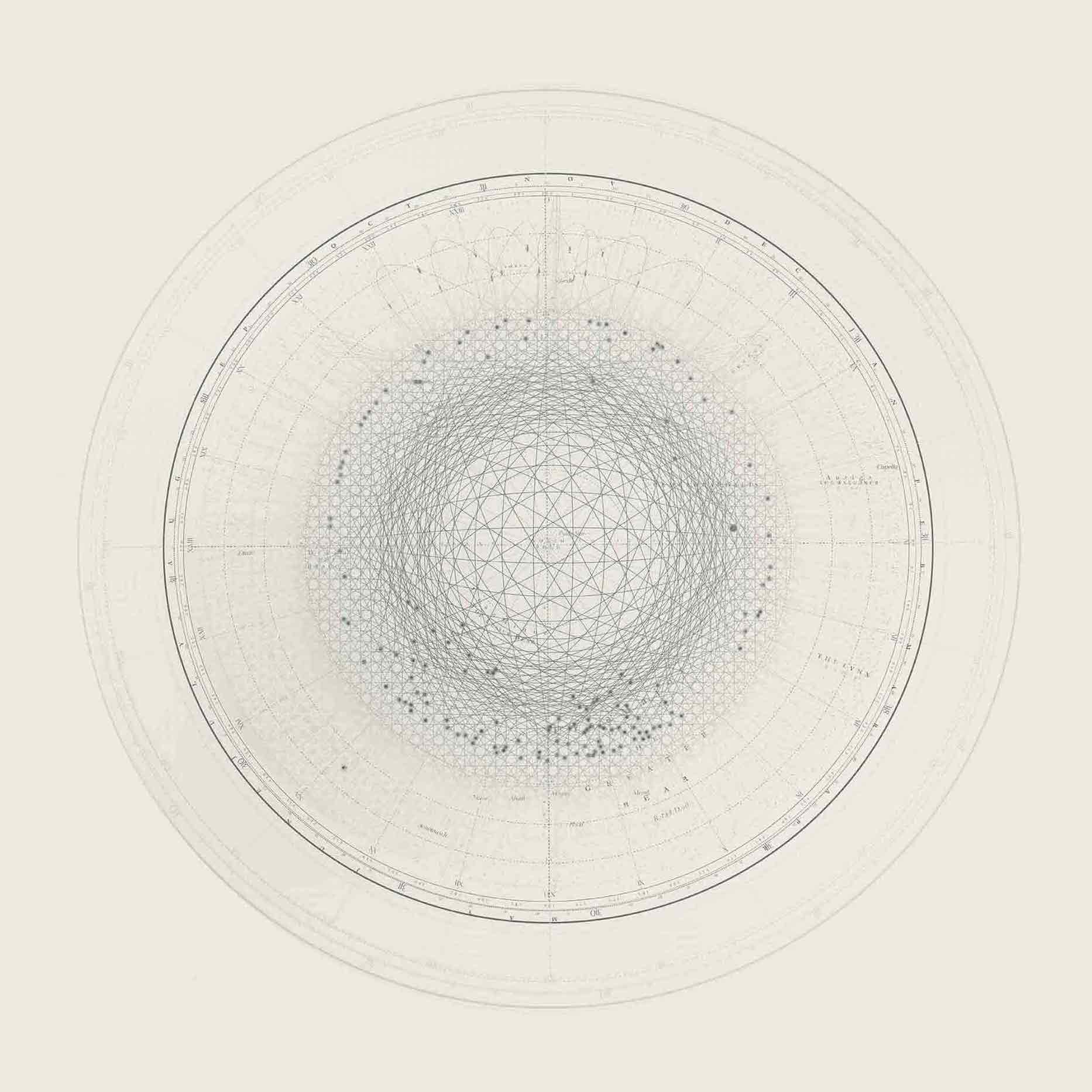
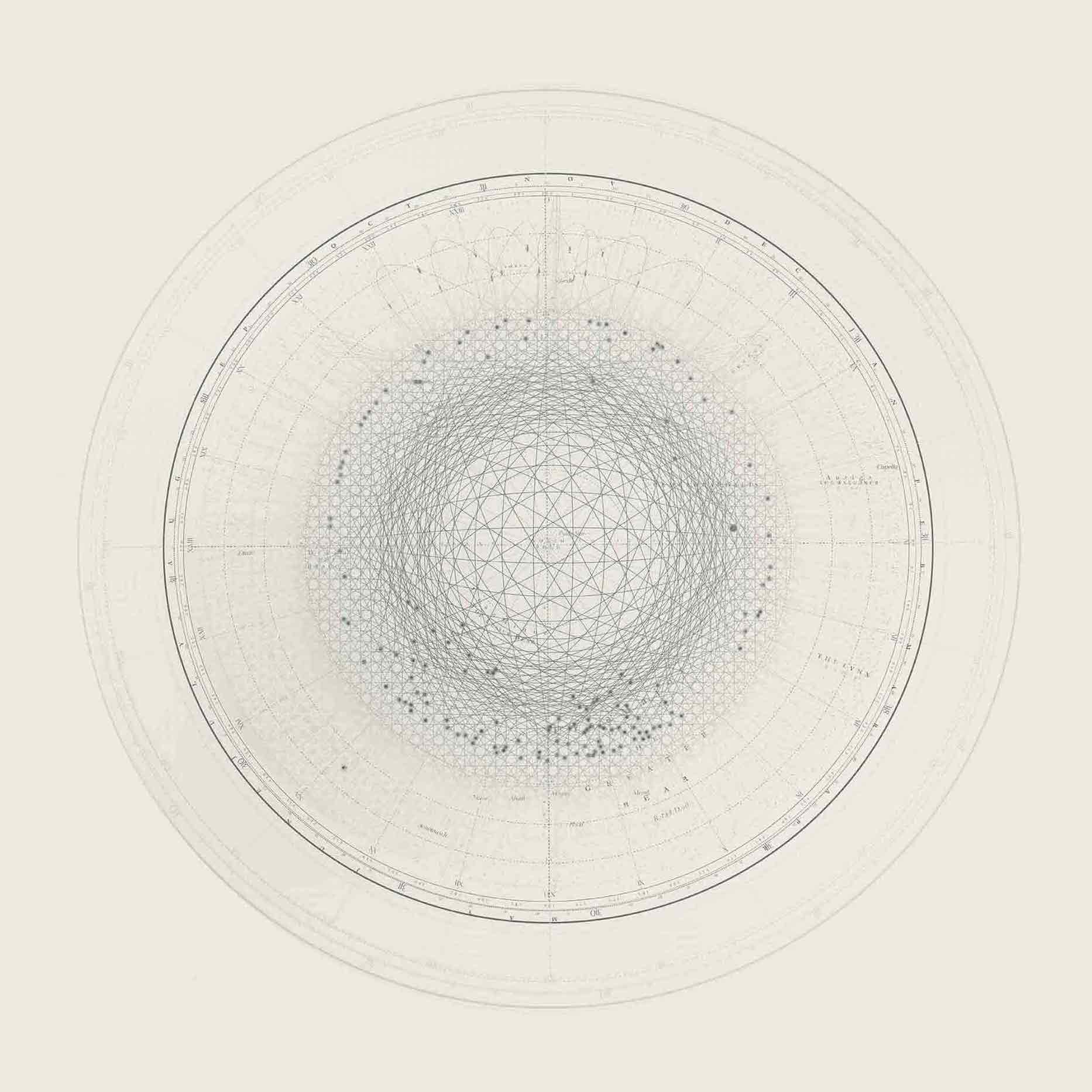
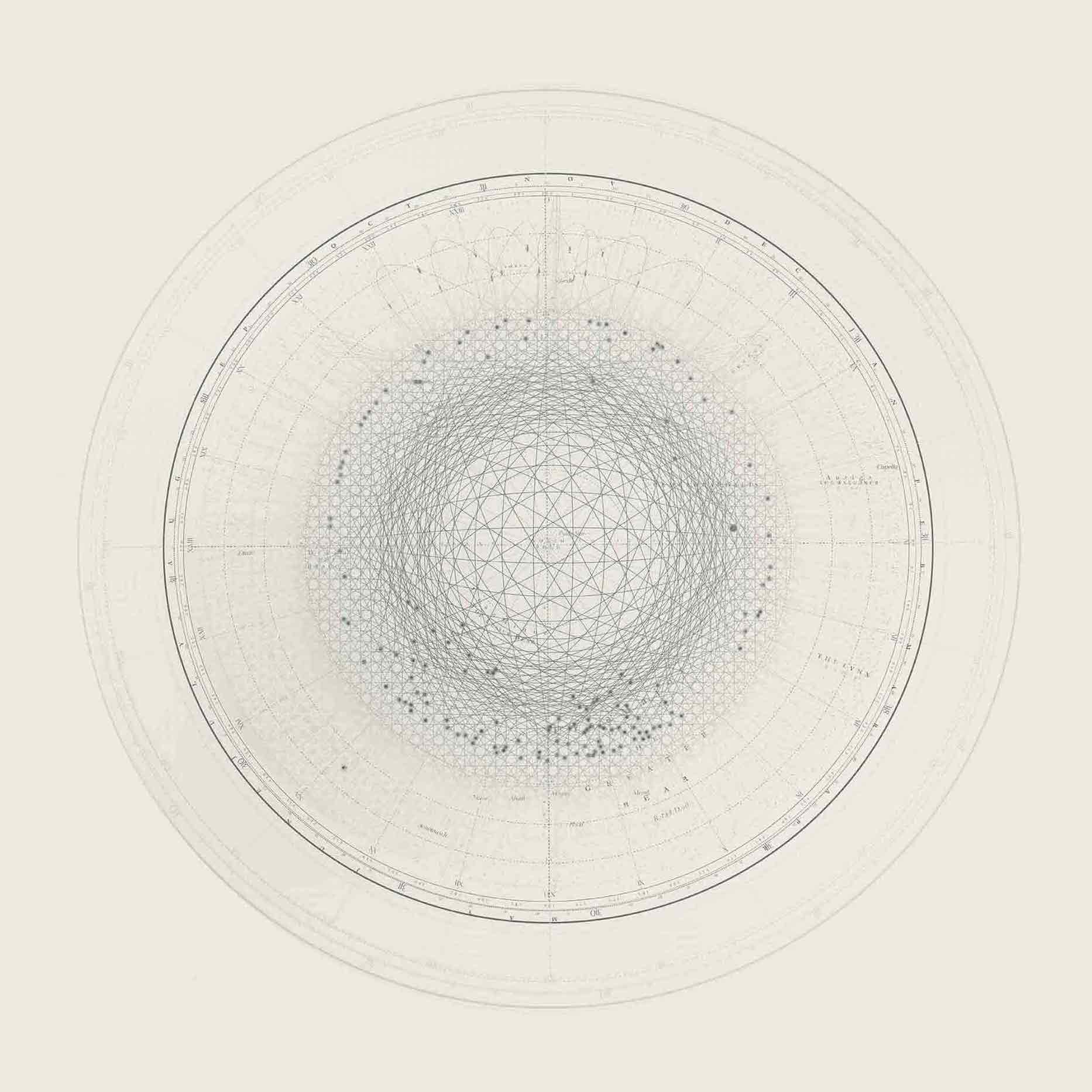
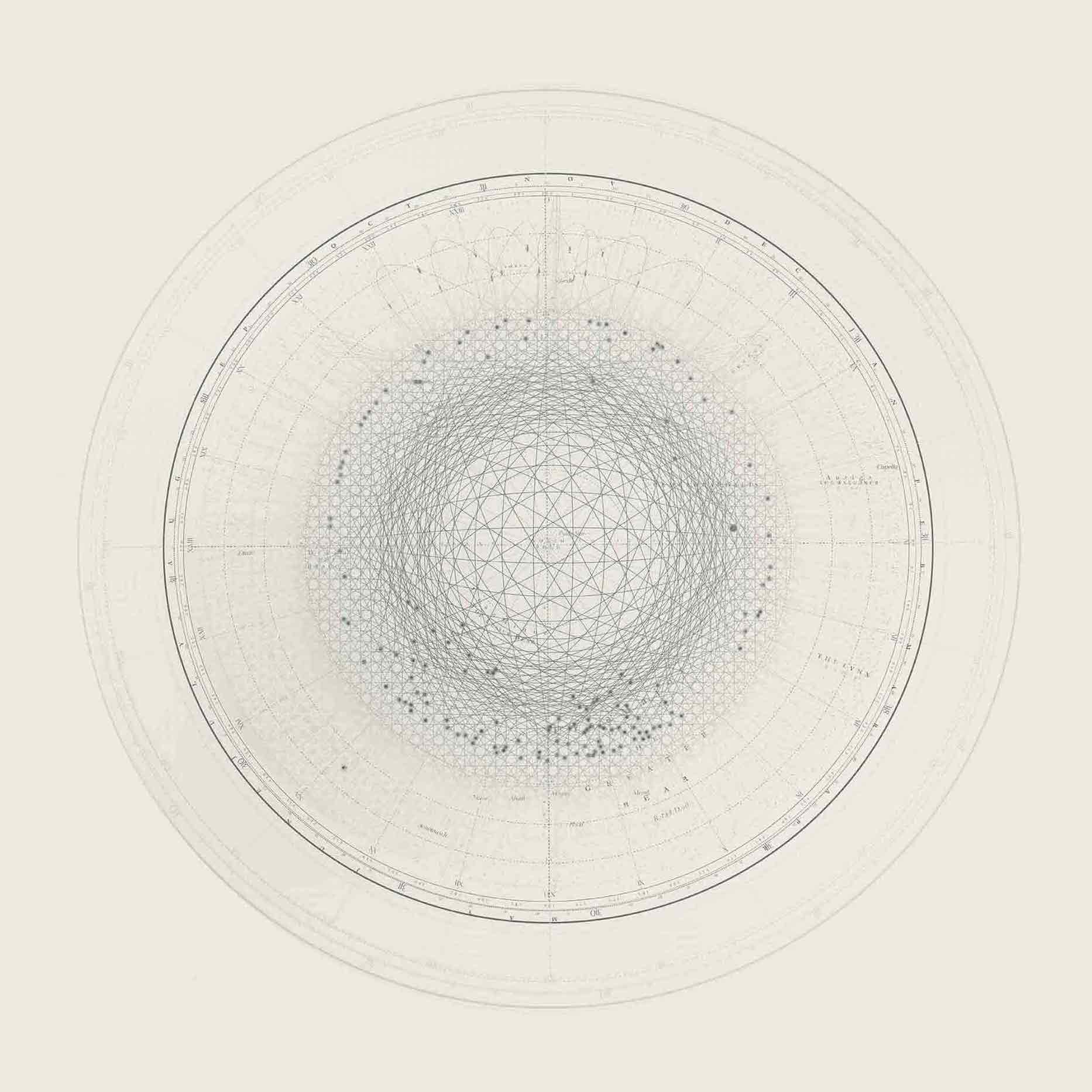
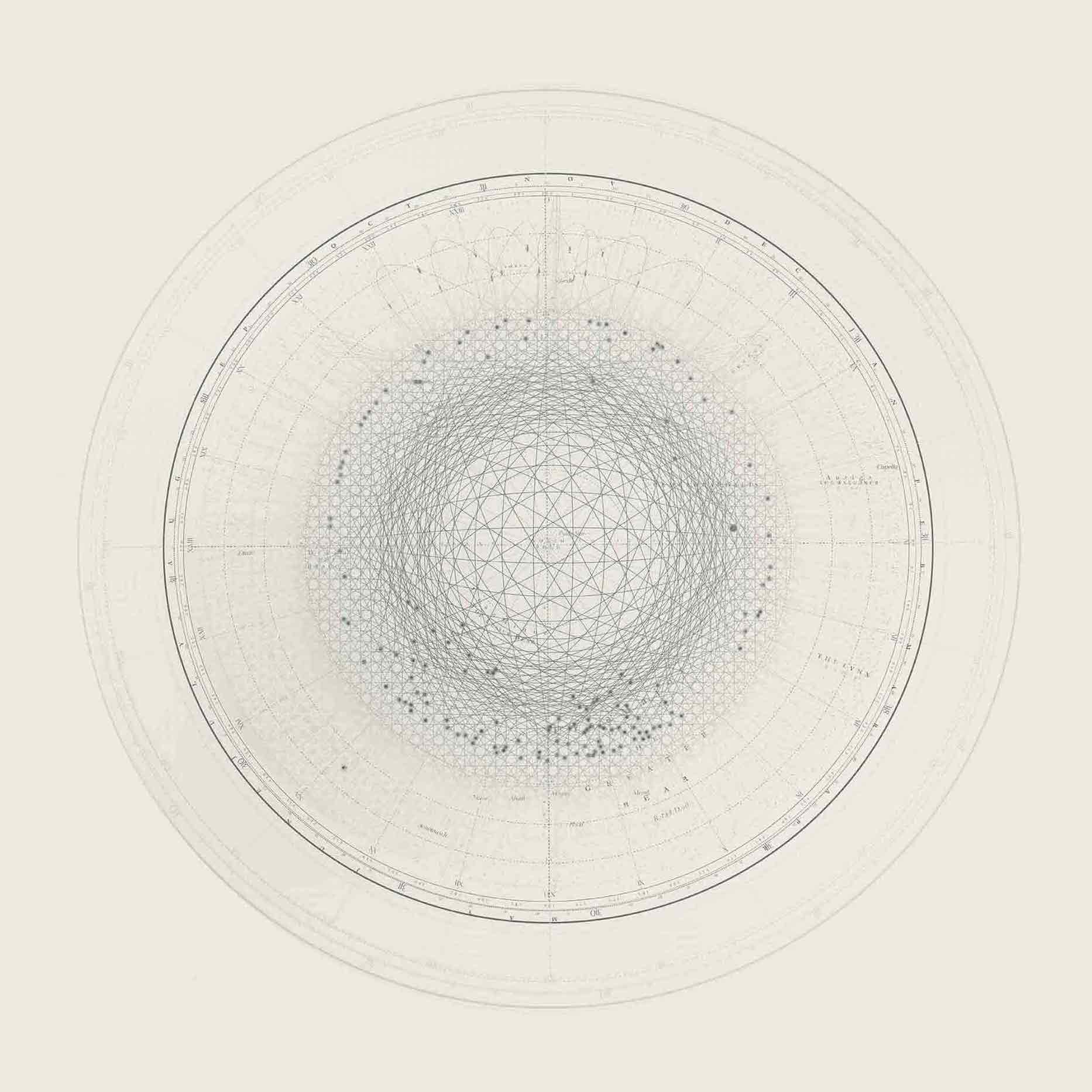
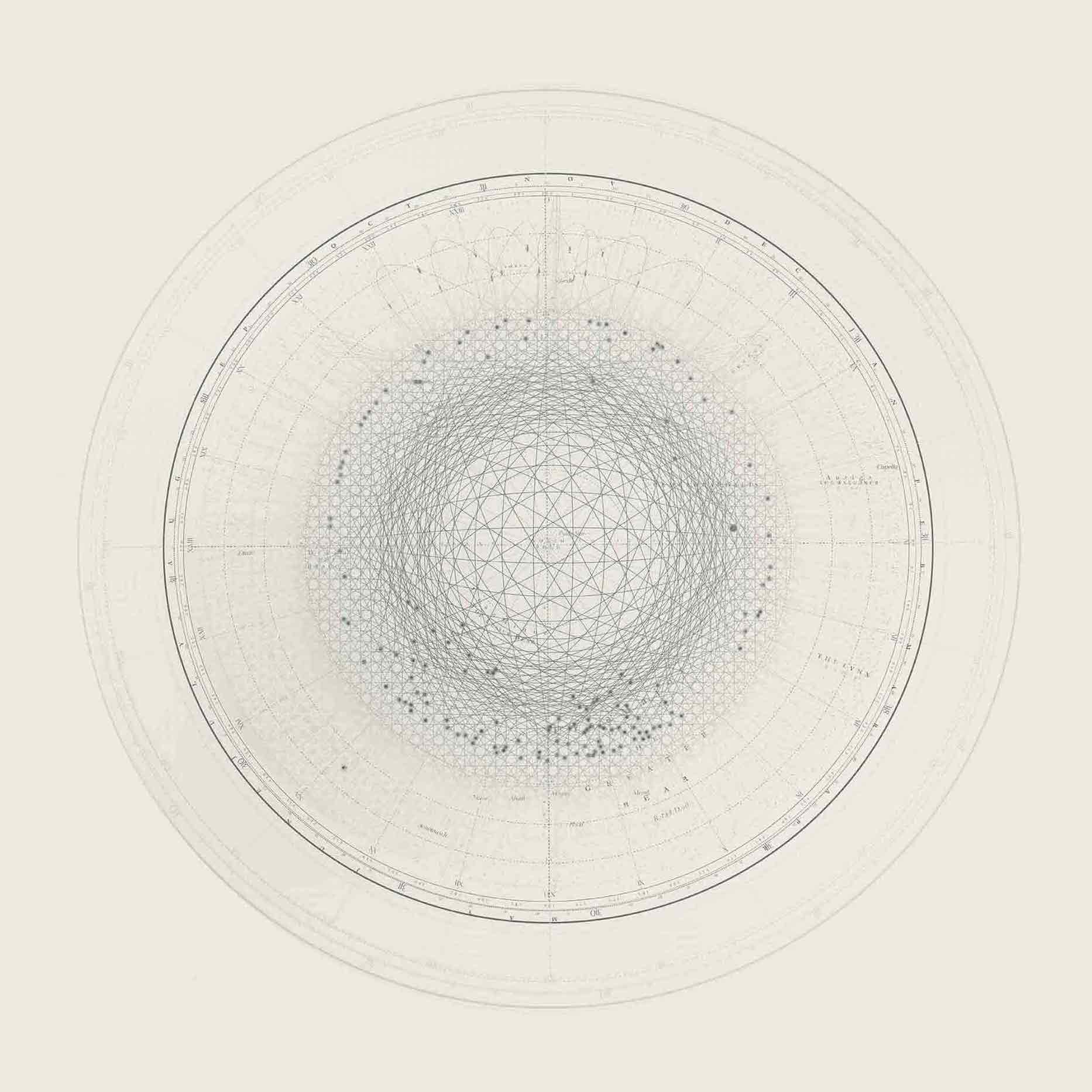
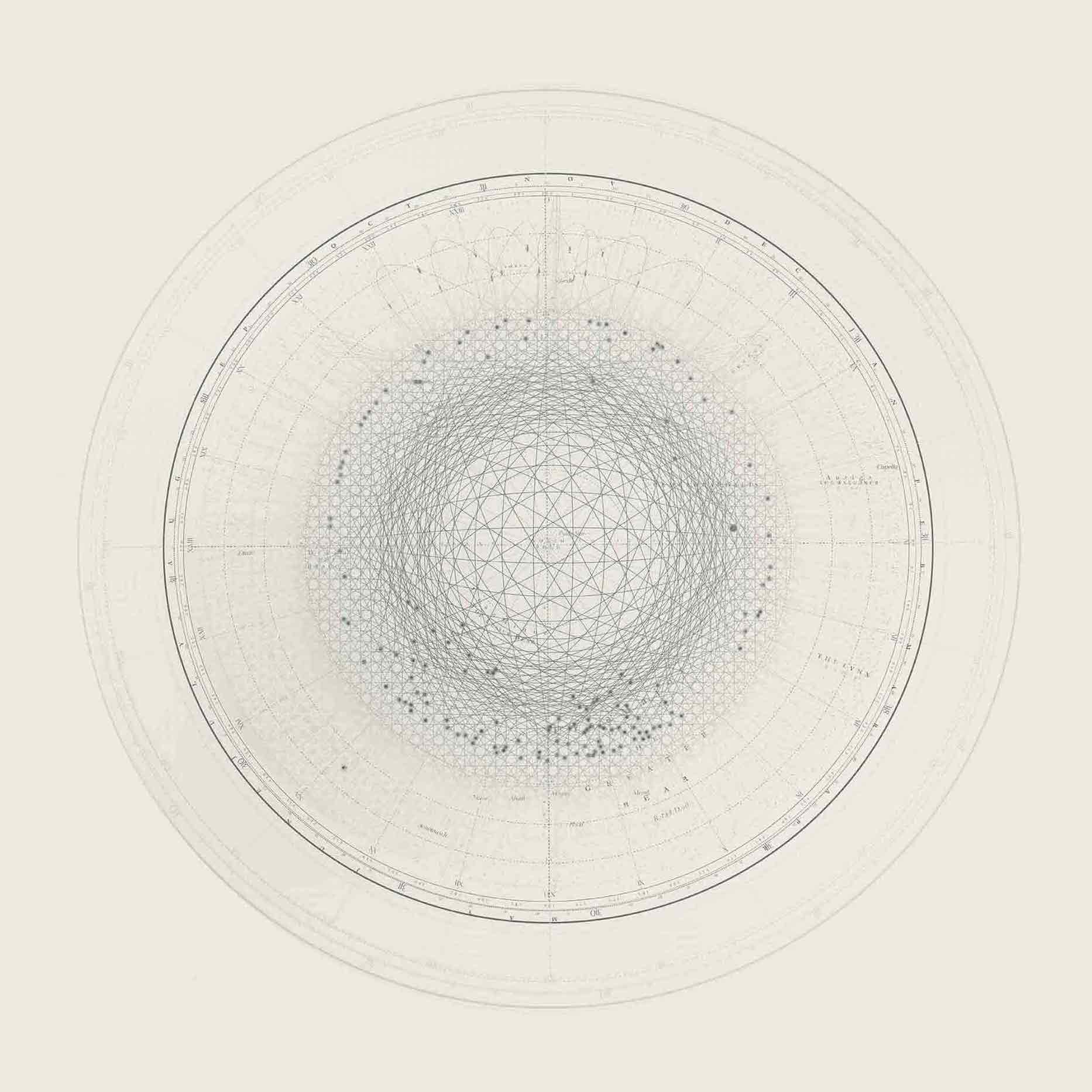
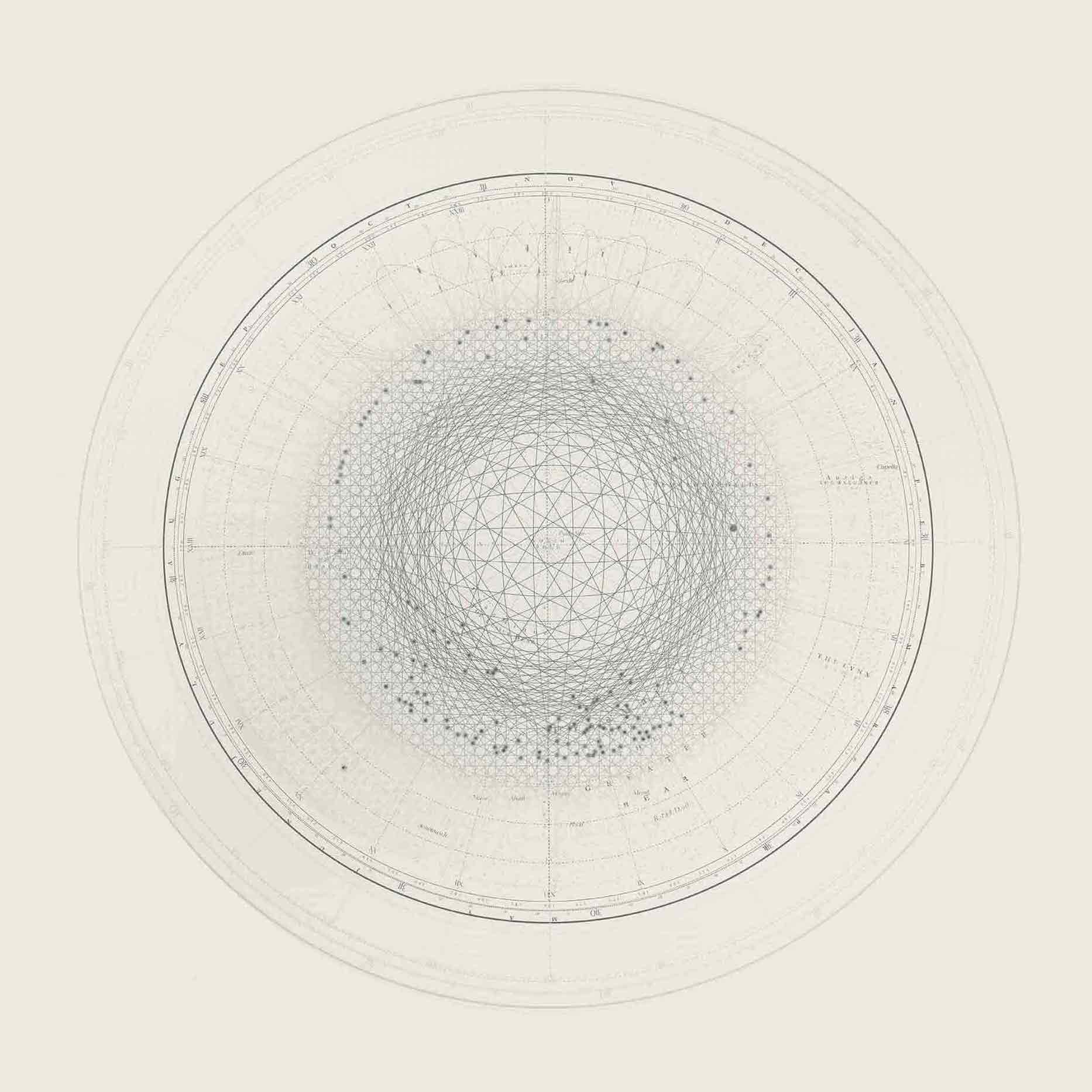
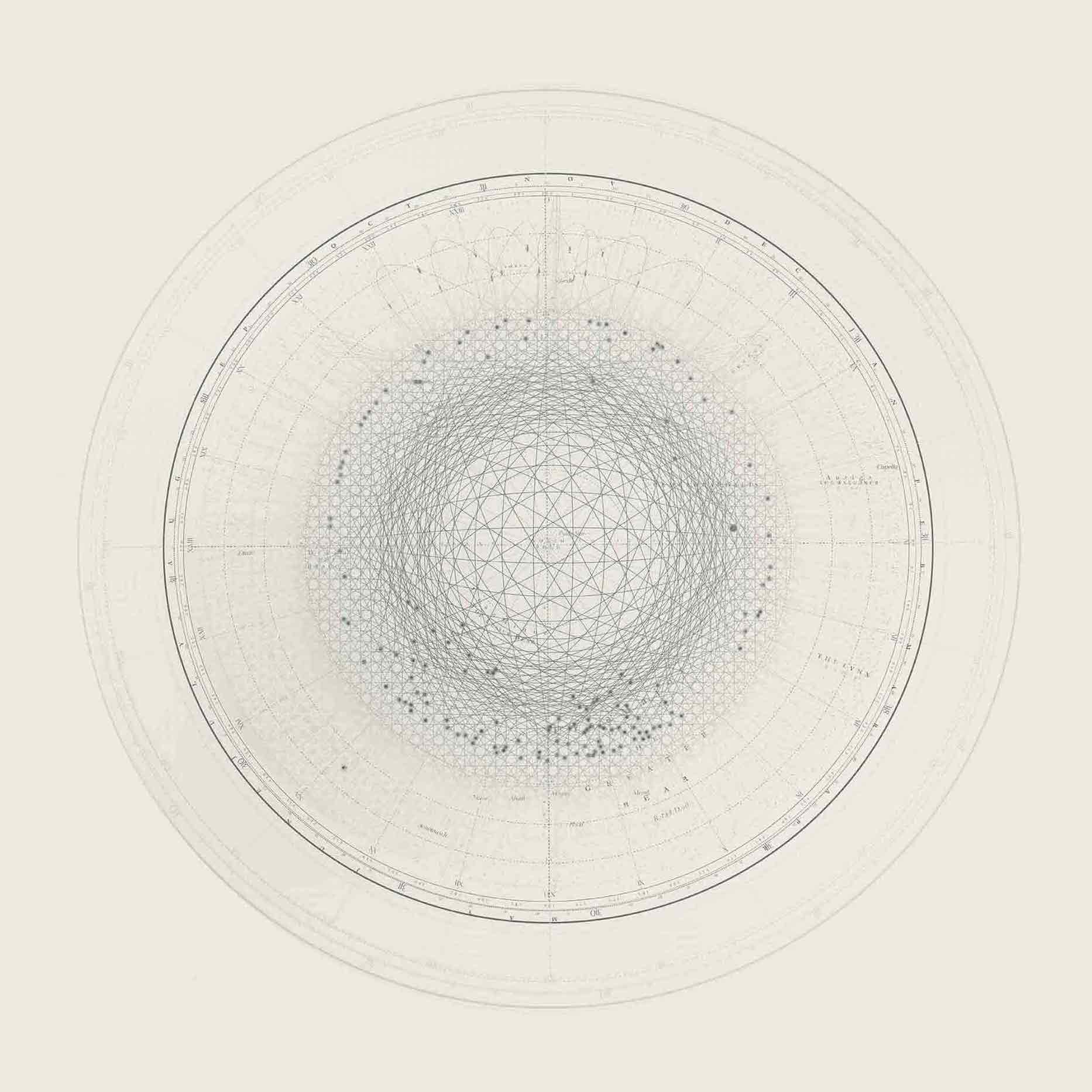
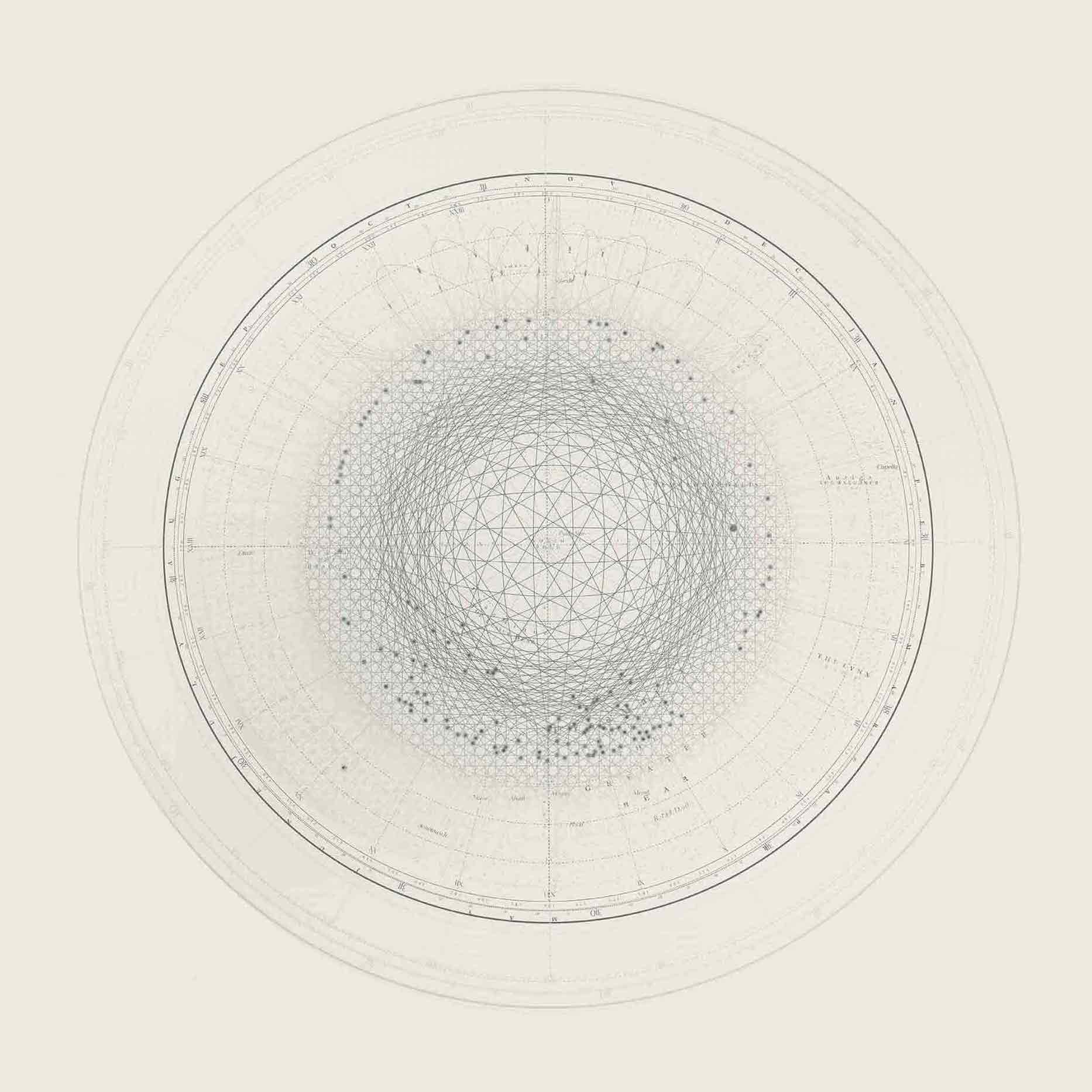
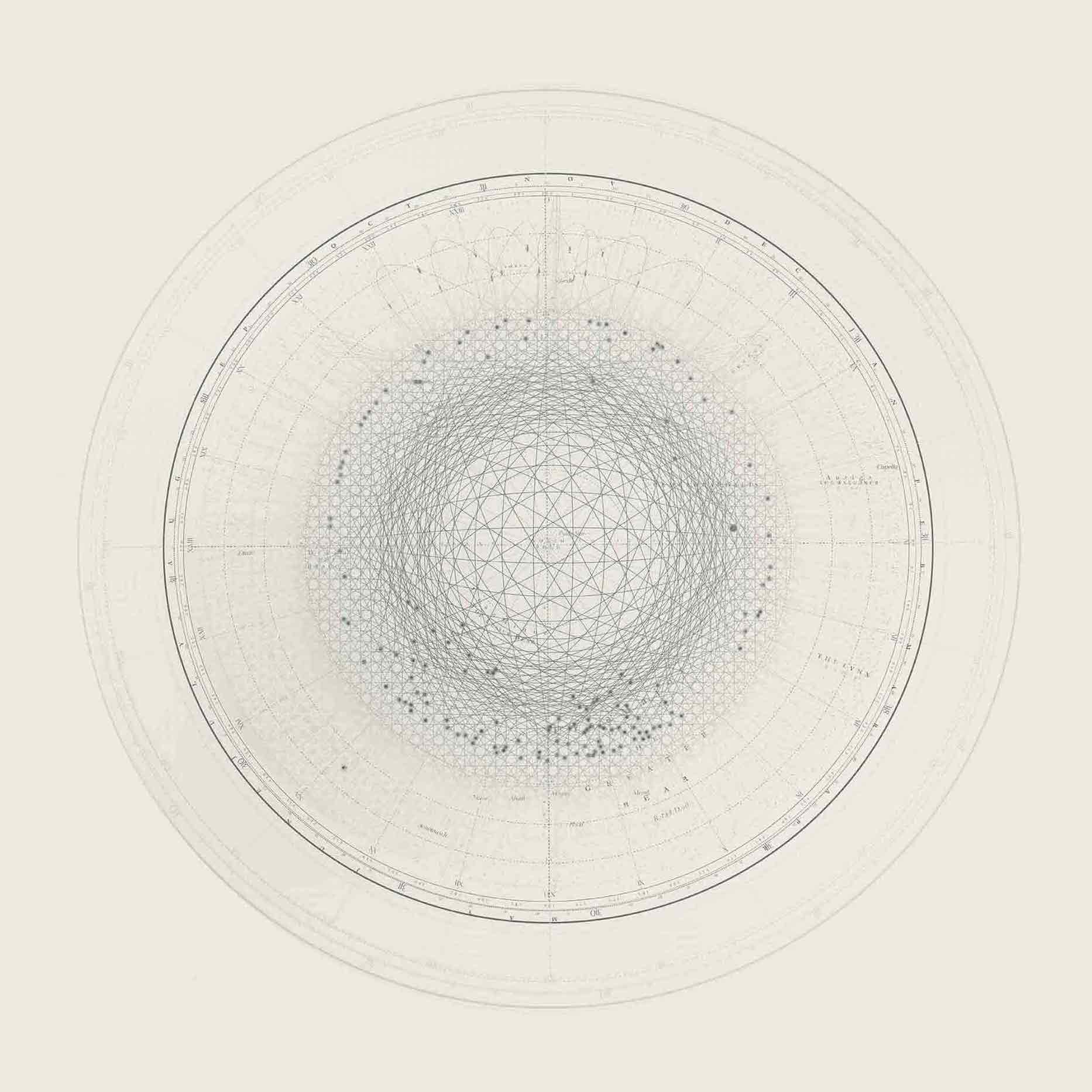
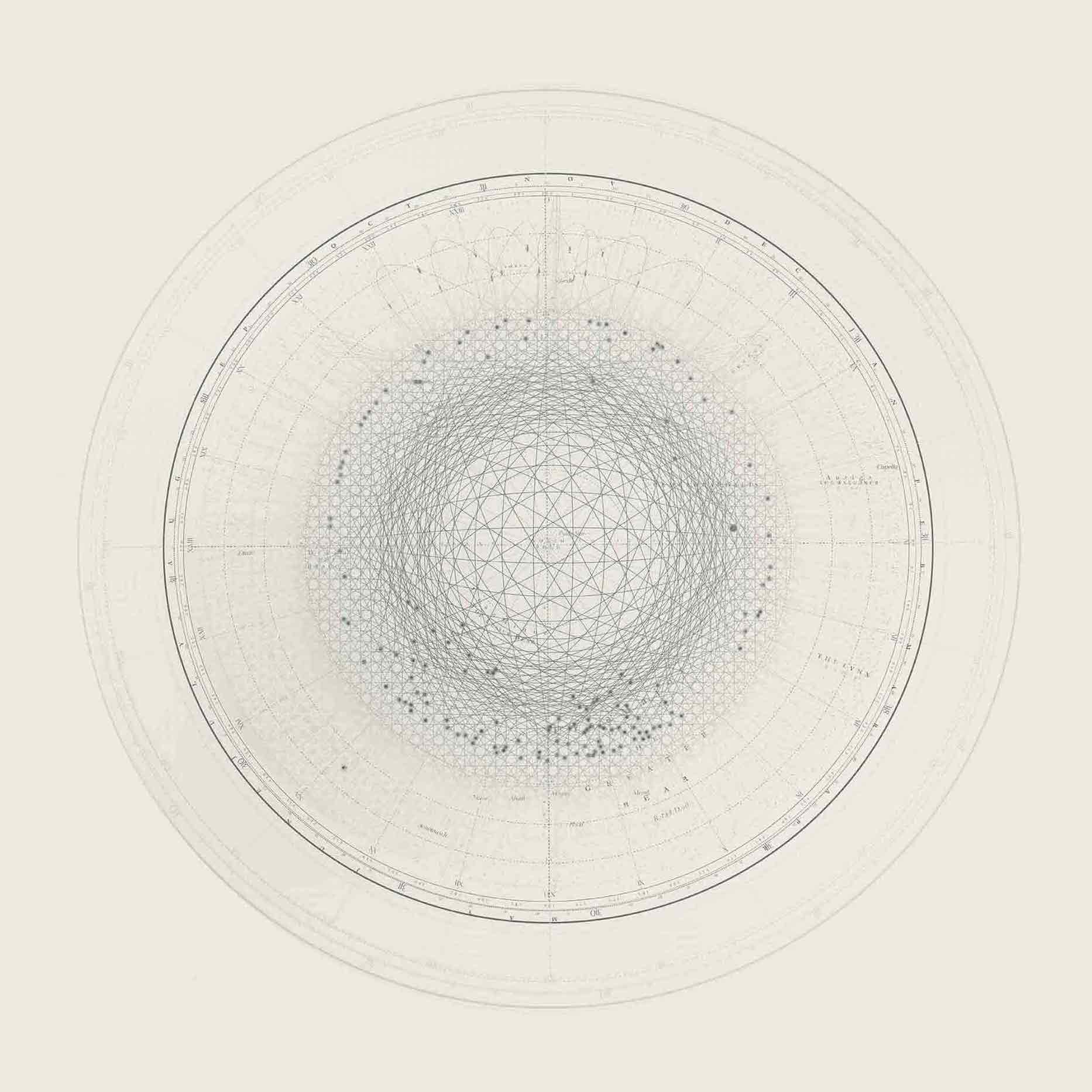
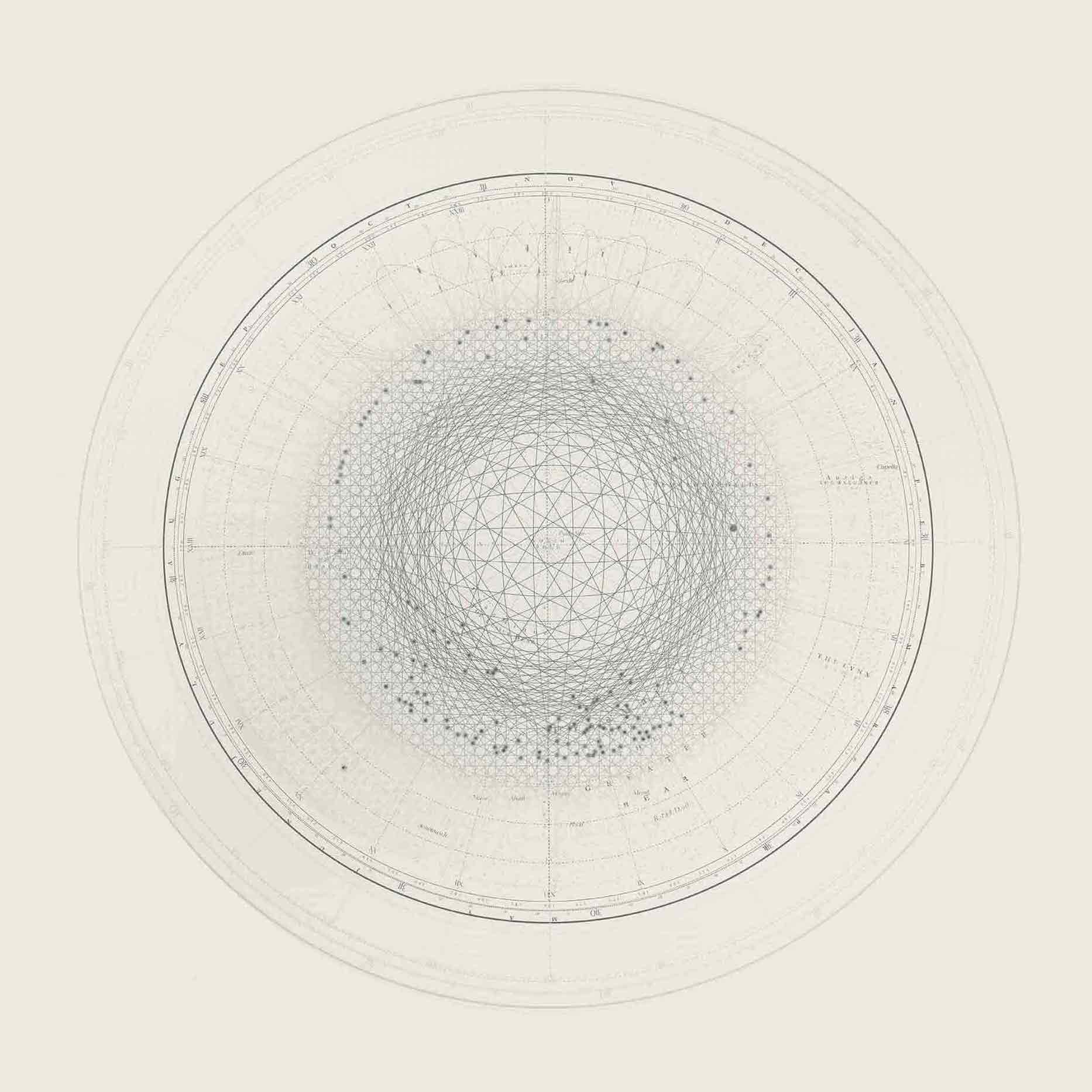
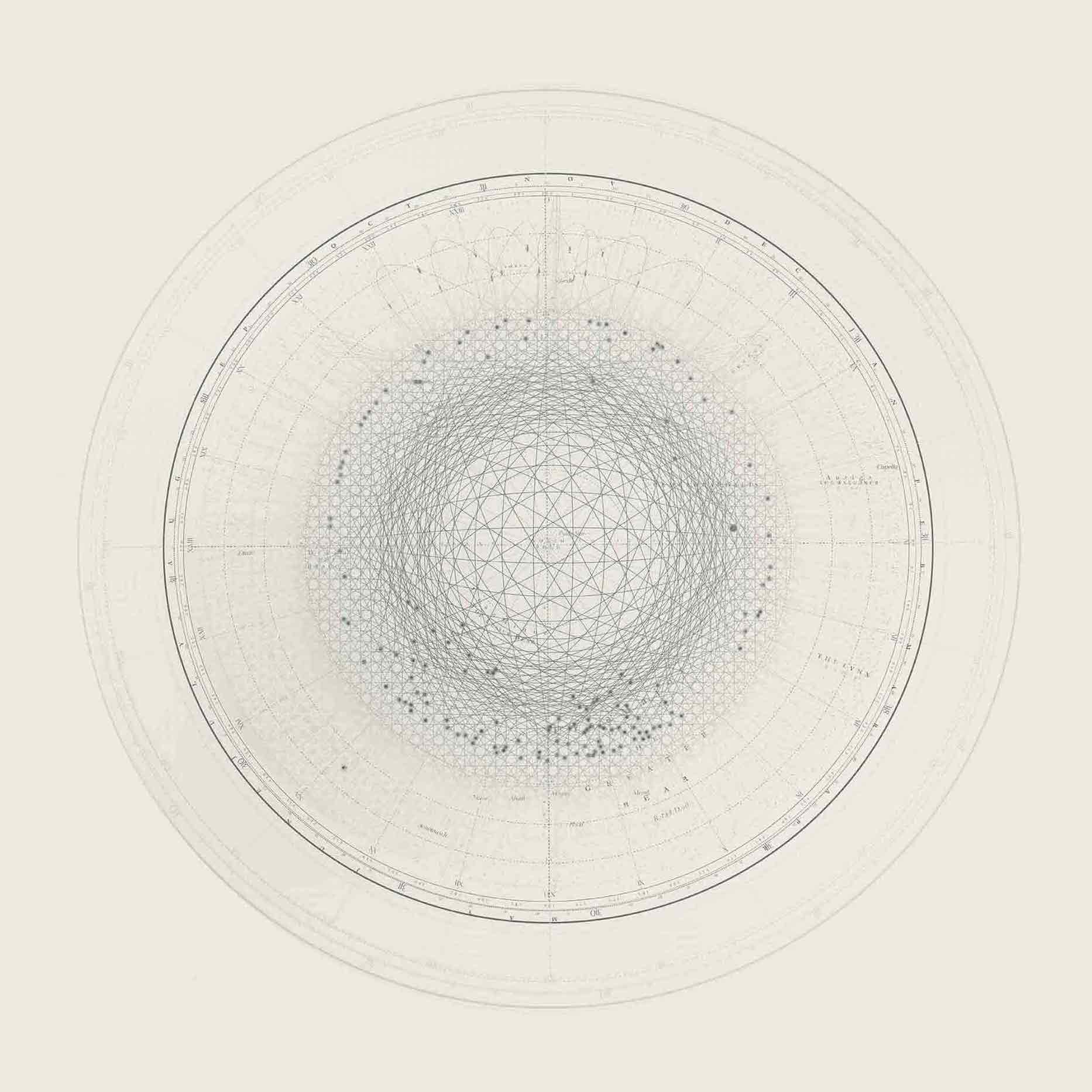
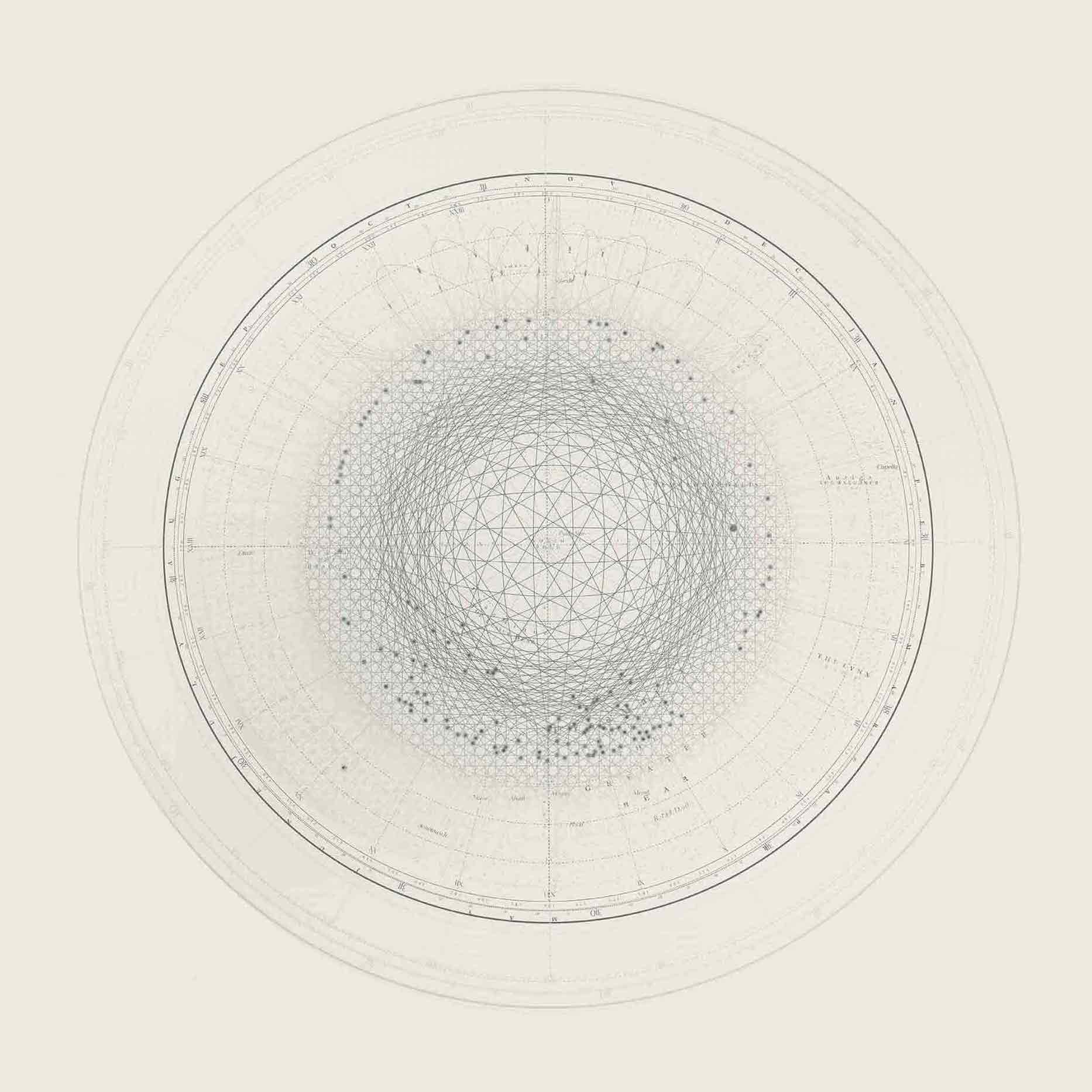
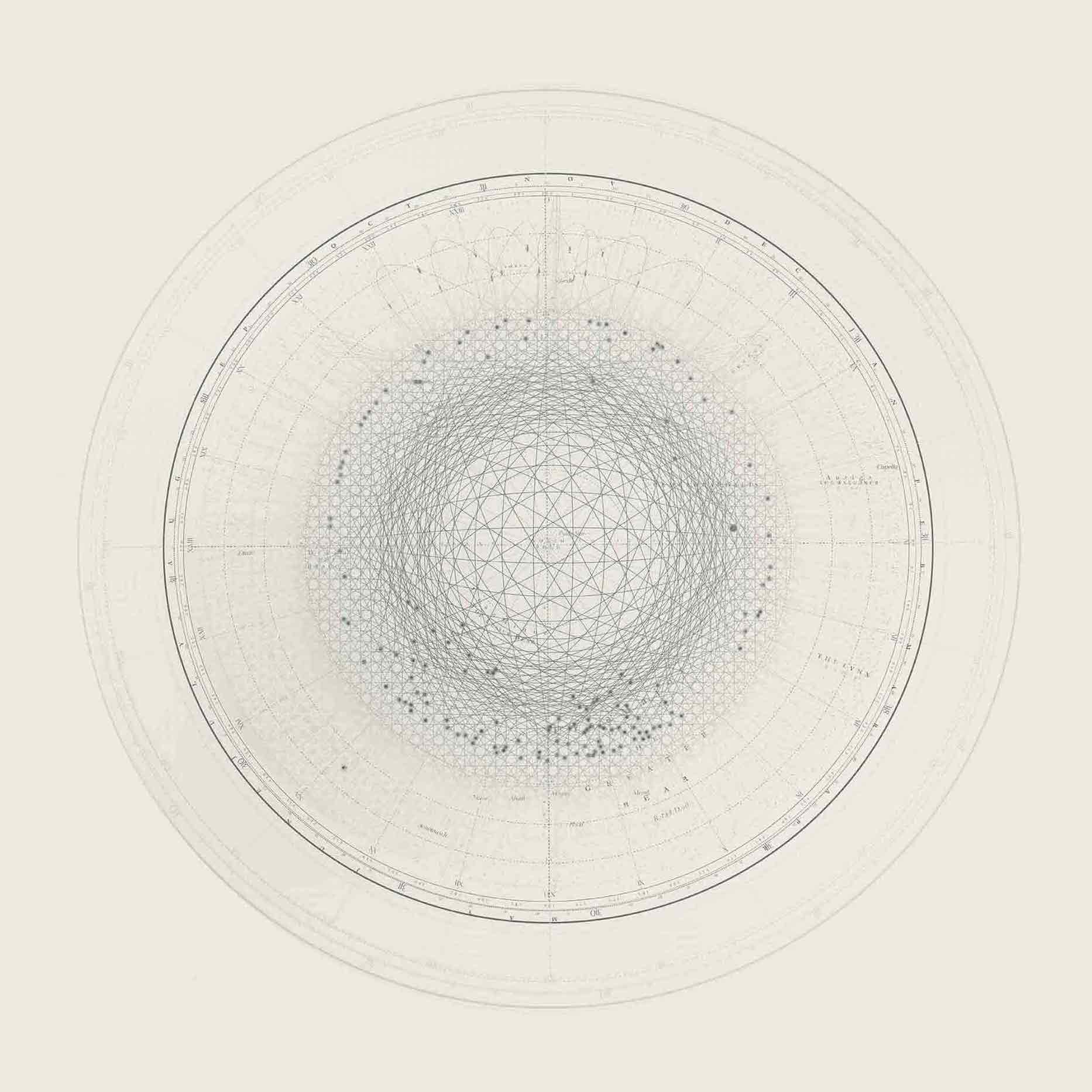